The 2020 Census will incorporate a new way to protect your identity. This technique, called differential privacy, will “inject noise” into released data to lessen the chance of individual identification. Are you aware of the coming changes? Have you thought about how differential privacy might impact your work?
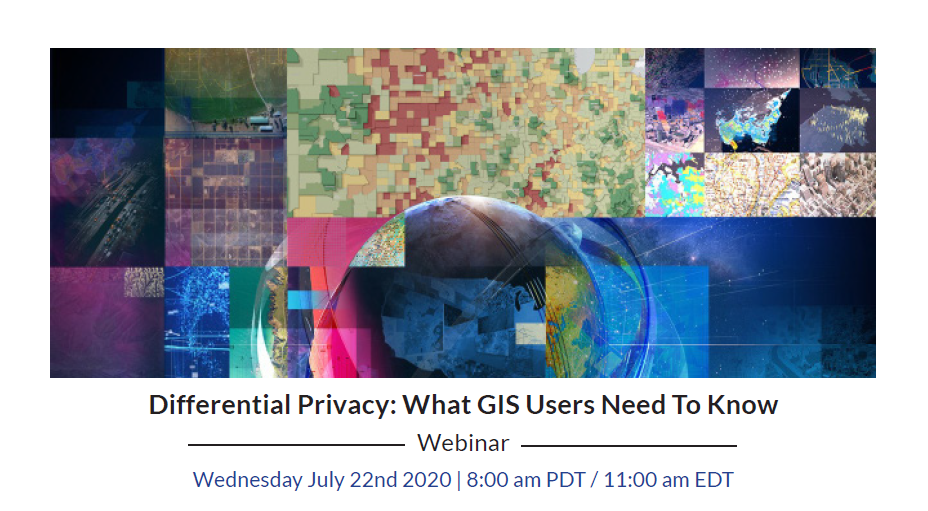
Join Esri, July 22nd, for a special webinar: Differential Privacy: What GIS Users Need To Know
with Dr. John M. Abowd, Associate Director for Research and Methodology and Chief Scientist at the US Census Bureau. Dr. Abowd will share insights into the US Census Bureau’s evolving approach to differential privacy. Joining Dr. Abowd will be Dr. Lauren Scott Griffin, Sr. Software Engineer and Spatial Statistician at Esri to discuss questions critical to the GIS community. This one-hour discussion will help GIS users prepare for and anticipate the marked changes in how census data are released. This webinar will cover some of the main points on what GIS users need to know to successfully work with Census 2020 data. Register now and submit your own question about differential privacy for Dr. Abowd to address.
WATCH THE WEBINAR RECORDING HERE
Accuracy vs. Individual Protection
The Census Bureau operates with a constitutional mandate to enumerate the US population every ten years. The Census Bureau is also required to keep the information they collect confidential for 72 years, and under Title 13, they must protect any individual from being identifiable in published data. These mandates are complex on their own and when viewed together are at odds with one another. This dichotomy between publishing accurate data and protecting an individual’s identity is a perpetual challenge for the Census Bureau. In past censuses the Census Bureau has used various forms of disclosure avoidance to ensure that privacy is protected while also releasing high quality data that fits the needs for numerous use cases. These techniques historically consisted of table suppression and data swapping. Census 2020 brings a new era of disclosure avoidance with the implementation of differential privacy. According to the Census Bureau’s Chief Scientist, Dr. John Abowd, the shift to differential privacy “marks a sea change for the way that official statistics are produced and published.”
Why Move to Differential Privacy?
Increases in computing power, innovations in data science, and the general increase in the amount of data collected has raised the fear of a “reconstruction attack” that utilizes census data. A reconstruction attack can combine publicly available data with private data to uncover precise information for individuals. The Census Bureau performed an internal experiment that attempted to reconstruct the 2010 Census data using commercially available databases. The bureau was able to correctly identify the name, census block, sex, age, race, and ethnicity of 52 million individuals. This unsettling finding, along with other successful reconstruction attacks, was the catalyst for the Census Bureau to implement differential privacy to protect Census 2020 data.
What is Differential Privacy?
