"By fully implementing this technology, Radius can save millions in dedicated cabinet surveying costs. We will use existing imagery where we already have a process for updating pictures during the ordinary inspections. The added benefit for our customers is that we can now provide answers in seconds instead of days."
case study
Machine Learning Techniques Enrich Asset Data
Radius operates the Denmark electric grid, which supplies energy to over one million customers in Copenhagen, North Zealand, and Central Zealand. Radius recently merged with a neighboring utility and therefore needed to combine information about the electric systems. This process identified gaps in the data that would affect operations.
Electric & Gas
Challenge—Missing information for low-voltage secondary fuse cabinets caused delays in processing new customer connections.
Solution—Artificial intelligence tools in ArcGIS processed existing photographs efficiently and accurately to extract the missing information.
Results—The process enriches asset information in minutes—staff no longer have to wait for the next inspection cycle to gather additional data in the field.
Challenge
The utility operates approximately 300,000 low-voltage secondary fuse cabinets. These cabinets establish connection points for new and existing customers. After the merger, staff discovered that some of the data about fuse cabinets was incomplete. For example, some cabinets were missing information about the fuse type, capacity, and unused slots inside.
Because surveying so many cabinets in the field would require a substantial investment in time and labor, the utility sought a better way to glean this information.
Solution
Radius held an employee innovation week to encourage creativity and the application of new technology. One team used ArcGIS to test using artificial intelligence to enrich the cabinet data efficiently with machine learning techniques. The team used existing photographs of various cabinets to prepare the machine learning model. Staff members applied the open-source Visual Object Tagging Tool (VoTT) to annotate sample photographs. This process demonstrates how to distinguish the features by creating examples that enable the solution to detect the bonding box types and class labels with predictive accuracy.
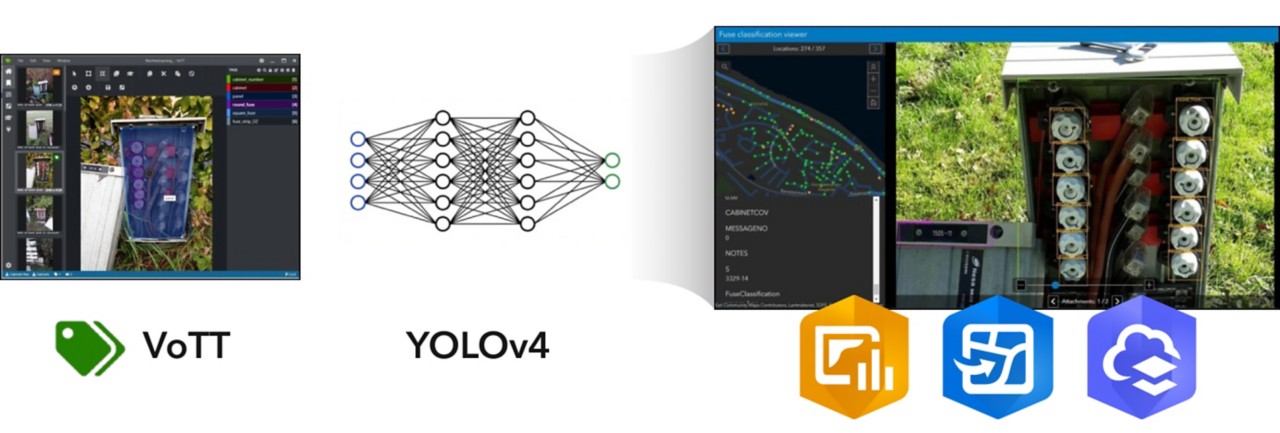
Artificial intelligence, using machine learning techniques and tested by ArcGIS, detects the features it was trained to recognize. (square fuse.png)
Building on this data, the team leveraged the You Only Look Once (YOLO) object detection method. YOLO is an open-source technique for image recognition, written in a dark net framework. The YOLO model gives good prediction accuracy. Good performance was essential to support the intended applications like real-time identification of features with mobile devices.
