Pretrained deep learning models can save you a heap of time! With the ever-increasing volume of imagery that’s available today from a variety of platforms and sensors, the opportunity to leverage simple AI models to automate feature extraction is very appealing. To help your organization readily access this wealth of information, Esri has released several ready-to-use pretrained deep learning models on ArcGIS Living Atlas of the World.
Within this collection of imagery-feature extraction models, there are several that are specifically useful to the Energy Industry. From detecting new well pads in the Permian Basin and extracting new building footprints along pipeline rights-of-way, to extracting your field access roads – these and several more are available for use today.
For some time, with the existing capabilities in ArcGIS, you’ve been able to train dozens of deep learning models on geospatial datasets and derive information products using the ArcGIS API for Python or ArcGIS Pro, and scale up processing using ArcGIS Image Server. The challenge has been you needed enough data, and the resources and time to train the models, but these newly released models are a game changer!
They have been pretrained by Esri on huge volumes of data and in many cases can be readily used to automate the tedious task of digitizing and extracting geographical features from satellite imagery and point cloud datasets. So, they more readily enable the power of AI and deep learning for the your user community, today! What’s more, these deep learning models are accessible for anyone with an ArcGIS Online subscription at no additional cost.
Using the models
Using these models is simple. You can use geoprocessing tools (such as the Detect Objects Using Deep Learning tool) in ArcGIS Pro with the imagery models. Simply, point the tool to the imagery and the downloaded model, and that’s about it – deep learning has never been this easy! A GPU, though not necessary, can help speed things up, and with ArcGIS Enterprise, you can scale up the inferencing too using Image Server.
You can also consume these models directly in ArcGIS Online with ArcGIS Image for ArcGIS Online which is a complete software as a service (SaaS) offering for hosting, analyzing, and streaming imagery and raster collections all without an ArcGIS Enterprise deployment.
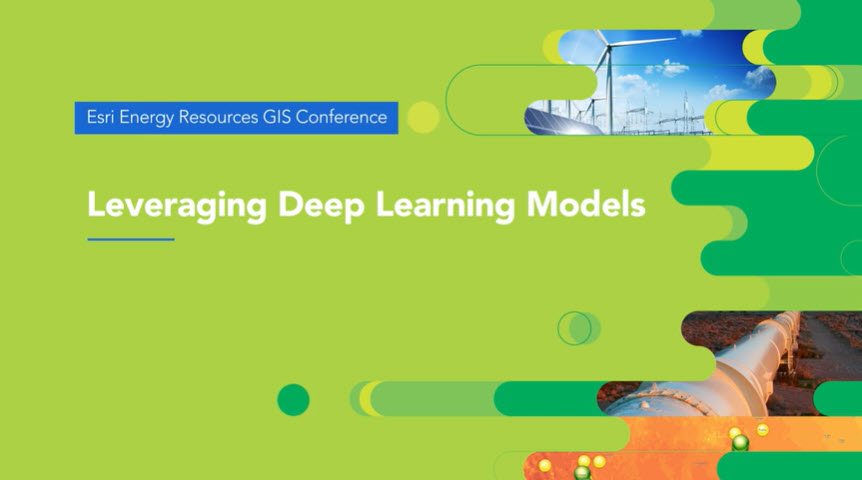
How can you benefit from these deep learning models?
It probably goes without saying that manually extracting features from imagery—like digitizing footprints or generating land cover maps—is hugely time-consuming. Deep-learning automates much of the process and significantly minimizes the manual interaction needed to create these products. However, training your own deep learning model can be complicated – it needs a lot of data, extensive computing resources, and at least some knowledge of how deep-learning works.
With these ready-to-use models, you no longer need to invest time and energy into manually extracting features or training your own deep-learning model. These models have been trained on data from a variety of geographies and work well across them and will continue to improve them over time. As new imagery comes in, you can readily extract features at the click of a button, and produce layers of GIS datasets for mapping, visualization and analysis.
Get to know some of the models most useful for Energy
There are now 30+ models that have been published to ArcGIS Online. These models are available as deep-learning packages (DLPKs) that can be used with ArcGIS Pro, Image for ArcGIS Online, ArcGIS Enterprise with Image Server and the ArcGIS API for Python.
I encourage you to browse all of them as you have the time, however I’d like to introduce a few of the models perhaps most interesting to Energy here:
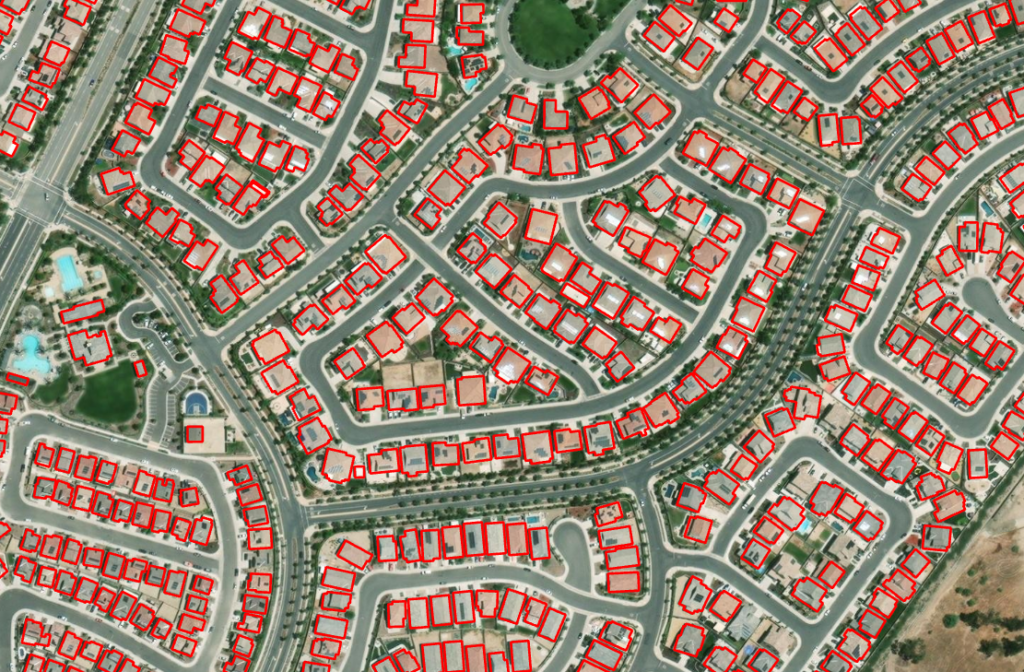
1. The Building Footprint Extraction model is used to extract building footprints from high resolution satellite imagery. While it’s designed for the contiguous United States, it performs fairly-well in other parts of the globe. There are model versions optimized for Africa and Australia as well.
Here’s a story map presenting some of the results. Building footprint layers are useful for creating base maps and in analysis workflows for encroachment analysis, change detection, and infrastructure planning. Here’s a video that runs through the workflow in ArcGIS Pro.
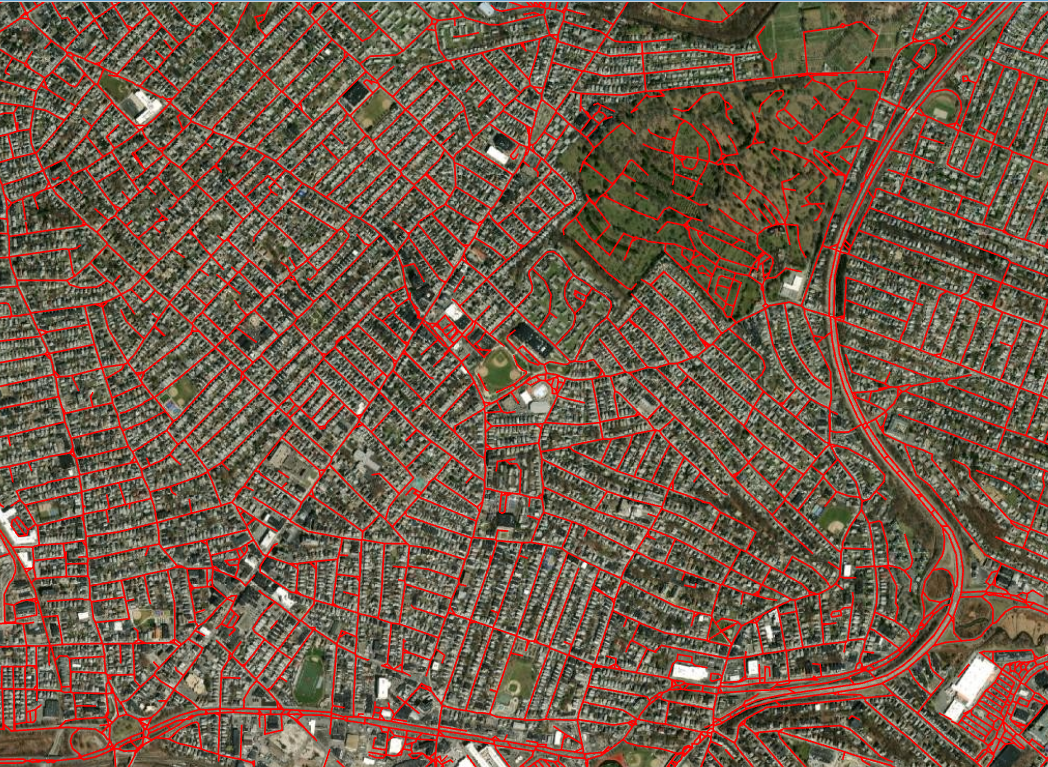
2. The Road Extraction model can be used to extract roads from high resolution (1 meter) aerial/satellite imagery. Road layers are useful in preparing base maps and analysis workflows for field planning and development, change detection, routing, and a variety of other applications. There is also a global roads model available.
Extracting road networks from satellite images often produces fragmented road segments when a semantic segmentation model such as U-Net is used. This is because satellite images pose difficulties in road extraction due to occlusion caused by trees, buildings (in off-nadir imagery), and shadows. This model uses multitask learning, which is inspired by how humans annotate roads by tracing them at specific orientations.
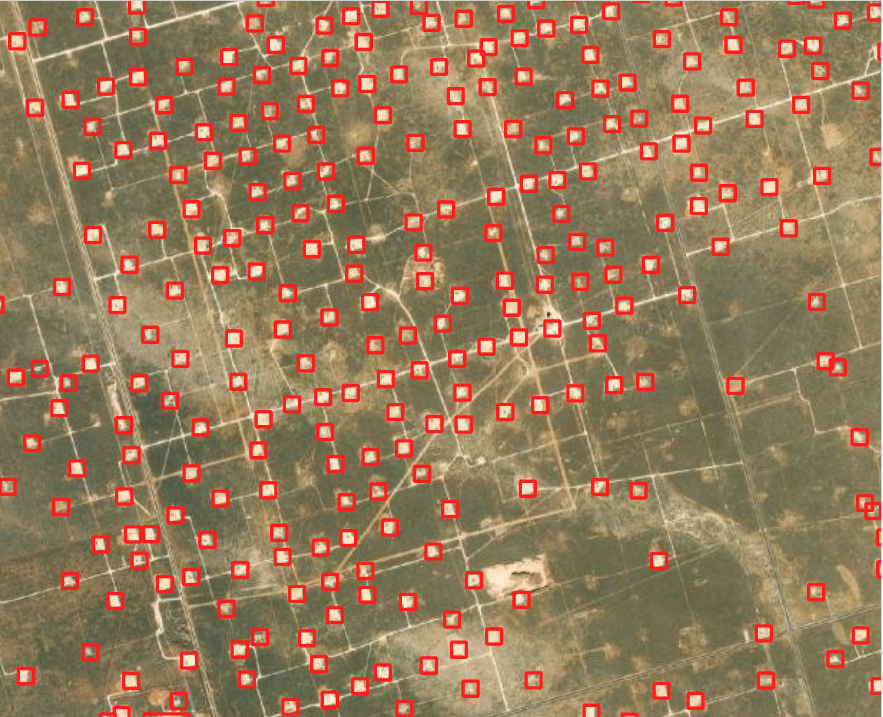
3. Well Pad Detection (Permian Basin) can be used to automate the detection of well pads in an area, used to monitor the progress of new drilling, or help perhaps provide competitive intelligence of activity in an area. This model is optimized for the Permian Basin and runs on freely available Sentinel-2 data. This model could be tuned to work in other regions as well.
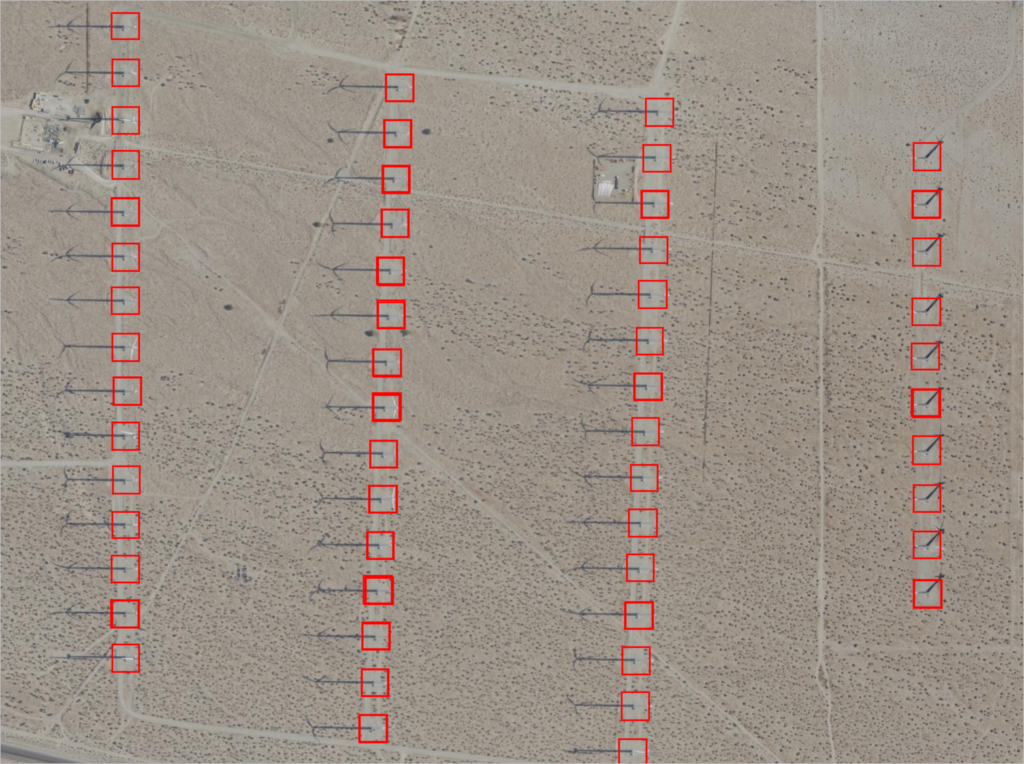
4. As the energy transition continues, we are seeing requests for a variety of analytics in this space. There are now published models for detecting Wind Turbines and Solar Photovoltaic Parks if you are looking for utility scale information, or maybe tracking solar panels on individual homes is of interest.
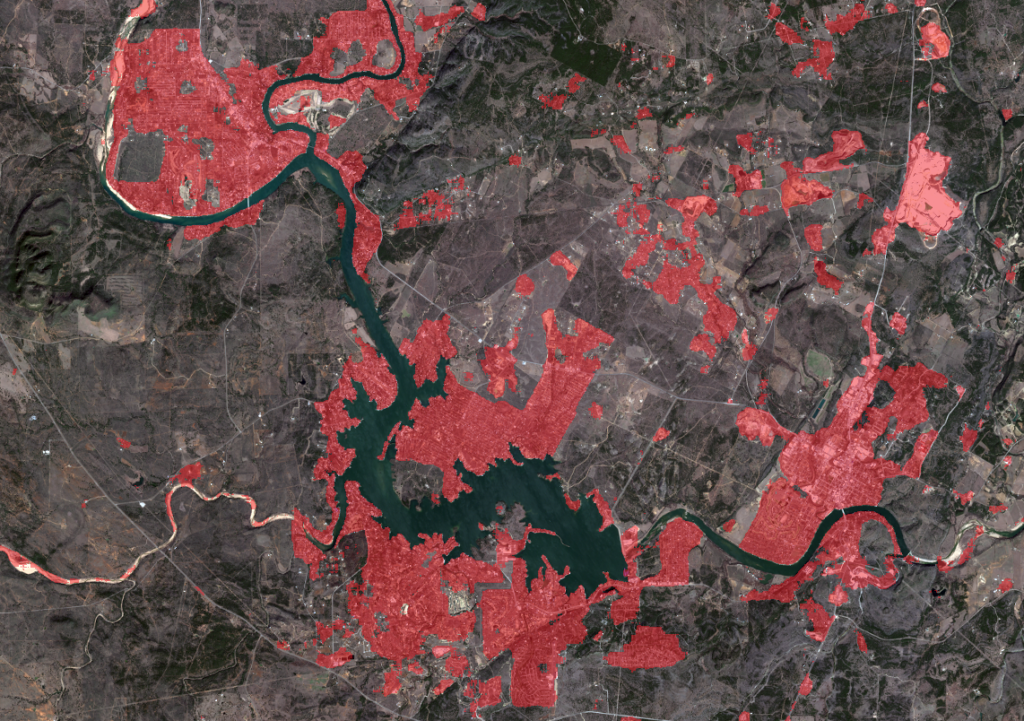
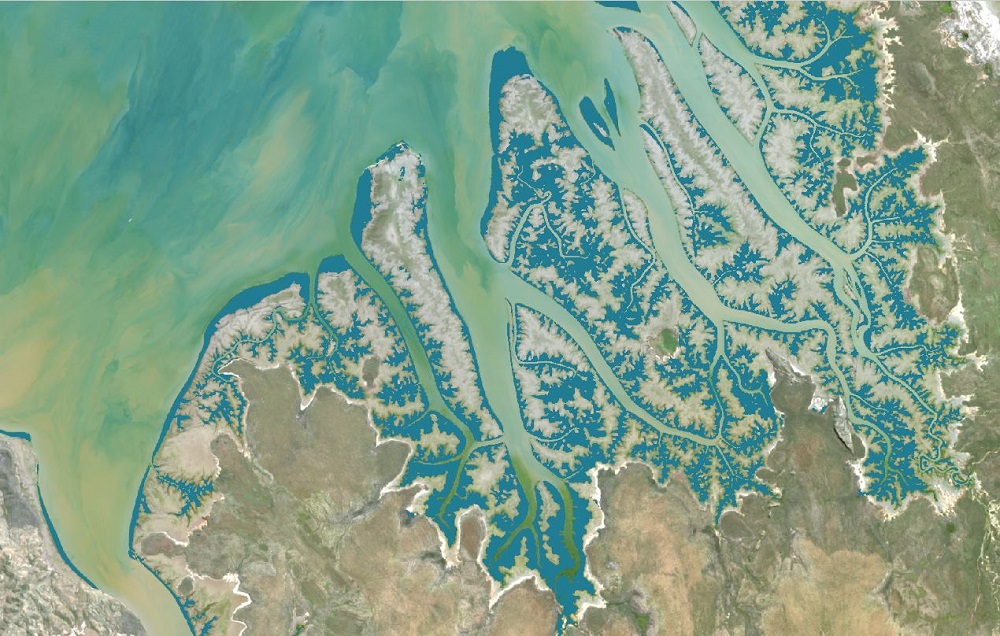
5. The last few models I’d like to mention are in support of sustainable operations. Every organization wants to minimize their impact and protect the communities and environment they work within. Several models have been published to help with this such as:
- Land Cover Classification (Sentinel-2 & Landsat 8) which let you track land use and development over time.
- Human Settlements Classification models (Sentinel-2 & Landsat 8) which may give you insights to population movements or indigenous population settlements.
- Mangrove Classification (Landsat-9) which helps you identify these critical areas for the maintenance and conservation of healthy coastal ecosystems.
Each of these models enable you to take imagery you may already be collecting today and extract new highly valuable information which helps support your current business workflows
There are a few more models we have tagged to this Gallery that may also be of interest to your Energy work. Check back often for additions or continue to browse the full list of models on ArcGIS Online.
Next Steps
Learn more about pretrained deep learning models and check out the models in the ArcGIS Living Atlas for yourself. Read more detailed instructions for using the deep learning models in ArcGIS. Have questions? Let us know, drop me a line, or put a note on GeoNet to share how they are working for you, and which other feature extraction tasks you’d like AI to do for you!