In parts one and two of this blog series, you learned how to prepare your environment for deep learning and best practices for using Esri’s pre-trained deep learning models. Now that you have run a model, you are ready to clean up your extracted features by removing objects misidentified as buildings and regularizing your final building polygons.
Two notes before we get into the tools:
- In this blog post we will focus on the output features from the Building Footprint Extraction model, but this workflow can also be adapted for other feature extraction model outputs.
- We will be going over some of the most useful tools that are available to refine your detected buildings output, but note that there are multiple workflows for editing features so this is not an exhaustive list.
Combine Multiple Outputs
In some workflows, you will run the Detect Objects Using Deep Learning geoprocessing tool more than once and need to combine multiple output layers to get your final building polygons. This can be accomplished with the Merge and Dissolve tools as detailed below. If you only have one output from the Detect Objects tool, you can skip these steps and proceed to clipping with parcel data.
- Open your geoprocessing tools and type Merge in the search bar.
- Open Merge (Data Management Tools).
- Add all the inferenced layers to the Input Datasets variable (select in the Contents pane then drag and drop).
- Edit the Output Dataset if needed (note that this is an intermediate layer that you may not need after finishing your QA/QC workflow).
- Leave the other default parameters and run the tool.
Some buildings were likely identified in both your output results. In order to avoid duplicate features, we will dissolve the boundaries between overlapping building polygons to form one polygon for the building.
- Open your geoprocessing tools and type Dissolve Boundaries in the search bar.
- Open Dissolve (GeoAnalytics Desktop Tools).
- Add the output layer from the merge tool above as an Input Feature.
- Edit the Output Dataset if needed.
- Leave the other default parameters and run the tool.
Split up Multipart Features
After completing the steps above for merging and dissolving together multiple building layers, you may notice that in some cases multiple polygons are being grouped together as one building. To ensure that we get each detected building as a single polygon feature, we will run the Multipart to Singlepart tool.
- Open your geoprocessing tools and type Multipart to Singlepart in the search bar.
- Open Multipart to Singlepart (Data Management Tools).
- Add the layer derived from the previous step as an Input Feature.
- Edit the Output Dataset if needed (note that this is an intermediate layer that you might not need after finishing your workflow).
- Run the tool.
Clip to Parcel Data
If you have land parcel polygon data, you can use it to refine your results by removing any “buildings” that we’re identifies by the model but fall outside of the known parcel boundaries. Assuming the parcel data is up to date, buildings outside these bounds are likely false identificcations. If you do not have parcel data, you can skip this step.
- Open your geoprocessing tools and type Clip in the search bar.
- Open Clip (Analysis Tools).
- Add your buildings layer as an Input Feature.
- Add the parcels data as a clip feature
- Edit the Output Dataset if needed (note that this is an intermediate layer that you might not need after finishing your workflow).
- Run the tool.
Remove Misidentified Objects
As described earlier in this process, the deep learning algorithm is never going to output a perfect result. Therefore some smaller features such as cars or piles of construction materials can be identified as buildings. We can remove these non-building polygons based on their area, as their footprints will be often be significantly smaller than a building footprint. In this case we are deleting features smaller than 50 square meters, but this value should be adapted based on the typical size of the object you are looking to detect.
- From the Map tab, under Selection, click Select by Attribute.
- Add the layer derived from the previous step as an Input Rows.
- Leave the Selection type as New selection
- Click + New Expression.
- Click the SQL button on the right above the table to toggle the SQL expression entry
- Enter: Shape_Area < 50.
- Click OK. Now any features under 50 square meters are selected.
- Click the Edit tab, and under Features, click Delete. This will delete your currently selected features.
- Click Save to save your edits.
