For most cities, keeping paved roadways in good condition is the single largest maintenance function they perform. This includes sweeping streets; patching pavement; applying life-extending overlays to streets and highways; and road striping to ensure that drivers, pedestrians, and others know the rules of the road.
Having a complete inventory of public roadway surfaces and pavement conditions is the first big step any city needs to take when looking to understand what kinds of road-based maintenance and improvements to undertake. Knowing the number of paved lane miles and the condition of the paved roadway network is critical for determining future maintenance costs and prioritizing funding.
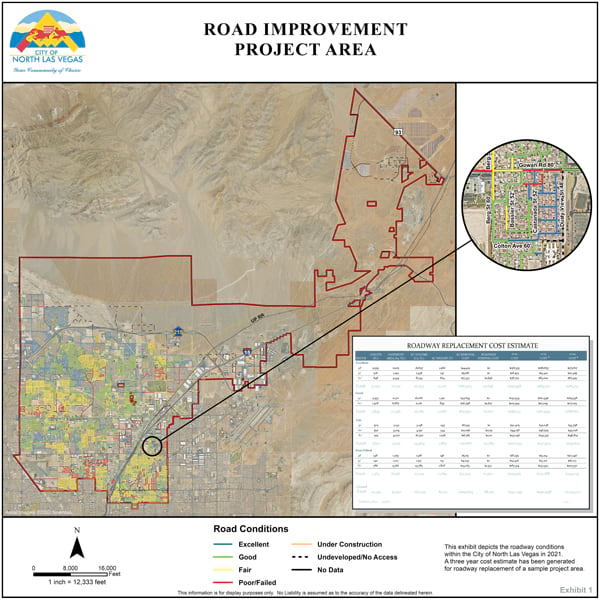
Over the last several years, the City of North Las Vegas, Nevada, has used ArcGIS technology to map its entire 102-square-mile area and create the various infrastructure layers needed to develop a geodatabase of off-site improvement projects (i.e., construction projects to repair and enhance infrastructure used for public travel). The aim was to build a roadway network schema that the city could employ to determine where pavement replacement needed to occur and how much it would cost. Now, this data is being used for longer-term planning on other roadway projects throughout North Las Vegas.
Putting Hodgepodge Data into a Single GIS Layer
As in many cities in the United States, North Las Vegas consists of private roads and public streets and highways that are maintained by different authorities. Moreover, the city is continually growing. From 2015 to 2020, it added 20.6 centerline miles to its road network, increasing its roadway profile by 3 percent over that five-year period. Thus, conducting a roadway inventory and converting it into useful data wasn’t a straightforward process and required frequent updates.
The inventory that the City of North Las Vegas carried out includes 640 centerline miles of public paved streets and 176 centerline miles of private paved roads. Since the city only maintains public roads, mapping and evaluating the public roadway network and associated road conditions in a single GIS layer was a major component of assembling the geodatabase.
To map the roadways, the city’s GIS analysts relied on parcel and centerline data compiled and maintained by the county. Relevant parcel information was obtained from plat maps, and each parcel was assigned a value to denote whether it was a public or private roadway. Analysts then used ArcGIS Desktop to query the public roadway parcels and join them to the street centerline data in the roadway layer.
While developing this layer, analysts discovered that the region has a long history of changing approved roadway widths and realigning streets. They also learned that there was limited data available on roadway conditions. Most historical information was maintained on hanging paper maps, in Mylar plans stored in slide drawers, and on various spreadsheets used throughout the city’s public works department. This hodgepodge data made it difficult to develop a roadway network schema.
To assist with all this, the city’s GIS analysts obtained raster aerial imagery of North Las Vegas from Esri partner Nearmap and stitched the images together in one seamless image database (SID) file using GeoExpress from Esri partner LizardTech (now Extensis). Analysts also reviewed scaled civil design drawings and evaluated historical pavement records to determine roadway dimensions and pavement thickness—something that had never been entered into the city’s GIS. This allowed the analysts to incorporate the condition and thickness of pavement sections as attributes in the roadway network layer.
Street Sweeper Operators Become Data Collectors
The next step was to determine the condition of roadway pavement throughout the city. And the team had an interesting idea: to have street sweepers collect data on road surface conditions.
For street sweeping, North Las Vegas is divided into 16 zones that are cleaned on various days of the week. Each street sweeper is equipped with software that records its location in real time. The idea was to have street sweeper operators use a four-button panel installed in the cab to record pavement conditions as they went over each section of street on their routes. The four-tier visual rating system worked as follows:
- Button 1: Poor/Failed roadway condition
- Button 2: Fair roadway condition
- Button 3: Good roadway condition
- Button 4: Excellent roadway condition
Each time an operator pressed one of these buttons, a ping within the city’s fleet management system provided the location of the sweeper and assigned the corresponding pavement condition to the section of road being driven. When the roadway condition changed, the operator pressed a new button to record another geolocation and register the new roadway condition going forward.
The city’s street sweeper operators generated 30,000 data points on roadway conditions throughout North Las Vegas. These were downloaded as a shapefile in ArcGIS Desktop and combined into a single layer. GIS analysts then spatially joined the shapefile to the street centerline network to obtain a roadway condition for each street. In areas where a street sweeper operator recorded different conditions for each side of the same road, that section of roadway was assigned a single, worst-case condition. Although there were some inconsistencies in the data—such as when a driver identified a pothole on a relatively good street, generating a poor condition rating on an otherwise adequate stretch of tarmac—the results were sufficiently reliable.
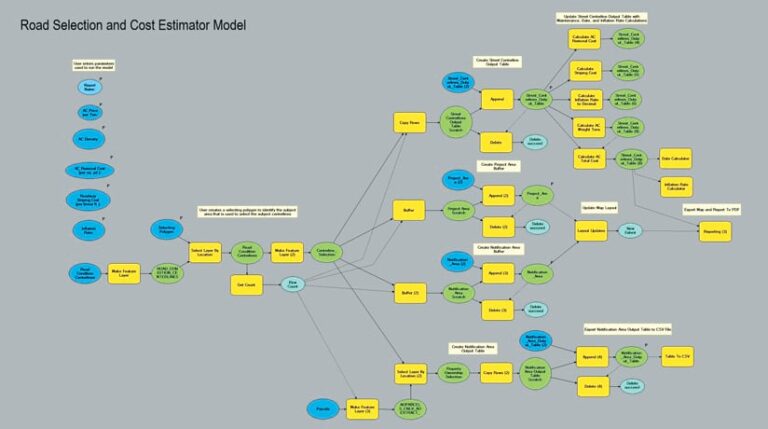
Modeling Future Cost Scenarios
Once the roadway network layer was built, the next step was to figure out what road maintenance and improvement work would cost.
GIS analysts outfitted the roadway network with a typical pavement life cycle cost algorithm and input that into ModelBuilder. The algorithm determines what it would cost to replace sections of pavement based on their condition, dimensions, and thickness—and it even includes an annual escalation factor for increased material prices and labor rates. Having all this in ModelBuilder brought geoprocessing tools and Python scripting together to manage all the data associated with the project, automate and document workflows, and automatically generate maps and reports.
One unique feature of the network model is its ability to outline an individual roadway or residential subdivision and determine the cost to repair or replace it. The algorithm can also evaluate the city’s entire paved roadway network—or any portion of it—and determine the 20-year pavement replacement costs. And with ModelBuilder, staff members throughout the city can run these cost analyses quickly, accurately, and consistently—even if they have little or no GIS experience.
Continually Improving Roads and Technology
Of course, roadway maintenance and improvement are never-ending. Although the data gathered through this project needs some additional vetting and the network still requires refinement, the City of North Las Vegas has already used the information to outline a new five-year Capital Roadway Improvement Plan and determine funding for other roadway projects. The new network can also help the city forecast where new roads will be needed, how much it will cost to maintain those future roadways, and how to optimize their life cycle costs.
Looking ahead, in addition to upgrading to ArcGIS Pro, the City of North Las Vegas is actively exploring how to use deep learning in ArcGIS API for Python to automate the city’s annual road surface condition data gathering operation. The team also plans to share this road condition data more widely via ArcGIS Online and dashboards built with ArcGIS Dashboards. This way, members of the community would be able to monitor the city’s efforts to maintain safe roadways and potentially contribute to identifying roadway hazards that require immediate attention.
For more information about this project, email City of North Las Vegas public works director Dale Daffern at daffernd@cityofnorthlasvegas.com.