Take the internet. Despite its status as the most transformative technology of our lifetime, the internet provides a simple economic benefit—it makes searching for information and communications cheaper. Artificial intelligence (AI)—the hottest technology on the block—has equally simple economics. It lowers the cost of prediction and diagnosis. And although blockchain is still an emerging technology, its economics are clear. It reduces transaction verification costs and the cost of operating a marketplace without a central authority.
Cheap creates demand. Cheap drives opportunities. Economics tells us that when the price of something drops, we tend to use more of it. And if that something is particularly valuable, it can have a dramatic impact. That’s why understanding a technology’s basic economics is such a great way to cut through the hype. It distills the value proposition to its essence—sharpening our understanding of how a technology impacts people and organizations.
It’s through this lens that I examine geospatial technology. Today we’re seeing an explosion of interest in digital maps and spatial analytics. Just look at the proliferation of map-based dashboards and contact-tracing apps that have surfaced in response to COVID-19. But pandemic-induced hype aside, there’s currently a real and material surge of interest across the board for geotech. In fact, 2020 Location Intelligence Market Study, a report by the Dresner Advisory Services, noted that half of organizations consider geospatial technology a critical or very important enabler of their business agenda. The mobile mapping market, for instance, has seen particularly strong growth and is set to grow by 15 percent per year to over $40 billion by 2024, according to research done by Global Market Insights, Inc. And that’s just one segment of the geospatial industry.
It begs the questions: What are the economics behind the growth? What valuable activity or ability does geospatial technology make cheaper? And what are the implications for people and organizations?
Cost of Spatial Awareness
Geospatial technology is a catchall for the range of modern tools that enable mapping and spatial analysis. These include established technologies like GIS, GPS, GNSS, and remote sensing, as well as the new wave of digital technologies that acquire, consume, and process location-based data. Given that it is generally asserted that 60 to 80 percent of all data contains a spatial element, the extent of geospatial technology is vast.
The key insight is that geospatial technology provides something quite simple—spatial awareness. It helps people understand where things happen, where they might happen next, and why they happen where they do.
On a personal level, spatial awareness is obviously important. We all need some level of geographic understanding to participate in society and go about our day. Whether it’s getting to work, deciding where to live, or detecting potential hazards, spatial awareness is an important ability.
For organizations, however, spatial awareness is important on another level. The interests and assets of virtually any organization vary significantly by location. Whether it’s customer spending habits or shipping routes, the factors that impact a company’s fortunes differ in character and complexity from place to place. Spatial awareness is critical to the viability and long-term sustainability of essentially any organization—or society, for that matter.
Without technology, spatial awareness is difficult, to say the least. The manual effort required to compile information about customers, competitors, suppliers, society, and the environment, and then analyze and map the spatial relationships is arduous, complicated, and time-consuming. All this to say, it’s very costly. Geospatial technology overcomes these challenges.
In simple economic terms, geospatial technology makes spatial awareness cheaper.
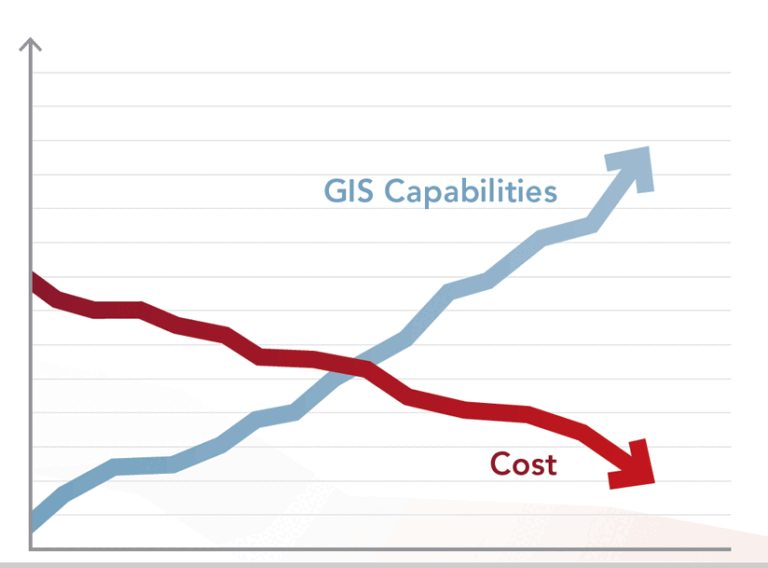
Cost Drivers: Data + Machine Learning
What’s driving the recent surge in demand? Geospatial technology has been around a long time, so this recent uptick suggests that certain new advances are accelerating the drop in the cost of spatial awareness. In fact, that’s exactly what’s happening, and two recent developments stand out: the explosion of accessible spatial data and the rise of machine learning.
When you put them together—more readily available spatial data and cheaper, faster AI-driven spatial analysis— you get better spatial awareness at a better price.
