Co-Authors: Catherine Bohn, Paul Dean & Siddharth Pandey, Dewberry subject matter experts
The aftermath of a hurricane can leave structural devastation in its wake. For the residents of the affected community, it is vital to quickly determine the impact to building structures in order to keep residents safe. Dewberry, an architectural and engineering firm, performs geospatial damage assessments for government clients following natural disasters. These assessments provide important information needed by federal, state and local emergency responders to make critical decisions regarding resource allocation during recovery and rebuilding efforts. Advancements in the field of machine learning (ML) are playing an increasingly important role in performing damage assessments as they apply ML for proactive disaster response.
How does ML support geospatial damage assessments?
Collect imagery from data providers
The predictive benefits of machine learning begin with data collection. Dewberry collects post-event imagery of hurricane and other natural disaster damage from data providers such as the National Oceanic and Atmospheric Administration (NOAA), Civil Air Patrol, and the National Insurance Crime Bureau. The data is uploaded into Esri’s Imagery Data Management System (ArcGIS Image Server) and is added to a compilation of imagery from previous natural disasters. It is then uploaded into a repository of data across various geographies. The data feeds the ML model, which begins to learn to identify patterns of disaster damage to help them to predict what structures will be affected by future disasters.
AI for automation
ArcGIS provides end-to-end deep learning capabilities, enabling users to label, train and perform feature extraction on their imagery. Using ArcGIS Image Analyst extension for ArcGIS Pro, Dewberry trains deep learning models to detect specific (and relevant) features within the imagery.
Labelling features
Prior to training a deep learning model, training samples using high-resolution imagery must be created to represent features of interest. Using the labelling capabilities in ArcGIS Pro, Dewberry digitizes features of interest such as: downed trees, building classifications (damaged and undamaged) and tarps covering holes in roofs. The specified labelled features of interest are housed in a feature class, ready to be referenced in training the model.
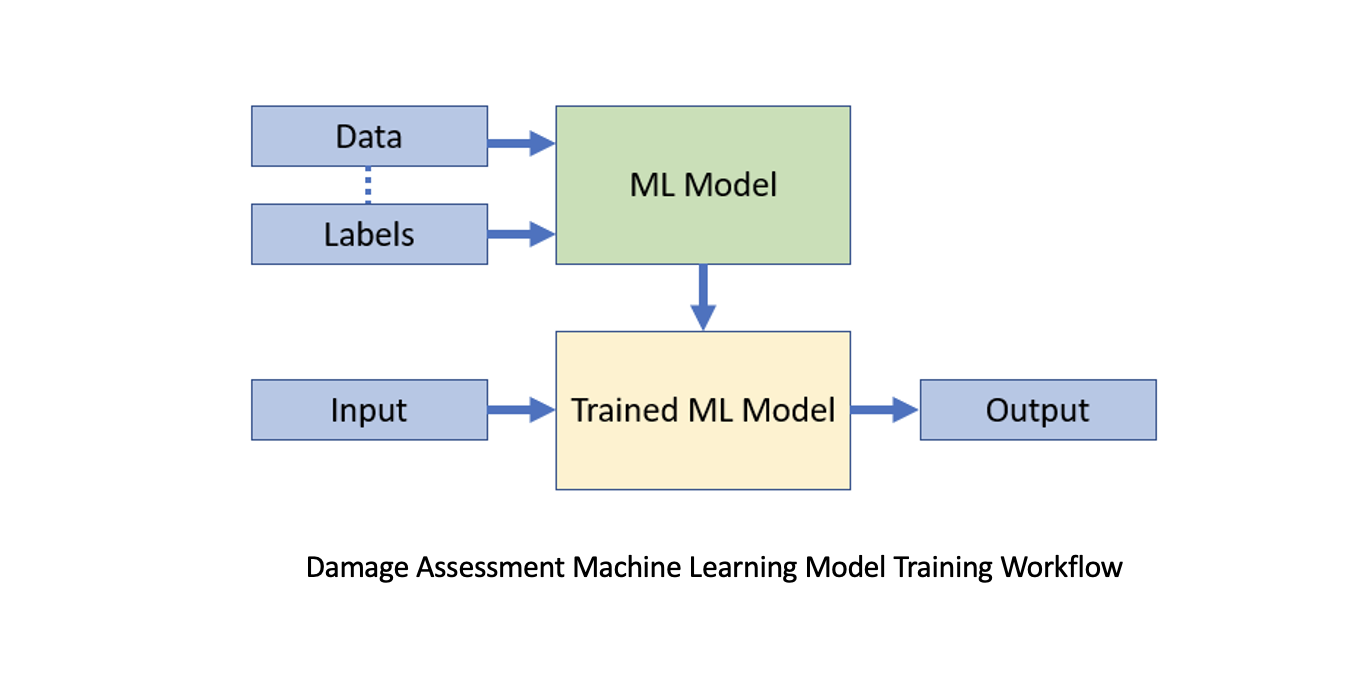
Train deep learning models
Once a training samples are collected, Dewberry trains models relevant to the problem set using the “Training Deep Learning Model” geoprocessing tool in ArcGIS Pro. Key models Dewberry has trained include:
- Object detection model to identify damaged tree stumps
- Object detection model to identify blue tarps
- Object classification model to classify structures at different levels of severity
The ground truth labels are then compared to the model classification results to get a quick qualitative idea of how well the model performed.
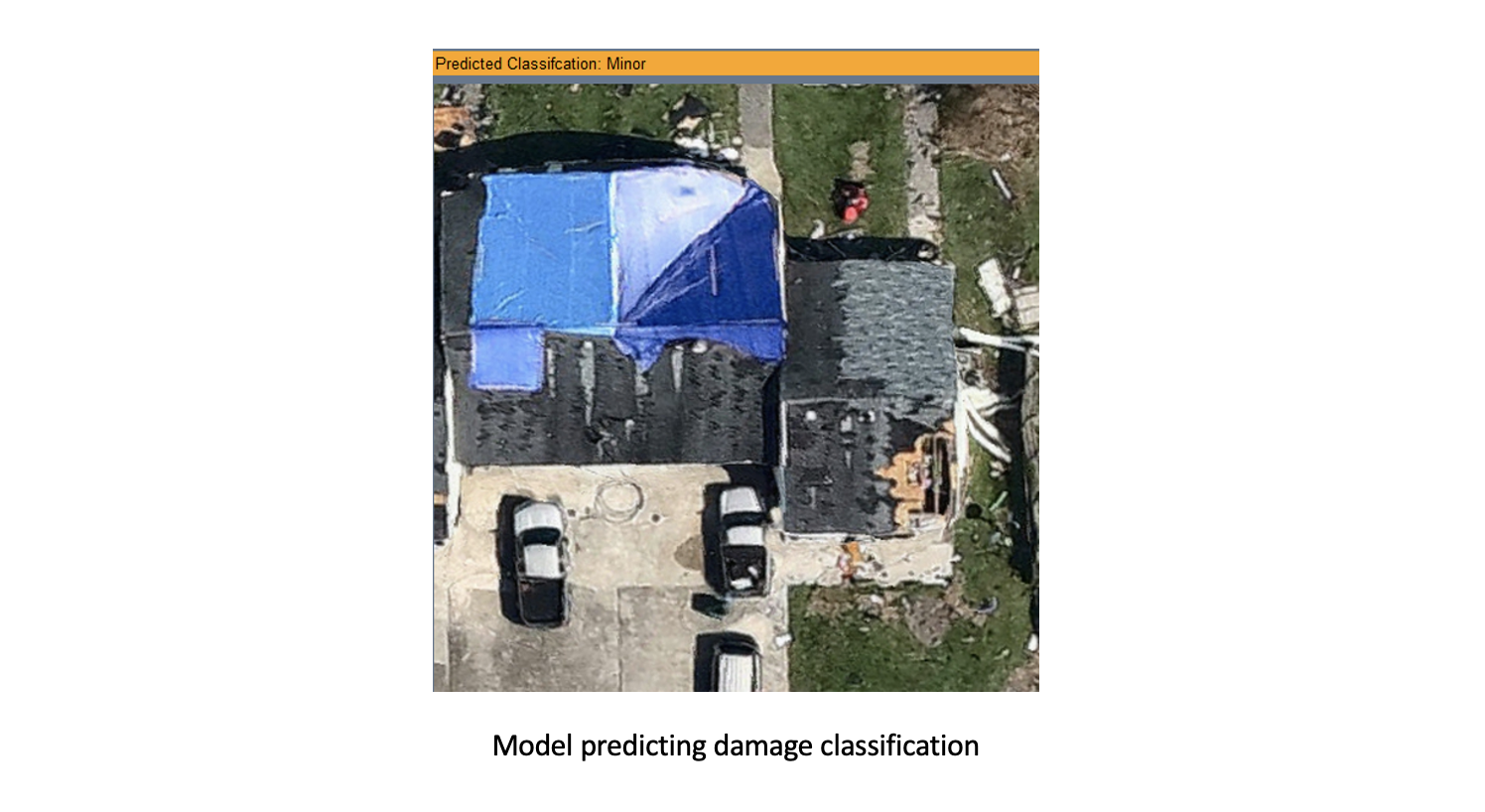
Perform damage assessments
Using the trained models with the inferencing tools in ArcGIS Pro, Dewberry then identifies damaged property structures and classifies the severity of the damage. The labels are also communicated to the Federal Emergency Management Agency (FEMA) for downstream analytical applications.
Before Esri’s ML technology was available, a Dewberry analyst visually interpreted post-event imagery, performing an average of 100 damage assessments per hour. Using trained deep learning models, Dewberry can now assess geospatial damage by completing 410,000 automated assessments per hour. This significant improvement confirms ML’s benefit to both interpret data with greater efficiency and increases the ability to extract a variety of data points.
