ArcGIS Image Analyst extension for ArcGIS Pro 3.0 release is introducing new Synthetic Aperture Radar (SAR) processing tools as well as enhancements to deep learning, change detection, multidimensional analysis, and stereo mapping.
Radar
In this release, we are introducing nine new synthetic aperture radar (SAR) geoprocessing tools to support processing Sentinel-1 Ground Range Detected (GRD) data. These tools form an end-to-end processing pipeline to produce analysis-ready SAR datasets and false color composites for easier interpretation of images. The analysis-ready SAR data can be explored and analyzed using existing tools and capabilities in ArcGIS Image Analyst such as image classification, change detection, and deep learning workflows.
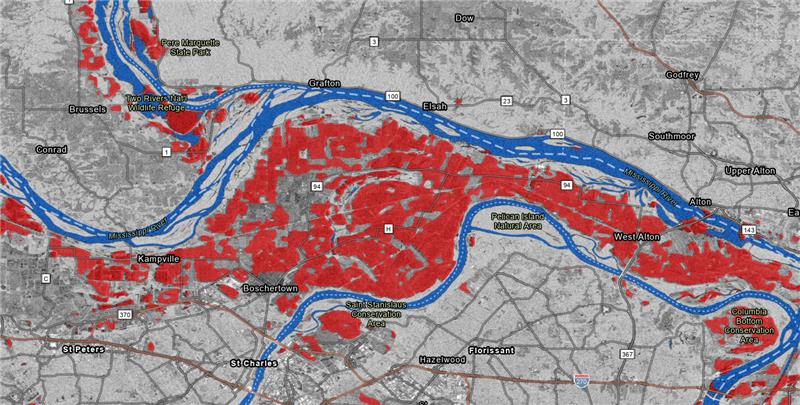
Deep Learning
This release also introduced several enhancements to our deep learning capabilities. We have integrated our tools with popular open-source libraries for machine learning and deep learning, ensuring that users have access to the latest innovations in this rapidly evolving field.
Tools
Detection and Tracking
Updates have been made to detect and track features in a video. With full motion video, users previously needed to pause recording to draw bounding boxes around moving objects of interest. Now, this is made easier with “Click to Track” which allows users to simply click on the object of interest and a deep learning model automatically begins tracking the moving object. In addition to this, this release pairs our tracking models with detection so users can track and detect objects simultaneously.
Performance Enhancements
One of the most time-consuming parts of the deep learning workflow is the creation and preparation of training data; traditionally, exporting massive training data has been tedious, taking more time and effort. With enhancements in this release, Export Training Data for Deep Learning tool now allows users to take advantage of all the processing power on their machine, leading to improved export performance by up to 9 times.
New Deep Learning Models
Typically, deep learning has required vast amounts of training data; however, in recent years, the field is slowly shifting towards Few Shot Detection (training a model with only a few samples). We have added DETReg – an object detection model that is designed to work well when users do not have a lot of training samples. This minimizes time dedicated to collecting vast amounts of training samples and allows users to integrate deep learning into their workflows with ease.
Furthermore, based on user request, a new model type to simplify user workflows is being introduced: Panoptic Segmentation. This model type allows users to run inferencing on the output from both pixel classification and object detection simultaneously so users can leverage a single powerful model to perform both things.