Humankind’s current rate of data creation is staggering. Some projections have the world’s data doubling every two years, and by 2025 doubling every 12 hours. That’s a mind-boggling amount of information, and most of it is spatial. Several factors are contributing to the growth:
- Advancements in traditional spatial data acquisition methods such as satellite imagery, aerial photography, surveying, and remote sensing
- Innovations in new acquisition technology such as drones and vehicle trackers
- Growth of in-situ sensor technology (the so-called Internet of Things)
- A massive influx of crowdsourced data generated by mobile mapping solutions and social media applications (including geotagged photos, videos, and text messages)
- The emergence of open data platforms from government agencies and businesses, allowing for the creation and sharing of raw spatial data as well as derived works such as thematic maps and analytics dashboards, which themselves become sources of spatial data
The net effect is that access to spatial data is byte-for-byte easier and cheaper than ever. And on that basis alone, we’re seeing people and businesses take advantage of this. But data is only one side of the equation. The other side relates to how spatial data is analyzed. This is where AI and machine learning enter the picture.
Machine learning gets around the problem of human involvement in analysis. I don’t say that to sound flippant, but certain aspects of spatial analysis are intractable—specifically, selecting variables. Machine learning uses prediction-based techniques to identify the most relevant variables to include in its statistical models. This is done based on how closely the variables produce an expected outcome derived from a training dataset. A great example is how insurance companies are using machine learning to identify property damaged in a storm. The machine learning model is fed a series of datasets containing images of damaged and undamaged properties. The AI then develops a pattern recognition model built from variables of its choosing. Based on the model, the AI predicts which properties appear to be damaged and which don’t. For a human to do this, it would be very time-consuming. AI makes this process a snap and is usually much more accurate.
When you put these benefits together—more readily available spatial data and cheaper, faster AI-driven spatial analysis—you get better spatial awareness at a better price.
Implications for People and Organizations
What does cheaper spatial awareness mean for people and organizations? When something valuable gets cheaper, economics tells us two things happen: (1) people start using it in nontraditional ways, and (2) other factors change in value depending on whether they’re complements or substitutes.
The Expanded Role of Spatial Awareness
As new machine learning techniques and ubiquitous spatial data lower the barriers to spatial awareness, we’ll start to see a deeper application of geography to long-standing problems. Consider human movement. Understanding why people travel from one location to another is notoriously difficult. Much of this understanding depends on scale—are we interested in short-term, localized movements, or longer-term, regional migration patterns. In both cases, the motivating factors are varied and complex. That’s why predicting where people will travel to is so hard.
Recently, we’ve seen advances in both cases. On the local scale, researchers have developed spatial AI models to monitor street cameras and adjust traffic lights to help disperse crowds after live public events. Traditional methods of planning exit routes are burdened by trying to account for all the possible reasons people go one direction or another, and on-the-ground crowd control tactics can be inefficient and quickly overwhelmed. The new approach analyzes real-time video to detect areas where crowds are forming and predicts which routes would best ease congestion. Controllers can then adjust traffic lights accordingly. The AI provides a much more dynamic level of spatial awareness, and actively enables public safety.
On the global scale, researchers have—for the first time—developed models that predict international migration patterns resulting from climate poverty. The models incorporated environmental, socioeconomic, and political factors into different climate scenarios that could affect regions of Central America. Included in the models were factors like carbon concentrations, gross domestic product (GDP), and border management. Over 10 billion data points were fed to a supercomputer, which developed predictions of how different populations would respond to different climate change scenarios. The models were compared against historical migration patterns to assess and refine their accuracy.
The result was five migration projections that illustrate the profound impact of climate change on population migration—specifically how it could drive the displacement of huge populations in the next 50 years. In the past, projections like these were impossible considering the cost of computing and data acquisition, and the relative immaturity of spatial modeling algorithms. In short, the price of spatial awareness was just too high. In the future, we will almost certainly see more of these breakthroughs.
Complements and Substitutes
When the cost of something important—like spatial awareness—changes economics tells us that the value of its complements and substitutes also changes.
Complements are goods or services that experience joint demand. Generally, as demand for one thing increases, demand for its complement also increases. Think of the way apps increase in popularity in conjunction with the rise of smartphones, or how pasta sauce demand goes up when demand for the pasta itself increases.
An obvious complement to AI-driven spatial awareness is the judgment-based skills needed to capitalize on the analysis it generates. In the future, activities such as sophisticated cartographic visualization, geodesign, data quality assurance, and spatial storytelling will be in high demand as organizations focus on making decisions informed by improved awareness. Also, there will be demand for the applications of ethics, social justice, and economic impact analysis, as these require deep consideration of subtle relationships that are currently the domain of human cognition.
On the other hand, demand for some activities will almost certainly decrease as machine-based analysis and data processing increases in popularity. These are called substitutes in economic parlance. As geospatial technology evolves, we’ll likely see a decline in roles that are readily substituted by the AI-driven model. These include manual, labor-intensive tasks like field data collection and basic map production, as well as spatial analyses that are outperformed by automated machine learning methods. Essentially any activity in a spatial workflow that doesn’t require human reasoning is facing replacement by AI.
Understanding the economics of geospatial technology isn’t a crystal ball, but it does help surface some possible implications. Specifically, the increasing availability of spatial data and the speed and accuracy of machine learning will dramatically enhance an organization’s location intelligence. This will lead to a much richer understanding of its business dynamics. In turn, demand will grow for competencies and tools that help managers develop business strategies that better reflect spatial reality. It’s an exciting time for the geospatial industry. Your best move is to avoid the hype and get prepared.