Report findings to FEMA
Once feature extraction with damage classification is complete, the results are reported to FEMA. FEMA subsequently depicts the results through operational dashboards, providing actionable insights to enable multiple stakeholders to make timely and informed decisions. The dashboards allow viewers to assess the damage label and proactively dispatch necessary aid.
Downstream Analytical Applications
ArcGIS goes beyond just extracting features using AI. It enables users to leverage the power of a complete geospatial suite of tools and applications to perform further downstream analysis. Dewberry’s damage classification in disaster sites is paving the path for additional analyses such as:
Debris estimation modeling
Following the Kentucky tornadoes in 2021, Dewberry reviewed information generated from damage assessments and input it into a debris estimation model. The model enabled analysts to determine approximately how much debris was generated from the damaged structures. Using the Model Builder and Summarize tools in ArcGIS Pro, the aggregated data was provided to the county to facilitate resource allocation for debris removal.
Identifying temporary housing sites
Following Tropical Storm Ida in Louisiana in 2021, Dewberry was asked to identify temporary housing locations for disaster survivors displaced from their homes, preferably close to the original communities as doing so supports a faster revitalization of the affected region’s economy.
Dewberry combined their damage assessments with tools provided in the ArcGIS Network Analyst extension for ArcGIS Pro and added in parcel data and damage assessment information to display hotspots where damage occurred. As a result, they identified large parcels of land which remained unaffected to place the temporary housing sites.
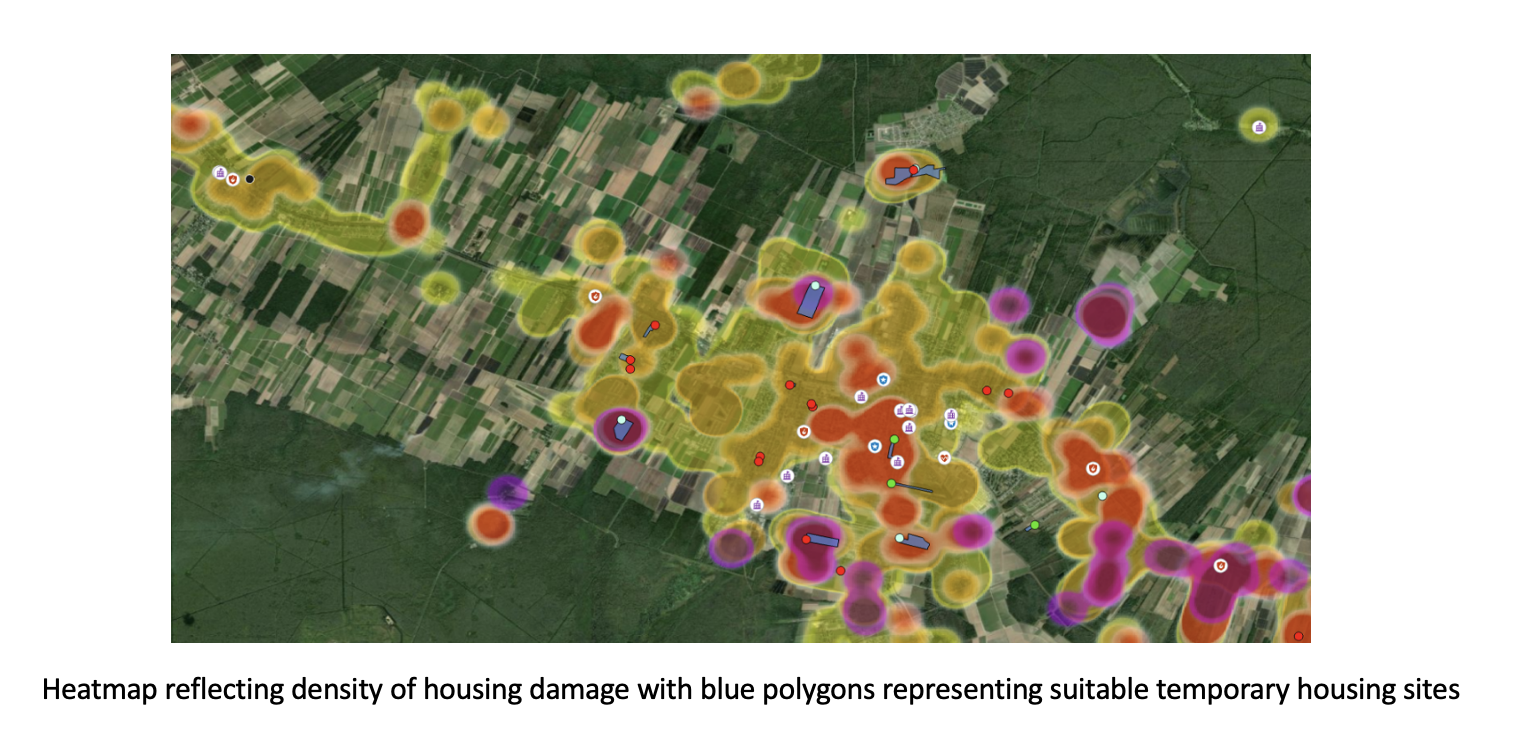
Summary
Dewberry is leveraging powerful geospatial tools and ML capabilities to facilitate efficient response and recovery in times of critical need. Using ML-powered geospatial damage assessments, they are developing and implementing action plans to help communities better equip themselves to respond to future natural disasters. With upcoming enhancements such as identifying non-structure damages (e.g., power lines, roads, bridges) and supporting other forms of disaster events, Dewberry is maximizing the potential of machine learning.
Article Discussion: