Solar power presents an immense opportunity for generating sustainable and green energy. Realizing its full potential requires identifying suitable locations for solar panel installations.
Pivot Energy, a national renewable energy provider headquartered in Colorado, needed assistance locating parking lots across various areas of interest that are appropriate for potential solar panel implementation. Using advanced GIS technology, a team from Esri partner Platte River Analytics helped Pivot Energy do this accurately and efficiently.
By leveraging a deep learning model from Esri, the team at Platte River Analytics extracted parking lot surfaces from high-resolution imagery. The team then used geoprocessing tools in ArcGIS Pro to conduct more precise measurements and calculations of potential sites.
The results of this analysis provided Pivot Energy with invaluable information, empowering staff to make data-driven decisions and plan out solar power adoption efforts more effectively.
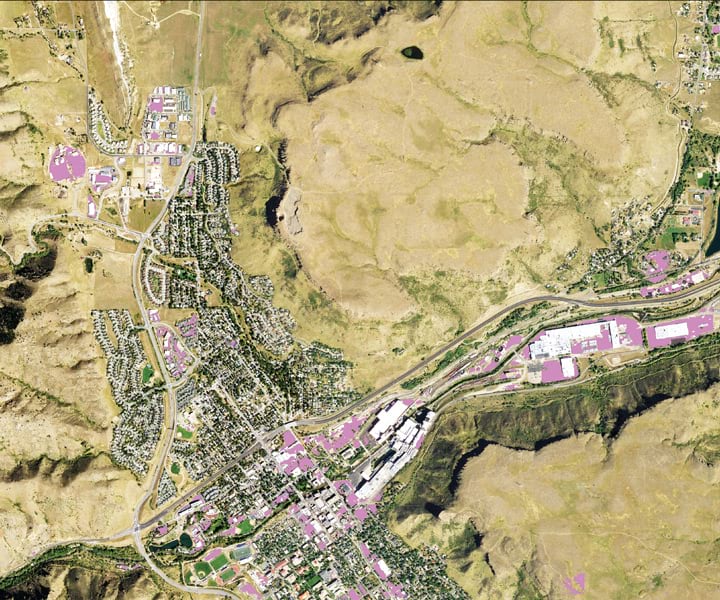
The Benefits of Parking-Lot-Based Solar Development
Parking lots offer significant yet underutilized space for solar power development. They possess key characteristics that make them ideal for generating solar energy, such as large surface areas, unobstructed exposure to sunlight, and proximity to electrical infrastructure connections. In addition, paved parking lots typically have very low slopes, are designed to drain, don’t compete with other land uses, and aren’t in full use all the time.
Solar-powered parking lots can provide numerous environmental and economic advantages to owners and communities as well. They can be quickly equipped with electric vehicle (EV) charging stations. This not only enables EVs to be powered directly by solar-generated energy, promoting clean transportation, but it also reduces electricity costs for owners and operators and opens avenues for potential revenue generation through energy sales. In addition, offering EV charging to customers attracts car owners who will spend money in the area while charging their vehicles.
All these are reasons why Pivot Energy saw the need to scour parking lots across the East Coast and, eventually, the nation to see which ones are ideal for solar energy development.
A Quick, Automated Way to Detect Suitable Parking Lots
To automate the detection of parking lots in Pivot Energy’s areas of interest, the team at Platte River Analytics relied on Esri’s Parking Lots Classification – USA deep learning model, available in ArcGIS Living Atlas of the World.
Developed by the Esri analytics team, this prebuilt model is trained to identify parking lots within sourced areal imagery. Like the more than 65 other deep learning models that the Esri analytics team has developed to detect objects ranging from Arctic seals to power lines, the Parking Lots Classification – USA model automatically extracts the assets from imagery without users having to invest time or money in training data or personnel.
For this project, the team at Platte River Analytics needed to use high-quality, submeter data that allowed the model to identify and analyze land features as detailed as parking lots. The team acquired one-meter resolution National Agriculture Imagery Program (NAIP) imagery from the United States Geological Survey’s EarthExplorer web app. The imagery in this app has been acquired by the US Department of Agriculture during agricultural growing seasons from 2003 to the present.
After downloading the NAIP imagery, the Platte River Analytics team seamlessly integrated the deep learning model into its ArcGIS Pro workflow. The team processed the imagery with the model, which automatically identified parking lots across dozens of Pivot Energy’s areas of interest.
The model was easy to use. The initial area of interest that the team looked into was the size of a large US city, and it took less than 12 hours to both download the imagery and process it in ArcGIS Pro.
To further analyze the identified parking lots, the team at Platte River Analytics used the Raster to Polygon geoprocessing tool in ArcGIS Pro to convert the raster outputs into polygons. This enabled the team to get more precise measurements and calculate the size of each lot, providing valuable information to Pivot Energy so staff could begin conducting feasibility assessments and get started with project planning.
From there, the GIS team at Pivot Energy was able to study regulatory factors—such as floodplains, tree cover, wetlands, and wildlife migration routes—around parking lots that were initially deemed acceptable for solar development.
Saving Dozens of Hours of Manual Work per Week
Taking a machine learning-based approach to finding suitable parking lots for solar panel installation enabled staff at Pivot Energy to make informed decisions quickly regarding which areas and specific parking lots could work for this endeavor. By using advanced GIS to assess parking lot locations and sizes, the developer can optimize project planning, ensure maximum energy generation capacity, and speed up the installation of solar infrastructure.
According to Rachel Mead, GIS manager at Pivot Energy, the process that the team at Platte River Analytics used to extract parking lots from imagery saved her own team more than 20 hours per week of manually searching aerial imagery for—and digitizing parking lots throughout—the company’s areas of interest, which stretch across the United States.
“It has been a huge time-saver having access to the deep learning models provided by Esri,” she said. “By automating this…we can save dozens of hours per week and realign that time to other projects.”