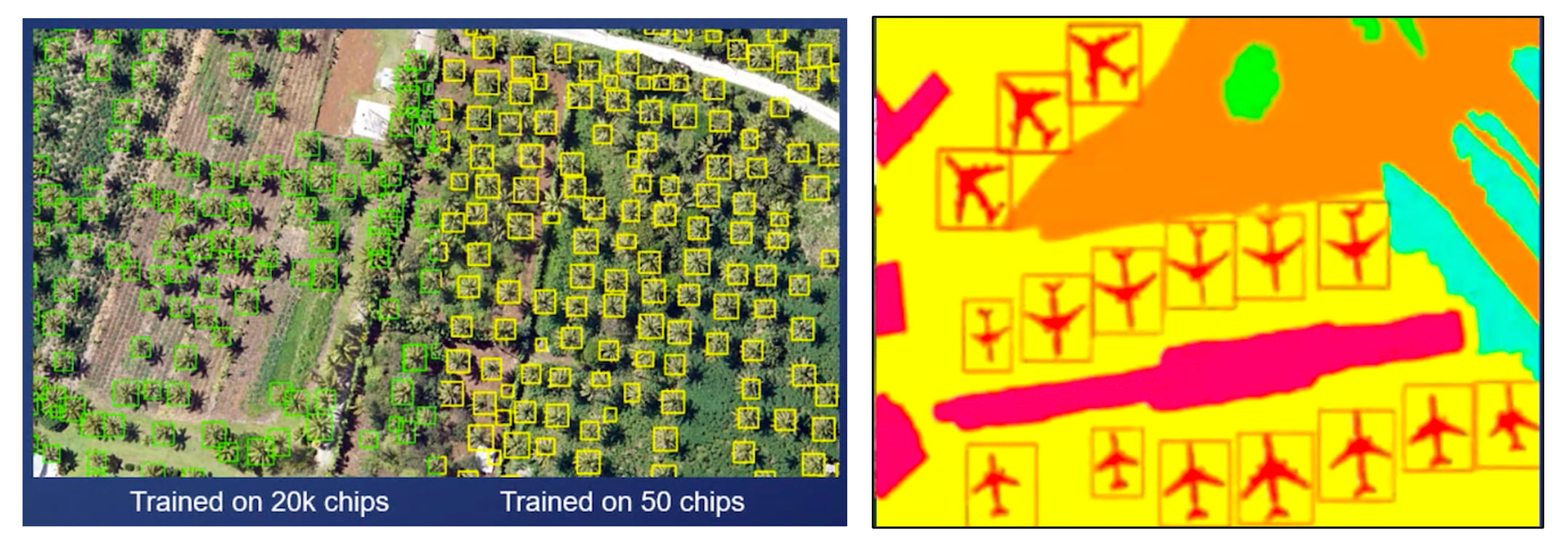
Motion imagery
The new Auto Detector tool within the Object Tracking display pane, which enables automatic detection and identification of target objects using a deep learning based detector model, has been introduced in this update. Please read the deep learning section above for more details.
Change Detection
When performing image-to-image change detection, users were limited to comparing single-band images or thematic rasters. In this release, we are introducing change detection across multispectral images. These new methods will compute change between the full spectra for each pixel. This allows greater flexibility, removes the need for prerequisite knowledge in choosing specific bands, and offers additional metrics for assessing pixel change or divergence. In the example below, the Spectral Angle Difference is computed between two Landsat 8 surface reflectance products to map changes in agricultural areas and lake levels around the Salton Sea, California.