Differential privacy is a “formal privacy” approach that provides proven mathematical privacy assurances by adding uncertainty or “noise” to the released data. This technique determines the amount of noise necessary to balance privacy loss and accuracy via mathematical formulas. With differential privacy the “acceptable risk” can be quantified through a measure called Epsilon. When Epsilon is set to zero, the data are completely scrambled. When Epsilon is set to infinity, the data are perfectly accurate. The bureau will establish Epsilon for every table released. This approach is set up to provide protection today as well as being “future-proof” in that it can protect against reconstruction attacks with any conceivable databases that may be available in the future.
This video Protecting Privacy with MATH, produced by minutephysics in collaboration with the Census Bureau, provides an excellent overview of differential privacy and reconstruction attacks.
What Will This New Data Look Like?
A successful implementation of differential privacy should look very similar to prior decennial census releases. However, while differential privacy is used to privatize the data, that is not the end of the story. The differentially privatized data are then subjected to post-processing changes. Post-processing involves the tweaks and adjustments necessary to account for negative numbers and non-integers created through the application of differential privacy. Post-processing ensures that smaller geographies like tracts sum to larger geographies like counties, but it also introduces its own error and bias into the counts. Together, differential privacy and the post-processing alterations are referred to as the 2020 Disclosure Avoidance System (DAS).
The Census Bureau has released 2010 Demonstration Data Products that provide the public with a sneak peek at what the 2010 raw data would look like after being pushed through the 2020 DAS. Data users and academics were eager to see the DAS in action, and the National Academy of Science Committee on National Statistics (CNSTAT) held a two-day workshop to explore the implications of the new DAS.
You can explore place-level changes between the original Census 2010 Summary File 1 (Sf1) data and the differentially privatized data in this application:
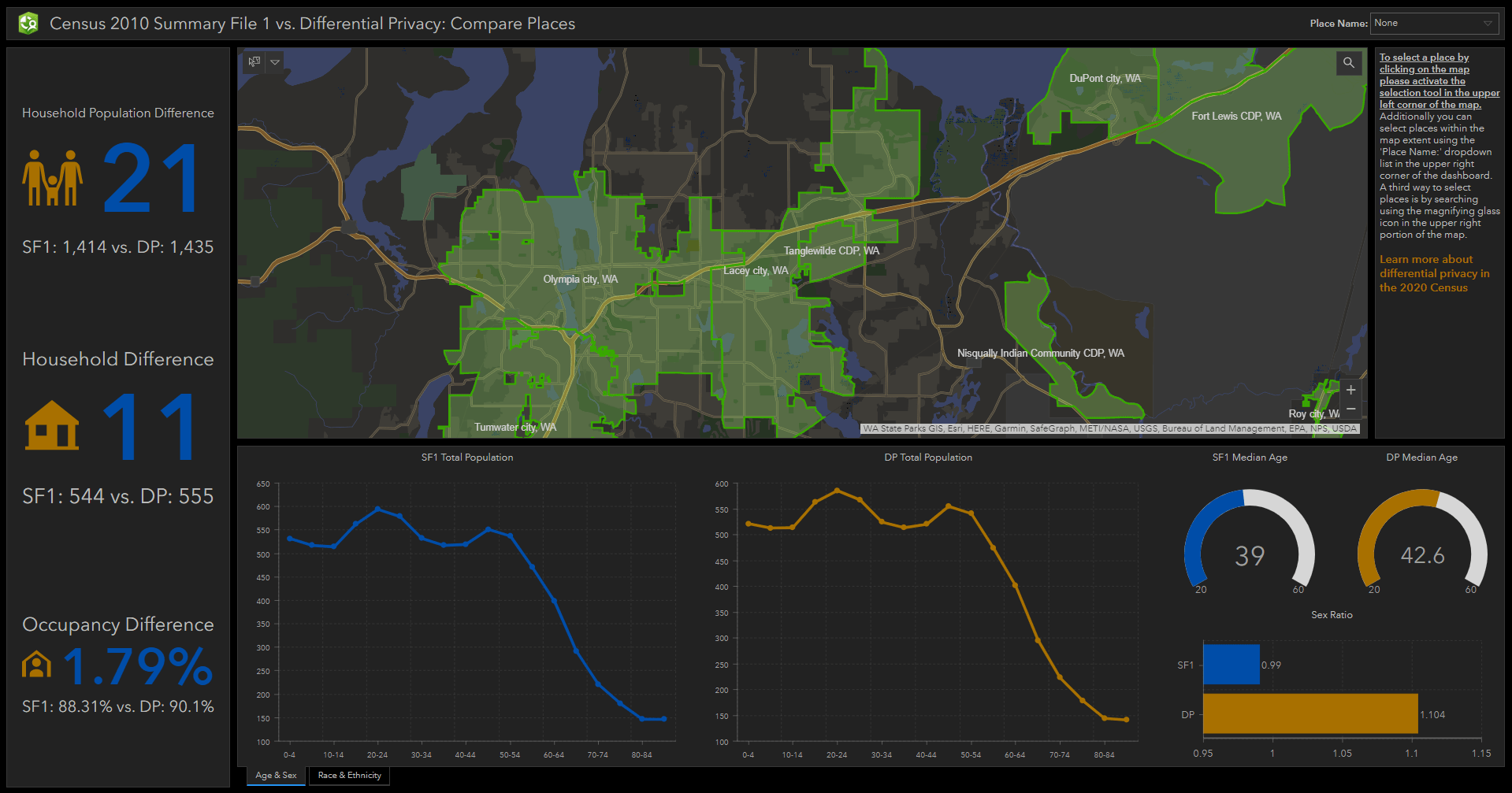
After extensive critiques the Census Bureau acknowledged many of the shortcomings of the original DAS and are working diligently to improve it. The bureau has committed to releasing information about each iteration of DAS and its application to 2010 Census data.
A Transparent Debate
The Census Bureau continues to innovate and adjust to a changing world. Last decade, the sheer expense of surveying one in six households put an end to the decennial census “long form.” With the advent of the American Community Survey (ACS), data users initially struggled to understand data reported as a five-year average along with Margins of Error (MOEs). With the release of ACS, the Census Bureau shifted to provide more transparency in the error associated with survey data. Data users now have access to error measures paired with every estimate in an ACS table. The move to implement differential privacy for the decennial census has many parallels with the early days of ACS. Decennial census data have always included error from prior disclosure avoidance techniques, imputation, and data collection challenges, but no metrics were released to inform users of the magnitude of this error. Differential privacy is shining a bright light on the error present in decennial census data and igniting a healthy debate over the accuracy vs. privacy conundrum. Many questions remain surrounding the usability of differentially privatized census data, including:
- Temporal Trends – How will longitudinal analysis from 2010 to 2020 be affected for small areas like block groups and school districts?
- Redistricting – Population counts will be subjected to noise. Will advocates of political equality challenge synthetic data?
- Small Area Analysis – Will small area analysis need to incorporate new tools to work with differentially privatized data? Will analysts have any metrics similar to margins of error to understand the magnitude of noise in the data?
- Marginalized Populations – Small geographies and small populations such as immigrants, American Indian Areas, and specific racial groups will be less accurate than data for larger groups and geographies. What are the implications for social justice and social equity? Is it possible to accurately count these populations while still maintaining individual anonymity?
- Spatial Distribution of Noise – The initial 2010 Demonstration Products showed that spatial structure was being added to the data. Will the DAS be able to avoid introducing spatial bias?
- Data Inconsistencies – The initial 2010 Demonstration Products included inconsistencies such as occupancy rates greater than 100%, and households with children and no adults. Will the DAS be able to reconcile household and population universes to correct these inconsistencies?
Esri is committed to help our users understand and leverage Census 2020 data. We will be partnering with the Census Bureau to provide best practices for working with differentially privatized census data.
2020 Esri User Conference
Please join us at the 2020 Virtual UC on Tuesday July 14th at 7:30 am – 8:30 PDT for a one hour discussion of what’s new in Esri demographics and the changes coming to Census 2020: Esri Demographics and Census 2020: What’s New in the U.S.
Also check out these story maps for other presentations on Esri data and content and modernizing official statistics with GIS!
Article Discussion: