According to consulting firm Gartner, bad data costs organizations an average of $12.9 million per year. Other reports show similarly staggering figures. The McKinsey Global Institute, for example, found that poor-quality data can lead to a 20 percent decrease in productivity and a 30 percent increase in costs.
The consequences of working with bad data are serious, ranging from diminished customer loyalty, lost revenue, and wasted resources to uninformed decision-making and missed opportunities. Data quality management should be a priority for all businesses. And as data volumes grow, being able to effectively manage data is indispensable. Organizations need to ensure that their data is high quality, meaning it is accurate, complete, consistent, and timely.
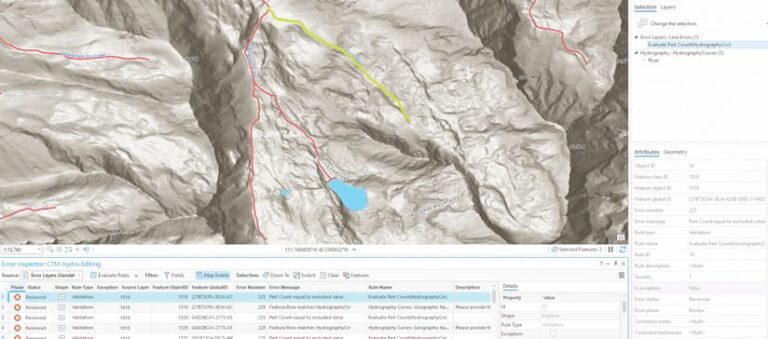
To address the risks that poor-quality data poses to organizations, Esri provides capabilities across the ArcGIS system that support general data quality requirements, as well as focused extensions for comprehensive data quality management. ArcGIS Data Reviewer, in particular, enables organizations in myriad industries to implement comprehensive quality reviews of their data based on their own unique requirements. This allows organizations to better analyze their data; identify patterns; and, ultimately, make data-driven decisions.
Defining Data Quality
One of the challenges facing data producers and consumers alike is defining what constitutes good-quality data. What works for one organization may not be acceptable at another, since quality is defined in the context of how the data will be used in problem-solving. Furthermore, data that is of good quality today may not measure up in the future as business processes and best practices change.
Sophisticated apps rely on high-quality data inputs to generate accurate insight and predictions. And while cutting-edge technology like AI and machine learning models can yield advanced analytics, poor-quality data can lead to biased models, erroneous predictions, and unreliable recommendations. This undermines the effectiveness of AI-driven decision-making.
For many organizations, data quality requirements are based on industry standards, input from subject matter experts, and experience. While these approaches differ from organization to organization, there are standards in place to create a common language when defining quality.
The International Organization for Standardization’s ISO-19157 provides this framework for geographic data. ISO-19157 presents guidelines for how to manage geospatial data quality, focusing on how to assess and improve the quality of geospatial data throughout its life cycle. The standard, which demonstrates how to evaluate and document geospatial data quality, defines various elements that contribute to data quality, such as completeness, positional accuracy, logical consistency, and temporal precision.
Raising Data Quality Standards
Given that many industries have undergone digital transformations, the volume and variety of available data have grown. This increased reliance on data has significantly raised data quality standards, since only high-quality data can be mined to generate insight.
Users of ArcGIS technology can use Data Reviewer to automatically and holistically manage the quality of their spatial data through configurable data checks. The workflows in Data Reviewer support the data quality requirements found across multiple industries. The extension automatically detects poor-quality data, makes it easier to get qualitative feedback from subject matter experts, and includes a framework for tracking corrective actions that improve data quality. The library of ready-to-use data checks provided with Data Reviewer can help identify errors in feature integrity, spatial relationships, attributes, events, polylines, polygons, and z-values.
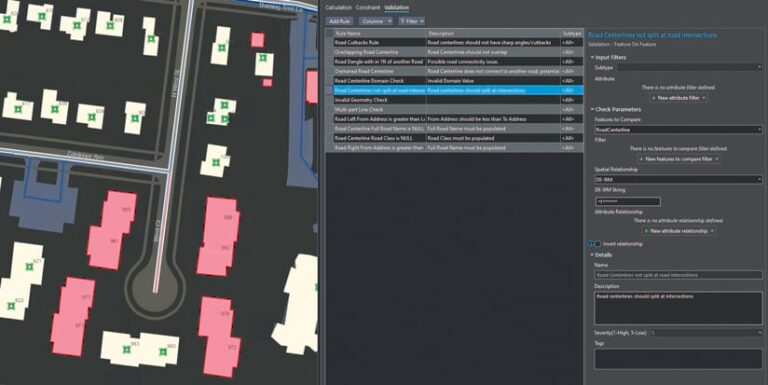
All kinds of organizations—from local governments and public works departments to utilities and water and sanitation districts—use Data Reviewer to improve the quality and reliability of their GIS data. This helps them streamline resources, reduce costs, and enhance productivity and transparency.
Overcoming Data Quality Challenges
One area that industries and governments around the globe are grappling with—and one that relies heavily on high-quality spatial data—is infrastructure improvement. Transportation networks, energy grids, communication channels, and water and wastewater systems the world over are aging. And the challenging operating conditions presented by improving these critical facilities are straining governments and citizens alike.
Many organizations involved in infrastructure improvement are trying to take advantage of technological advancements such as digitization, automation, and AI to fast-track modernization projects. This often requires them to upgrade their legacy systems to the latest solutions that follow newer architecture patterns. System upgrades like these are most often accompanied by data migration and consolidation efforts. These are substantial undertakings, and they need to be done right so that organizations can reap the full benefits of a system overhaul. This means that organizations need to let go of archaic data structures and workflows, which can cause gaps in data that compound over time.
Usually, modernization projects include adopting industry-specific solutions, such as ArcGIS Parcel Fabric or ArcGIS Utility Network, along with their associated data models. In preparation, organizations will often conduct a comprehensive review of their data holdings to determine their fitness for use in these solutions and the workflows they are intended to support. Many organizations employ Data Reviewer during these reviews to identify and document data that does not meet the requirements of their modernized systems.
Investing in Data Quality Management
In today’s competitive environment—with advanced technologies such as AI and machine learning changing the way that so many industries operate—investing in data quality management is essential. By ensuring that their data is accurate, complete, consistent, and timely, businesses can make well-informed decisions, avoid costly mistakes, and improve operational efficiency. And if they adhere to industry-backed data standards and regulations, they can mitigate the risks associated with having incomplete or inconsistent data.
Data Reviewer automates and simplifies the data quality management process for geospatial data. Learn more about ArcGIS Data Reviewer.