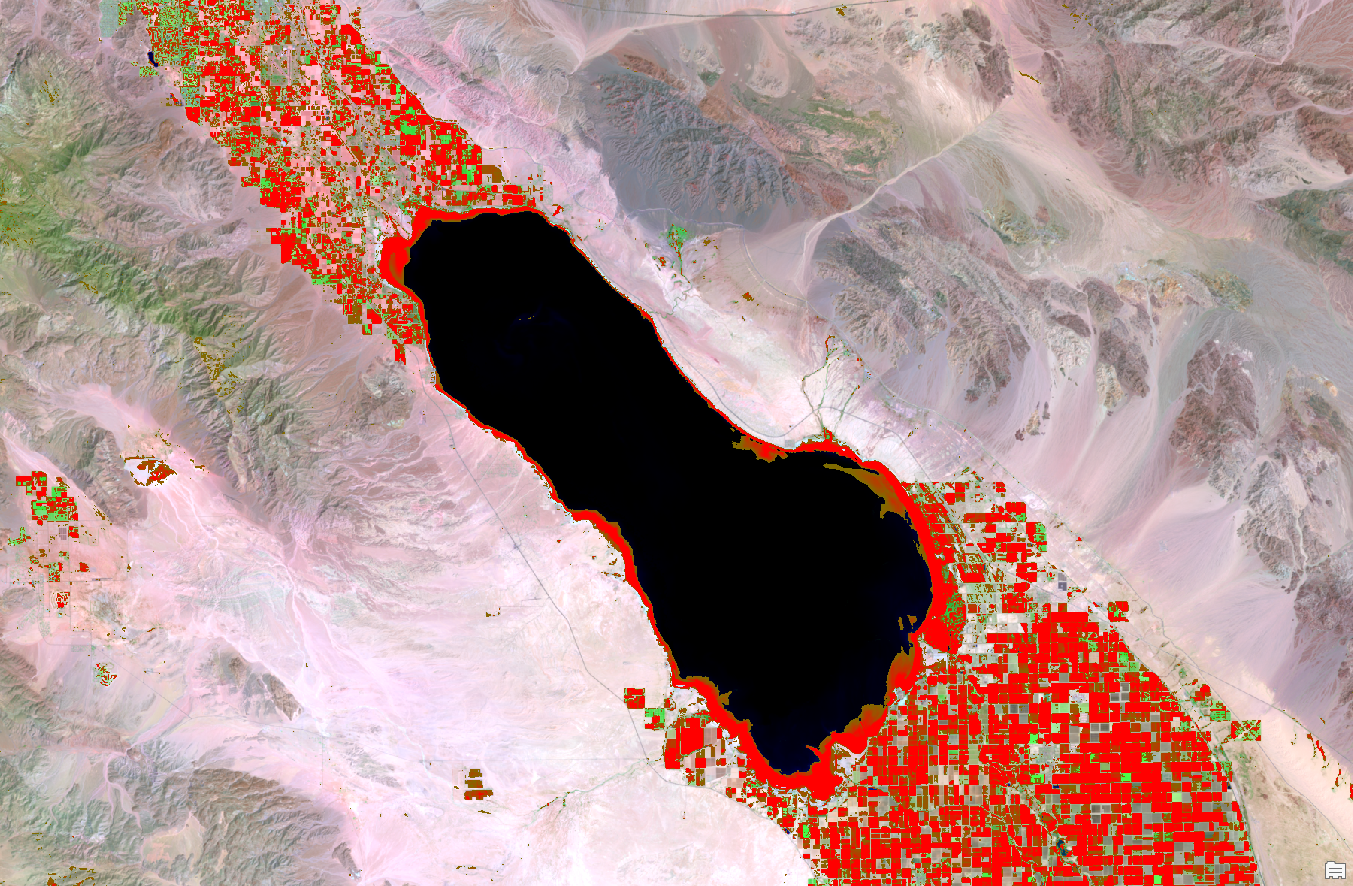
Multidimensional Analysis
Existing multidimensional analysis tools and raster functions have been enhanced to support more workflows. The Principal Component Analysis (PCA) tool for multidimensional data was introduced in the last update to look at spatial and temporal patterns using a multidimensional dataset. This means that instead of just looking at dominant spectral patterns in a multispectral imagery, users can run PCA on time series data. The tools are now augmented to perform spatial reduction to identify spatial and temporal patterns.