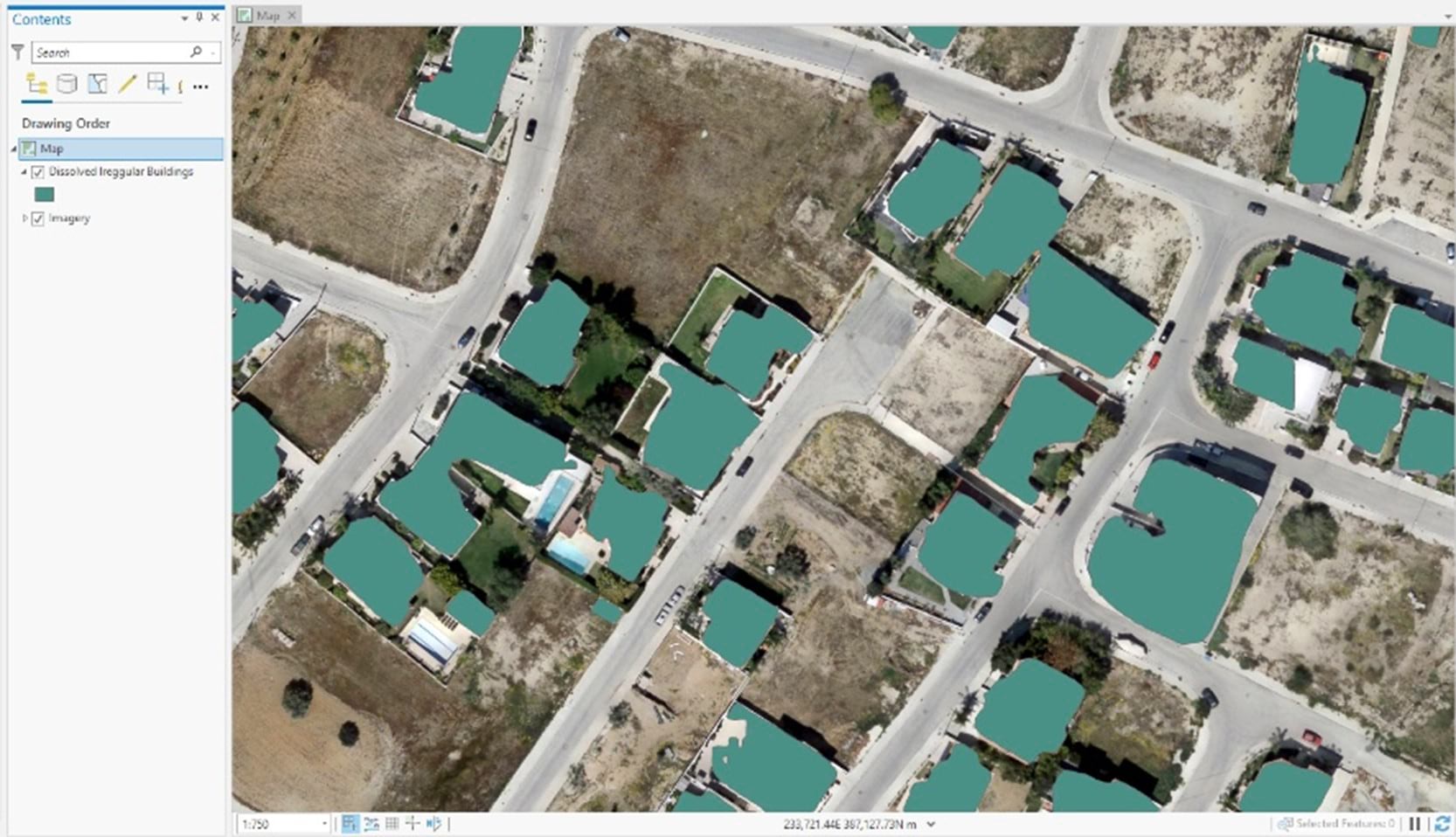
Regularize Buildings Footprints
As you can see in the image above, the shapes of our current building footprints are irregular, lacking the straight sides and right angles typical of buildings. ArcGIS Pro has a geoprocessing tool specifically designed to clean up these outputs and give you more realistic building footprint polygons:
- Open your geoprocessing tools and type Regularize Buildings Footprints in the search bar.
- Open Regularize Buildings Footprints (3d Analyst Tools).
- Add the layer derived from the previous step as an Input Feature.
- Edit the Output Dataset if needed (note that this is an intermediate layer that you might not need after finishing your workflow).
- Leave the Method as default, Right Angles.
- Specify a Tolerance In this exercise, we used a value of 5, which means a segment of the extracted polygon needs to be a minimum of 5 meters in length to be identified as a wall.
- Leave the other values as default.
- Run the tool.
For an additional challenge, you can calculate the typical ratio of length to width of a building in your image, and use this metric to further identify polygons that are not likely to be buildings.
- Use the Bounding Geometry geoprocessing tool to create a rectangle around each feature.
- Get the ratio of the length to width of the rectangle (filter out any anomalies that get a width that is much bigger than a length; this is good to filter out features that don’t have a logical footprint of a building).
- Select and delete these features.
Automate the QA/QC process
For those who enjoy working with Python, the following is a code snippet that will do the above job for you:
Article Discussion: