For decades, Kuwait has been on the cutting edge of geospatial technology. An early adopter of GIS among countries in the Gulf Cooperation Council (GCC), the government of Kuwait started using GIS in the late 1990s for planning purposes. As the technology evolved and a range of government agencies began implementing GIS, Kuwait’s Public Authority for Civil Information (PACI) emerged as a standout user and is now blazing trails in machine learning.
PACI provides civil identification to commercial and residential establishments across the country and to the people of Kuwait. Because of this, PACI keeps authoritative records of every address, business, and individual in Kuwait.
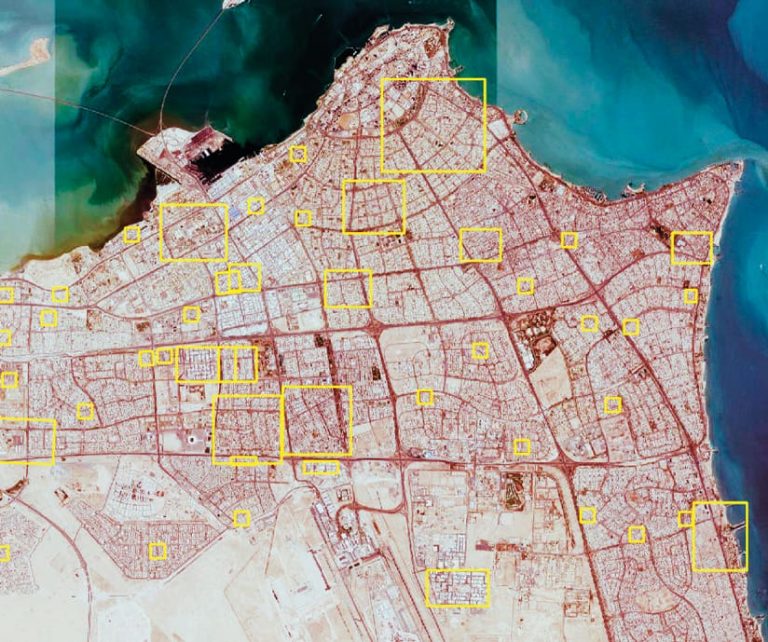
“All these components are linked together with a unique identifier called a PACI number,” explained Maher Abdel Karim, GIS project manager at PACI. “Each parcel or building or unit inside a building is uniquely identified through that [number] all over Kuwait, and there is no duplication.”
Having to keep track of all this data meant that PACI realized early on the importance of incorporating GIS workflows into its business processes. It has also enabled PACI to consistently innovate with GIS.
“We embarked on an enterprise-wide GIS project in 2011, where we converted all our CAD-based papers and digital records into GIS and built a complete enterprise GIS by integrating this with our legacy systems,” said Karim.
That helped PACI create a comprehensive basemap for the entire country. After that, the organization decided to simplify how people could search for addresses and businesses across the country, so it developed a mobile app called Kuwait Finder. The app provides the public with distinctly local information about all the businesses in Kuwait.
“Five years ago, this was a revolutionary application, and we at Esri assisted in developing a supporting, state-of-the-art, back end infrastructure,” said Mansour Raad, Esri’s global chief technologist.
“Kuwait Finder provides people with a hyper-local search engine plus navigation and certain local features, like supermarkets, health centers, or the date and location of a public figure’s funeral proceedings so people can go give their condolences,” said Karim. “It’s a really popular app in Kuwait—the number two app on both Android and iOS.”
But in a fast-growing country with unique geographic features, keeping the basemap that supports Kuwait Finder (along with many other projects) current proved to be a constant, complicated, and time-consuming job. What’s more, for Karim and his team to provide Kuwait Finder as a service, like they wanted to, they had to find a better way to update the basemap.
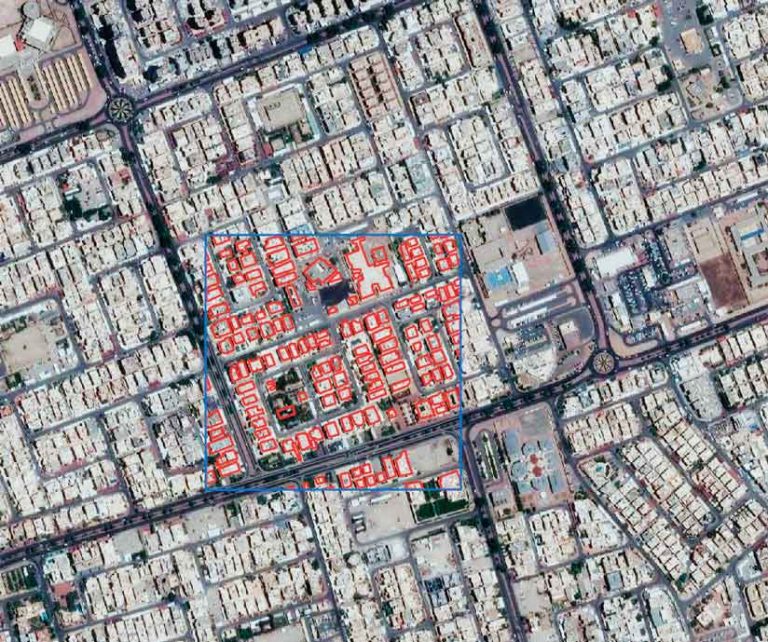
“So over two years ago, Maher goes, ‘What if we use machine learning for that?’” recalled Raad, whose work revolves around advanced geospatial analytics. “At the time, nothing was done using geospatial artificial intelligence, or GeoAI. But he and I developed a way to use satellite imagery to do road and building footprint extraction. This was a huge time-saver and very groundbreaking.”
“The things that PACI is doing are just really transformational for Kuwait as a whole,” said Linda Peters, global business development manager for official statistics at Esri. “Doing this deep learning, PACI is taking a process that used to take five analysts more than a year to complete and running it in less than a week.”
Unique Conditions Require Inventive Solutions
Geographically, Kuwait is quite distinctive, which has given rise to unique-looking infrastructure in and around Kuwait City, where everyone lives. The country’s largely flat and sandy desert landscape gives way to a warm coastline on its eastern edge and small patches of agricultural land in the southeast. Rapid development has been a mainstay of life in Kuwait for decades. In a bid to become a regional and international financial and trade hub by 2035, new construction has soared in recent years.
“Because Kuwait is a burgeoning country, every time you go somewhere, a road will change or a new one will appear that wasn’t there a few months ago,” said Raad. “Keeping up with this is difficult.”
Which is why Karim wanted to use machine learning to extract road data and building footprints from satellite imagery. But aside from the fact that GeoAI was nascent technology at that time, Kuwait faced other issues as well.
“In the United States, you can find high-accuracy satellite imagery and aerial and drone photos. You’ll find a lot of green areas or gardens or structures, and there will be added contrast on the imagery, which can help you distinguish between buildings and the surrounding landscape,” said Karim. “In Kuwait, due to its desert landform, the contrast in the satellite imagery is very weak. So it’s difficult to distinguish between the building and the street.”
Additionally, the shapes of Kuwait’s houses vary a lot, which is not the case in many other countries. And even the shapes of parking lots and streets aren’t standard.
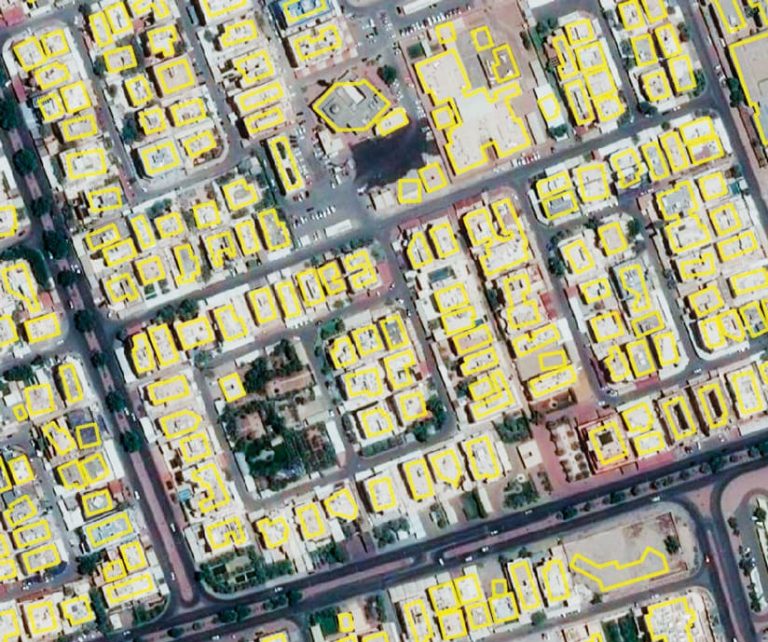
“Another problem was the absence of synchronization between satellite imagery and vector data,” said Karim. “This vector data is needed for ground truthing that PACI can feed into a model to help it learn what various features look like.”
PACI had to start from scratch, creating new ground truth data that includes building footprints, street polygons, and parking lots. These features were used to train the model to detect what to pull from satellite imagery. From there, the team at PACI had to test various models and manually check the GIS features they were producing.
“When we started evaluating the output of the models, we found that it was totally different from what we were looking for,” said Karim.
In short, the models didn’t reflect what Kuwait actually looks like. So the team had to innovate again.
“Since we were already creating the ground truths for buildings and streets and parking lots, we built a model that can provide us with these three feature types,” said Karim.
Then came more training and testing. PACI took a 600-square-kilometer area (about 230 square miles) of satellite imagery of Kuwait and had the model infer where buildings and streets are to provide the prediction raster.
“We ended up taking what Kuwait City looks like, what the desert looks like, what the agricultural areas look like, what the region by the gulf looks like, and we taught the machine those things by explicitly labeling everything,” Raad explained. “Maher and his team spent a lot of time labeling the data, which is very important in machine learning, and it paid dividends.”
“After postprocessing, we got around 111,000 building footprints and around 78,000 street segments, and this took around one and a half hours,” said Karim.
The team then checked the model’s output building by building. By Karim’s estimate, it took just over 48 hours total to do quality control on the data, which reached 97 percent accuracy.
“Before, this would have taken us 119 days, so we are comparing a week to 119 days,” said Karim. “You can imagine the productivity increase by applying machine learning and deep learning models to automate the GIS workflow for basemap updates.”
Forging Ahead with New Ideas
While the project has come a long way in two years, it is still a work in progress.
“We are still trying to automate as much as we can from this process in order to reach the point that we minimize manual efforts and manual processes,” said Karim. “We are trying to enhance our models and our workflows to achieve this.”
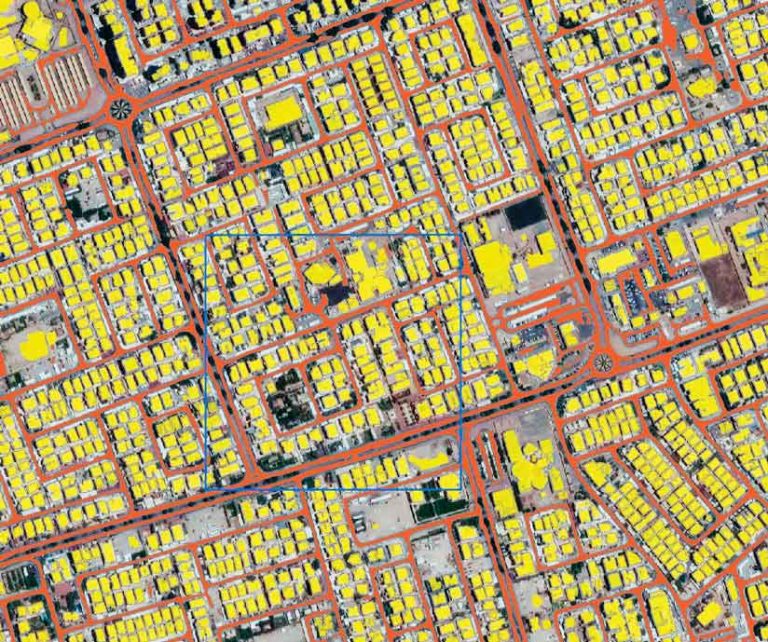
At the same time, Karim and his team are already working on adapting machine learning to new projects—namely, to 3D, street-level imagery.
PACI currently has its own street-level imagery of the whole country and serves it to the public via Kuwait Finder. As with the basemap, this street-level imagery needs to be updated regularly. So what Karim is working on now is how to extract features from this street-level imagery to enrich PACI’s GIS layers.
“In order to use deep learning to do this, we decided to create a new training dataset based on the imagery that was captured in Kuwait,” said Karim. “We currently have around 41 different classes, like cars, buses, people, manholes, traffic signs, stop signs, and traffic lights. So we are combining these 41 classes…and converting them back to GIS.”
“All this is really avant-garde,” said Raad. “Maher is very forward thinking about what he needs to do.”
And he has a great team behind him, whose members believe in extending the benefits of GIS not only across government agencies but throughout Kuwait as well.
“PACI has one of the best GIS teams that works on a range of different projects—not only keeping data updated but also providing lidar surveys; doing machine learning; updating applications, like the mobile apps and Kuwait Finder; creating different information products; and supporting other government agencies,” said Raad. “PACI has been bold enough to embrace the latest developments and cutting-edge technologies, which has helped the country in general understand how effectively GIS technology can be implemented.”