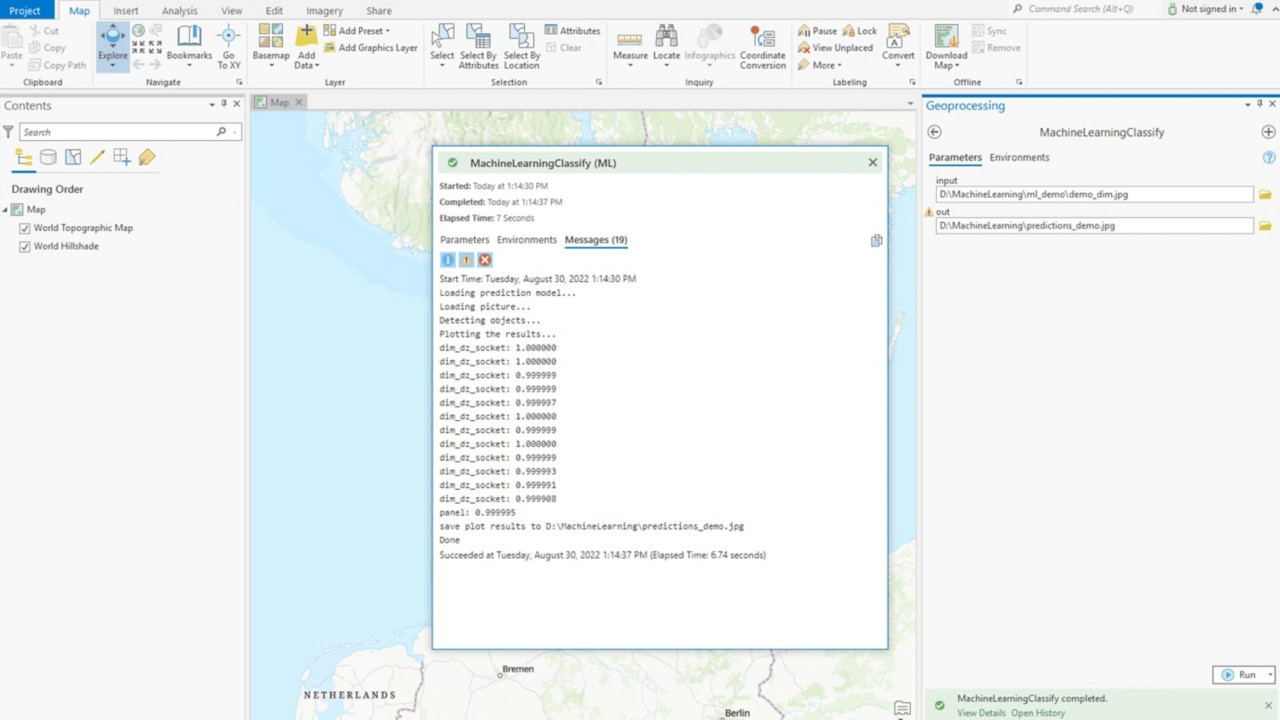
With an adequately trained machine learning model, the team employed ArcGIS Pro to programmatically evaluate additional cabinet photographs. Using Python and deep learning frameworks for ArcGIS, the model processed input pictures; runs object detection; and outputs visual results, recording them in the database. These steps derived new cabinet and fuse data automatically using machine learning.
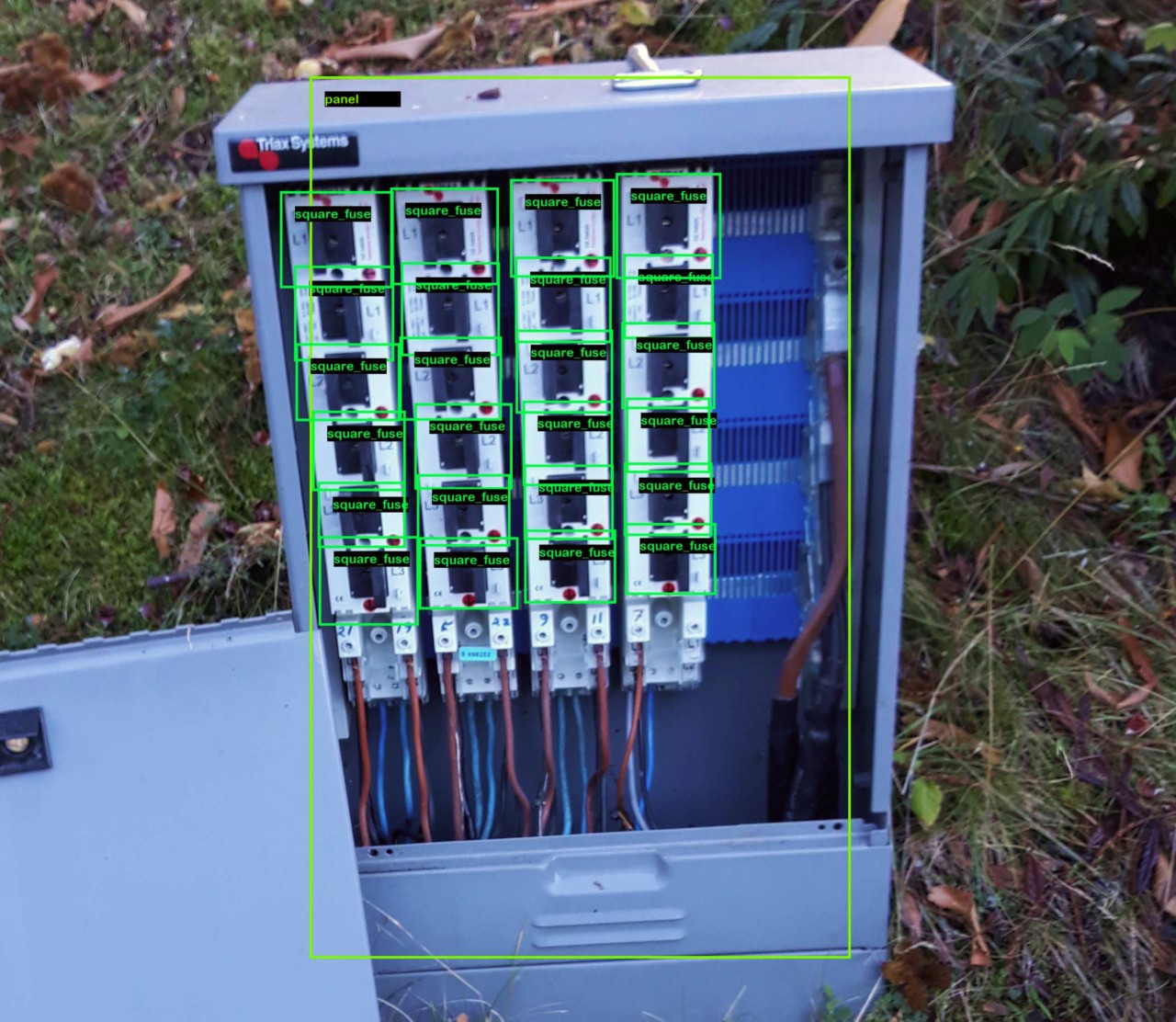
Results
The usual practice was to send a technician to inspect and verify all cabinet data before designing a new connection—for example, verifying that a fuse position was open and available for a new customer. Radius expects to avoid these trips to the field by utilizing reliable data derived from existing equipment photographs.
The machine learning analysis accurately identified batches of photos that are useful for verifying cabinet type, designation, number of positions, and fuse type. The process enriches asset information in minutes, so staff no longer have to wait for the next three-year inspection cycle to gather additional data. The resultant accuracy exceeded 99 percent, surpassing the results from typical manual methods.
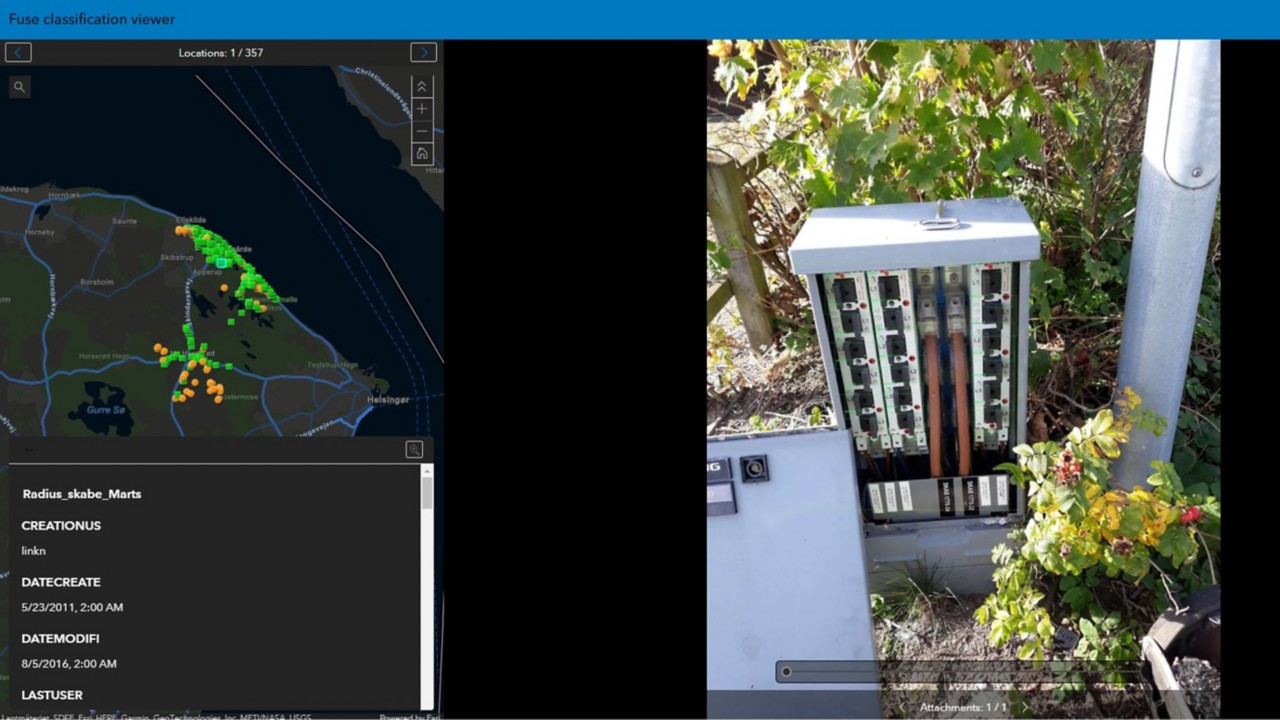
In the future, Radius plans to expand this tool to determine used and unused positions within the fuse cabinets. Having this information will improve speed and customer service while reducing costs.