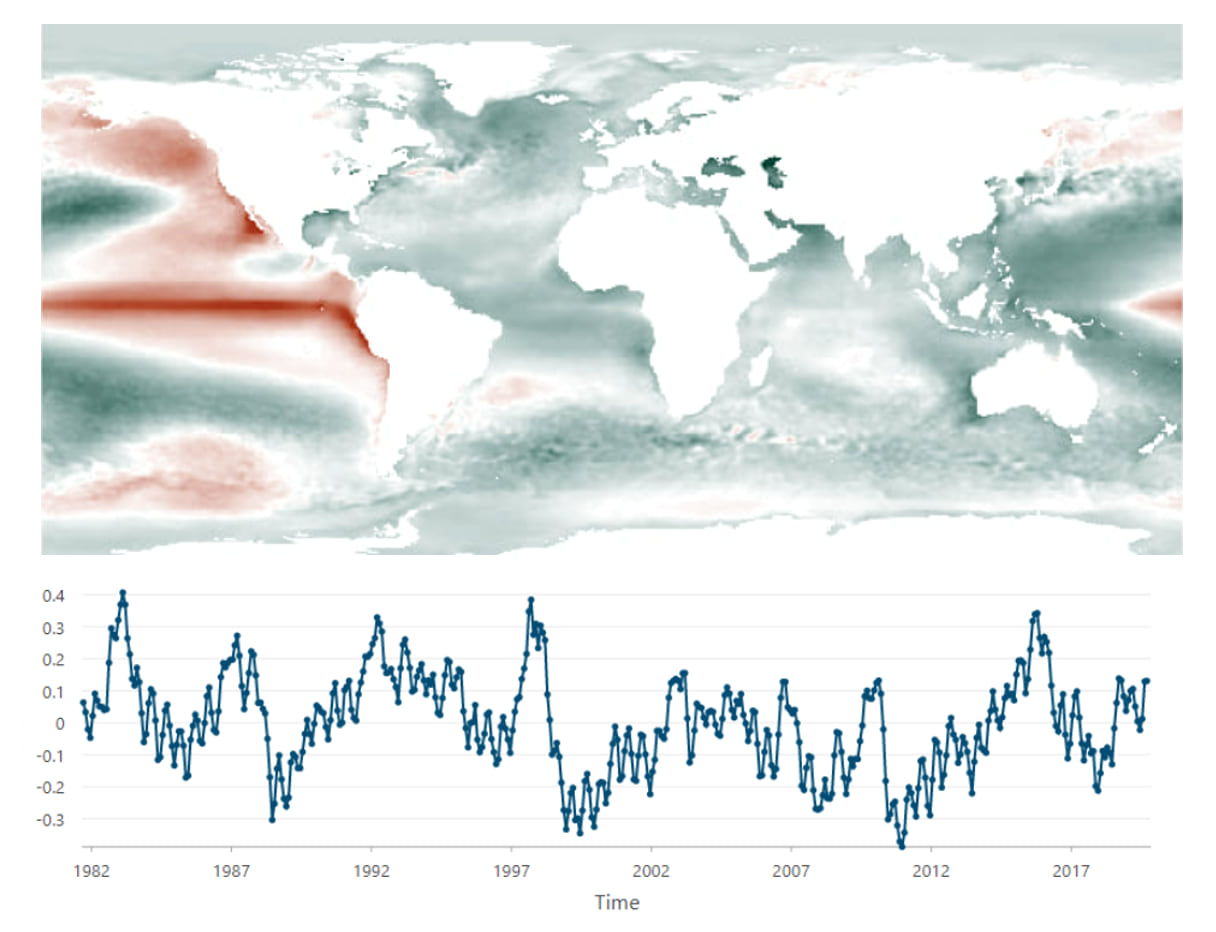
Stereo mapping
This announcement also highlights improvements to stereo mapping. In this release, the user experience analyzing and extracting 3D terrain features has been enhanced with Terrain tracking using a Digital Elevation Model (DEM). This enhances efficiency by enabling users to focus more on stereoscopic analysis and less on cursor height adjustments when in terrain following mode.
The final update to stereo mapping is expanded support for additional sensor models. Previously, only imagery defined by RPC sensor model was supported. Now, users can also work stereoscopically with Maxar satellite images defined by a physical sensor model. Support for other satellite data defined by the physical model will be rolled out in subsequent releases.
Ready to give these new capabilities a try?
Check out these resources to get you started:
Article Discussion: