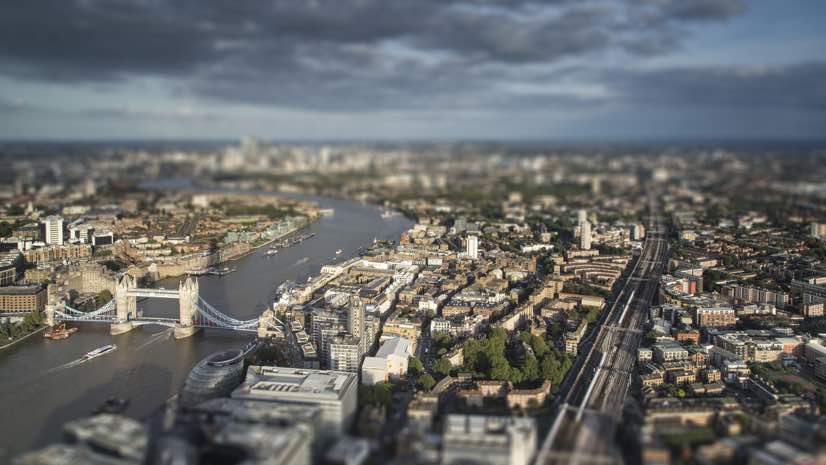
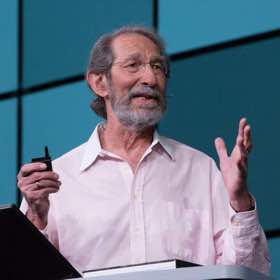
The more data, the better, but mindlessly just collecting and processing it—even spatially, with algorithmic methods only—is potentially dangerous. It will lead to quite erroneous conclusions. It doesn't typically lead to understanding.
September 21, 2017
Physicist Geoffrey West highlights the power of geography
The age of big data brings a shift in problem solving that relies on algorithms, cloud computing, and data analysis as a means to test assumptions and arrive at answers. While no scientist would say that more data is a bad thing, theoretical physicist Geoffrey West cautions that underlying principles are vital.
West has spent considerable time investigating the laws of science beyond his initial inquiries in physics. After long stints at Stanford University and Los Alamos Labs, he joined the Santa Fe Institute (SFI), which is famous for its focus on understanding complex systems. West is a past president of SFI, and this is where he began to develop large-scale statistical inquiries of biology that soon expanded to encompass a predictable mathematical means to understand cities and even companies.
West recently published a book, Scale: The Universal Laws of Growth, Innovation, Sustainability, and the Pace of Life in Organisms, Cities, Economies, and Companies. He was the keynote speaker at the 2017 Esri User Conference (Esri UC), where he was a huge hit with the audience. He acknowledges the unique role of geographic information system (GIS) technology to provide visual verification for big data inquiries, and GIS users are excited about his pursuit of a science of cities. We spoke to him about models and theories and the benefits of big data.
This interview has been edited and condensed, and there are a few places where West’s remarks have been supplemented with additional helpful material from the book Scale.
The more data, the better, but mindlessly just collecting and processing it—even spatially, with algorithmic methods only—is potentially dangerous. It will lead to quite erroneous conclusions. It doesn't typically lead to understanding.
The cancellation of the Superconducting Super Collider (SSC) particle accelerator in 1993 pushed you down a different path. This machine would have generated a great deal of data and answers, right?
West: The Large Hadron Collider at CERN [Conseil Européen pour la Recherche Nucléaire, or European Council for Nuclear Research] produces roughly 100 times more data than you can process if you used all of the computational capacity across the planet. The SSC would have produced 10 or 100 times more than that.
One of the interesting offshoots of the whole field of high-energy physics has been how to deal with big data. Many people don’t realize that the World Wide Web came out of addressing that question. It was one of the most marvelous unintended consequences of building such a machine. The search for the golden nuggets in this mountain of data is one of the mostly unheralded achievements of that whole experimental field.
The pace of data creation has increased dramatically since then, thanks largely to the Internet and the emerging Internet of Things. How do you feel about the growing volumes of data?
West: The more data, the better, but mindlessly just collecting and processing it—even spatially, with algorithmic methods only—is potentially dangerous. It will lead to quite erroneous conclusions. It doesn’t typically lead to understanding.
Developing models and theories and trying to expose underlying principles are the ways science has progressed. With this onslaught of big data, these frameworks become more important.
You start to believe everything the computer churns out is reality, but you should be highly cautious, as there are many problems. One needs to develop even more vigorously the underlying concepts and ideas. Without those ideas, we don’t know what kind of data we need, how much, or what critical questions we should be asking.
This is one of the battles that I fight. Computer scientists and others say you don’t need underlying principles anymore because the data speaks for itself. I think that’s a dangerous position to take.
[Editor’s note: West has tracked this trend in computer science and academia, and in his book Scale, he expresses how he’s particularly bothered by an article by Chris Anderson that appeared in Wired magazine in 2008, titled “The End of Theory: The Data Deluge Makes the Scientific Method Obsolete.”]
One of the unique things about geographic information science (GIScience) is that visualization relates the data to points on the ground, so there’s a visual verification.
West: Exactly, you have that. The problem is that in so many other applications, you don’t have an analog to that. The only way you discover anything interesting is that you have a theoretical framework and models that tell you that most of this big data isn’t interesting. Don’t get blinded by it.
For example, the Large Hadron Collider has 150 million sensors, with 600 million collision events recorded every second. That’s a phenomenal amount of data—150 million petabytes per year if you captured it all, which is more data than the whole of [what] the Internet holds. The only way you get anything from that data is with a conceptual framework and a theory to narrow what you’re looking for. A framework has distilled all that data to a more manageable 200 petabytes per year.
Big data reveals patterns that allow prediction but don’t necessarily reveal the why behind the predictions?
West: That’s right. You need the why in order to extrapolate into the future. It’s that old question of causation versus causality. Just seeing some correlation doesn’t imply cause, of course.
When you have [an Isaac] Newton who comes along and says, “This is the reason why,” it revolutionizes everything. You get a deep understanding that reveals something in a new way. Newton’s findings go way beyond the motion of the planets—it’s the motion of everything around us. It’s extraordinary.
That’s the paradigm we need to explore. We need to remain with the observation data, looking for patterns; regularities; and the development of concepts, theories, and principles. I’m very concerned with the movement away from that and the idea that data will speak for itself.
[Editor’s Note: The following excerpt from West’s book Scale provides an intriguing example:]
Just because two sets of data are closely correlated does not imply that one is the cause of the other. For instance, over the 11-year period from 1999 to 2010, the variation in the total spending on science, space, and technology in the United States almost exactly followed the variation in the number of suicides by hanging, strangulation, and suffocation. It’s extremely unlikely that there is any causal connection between these two phenomena: the decrease in spending in science was surely not the cause of the decrease in how many people hanged themselves. However, in many situations, such a clear-cut conclusion is not so clear. More generally, correlation is in fact often an important indication of a causal connection, but usually it can only be established after further investigation and the development of a mechanistic model.
What’s the danger?
West: The danger lies mostly on the socioeconomic sphere, where we use data to make not just predictions but major policy decisions. For short time scales, those extrapolations are probably fine. It’s a question for long-term strategy where these questions become suspect. Long-term strategy involves nonlinear processes and a lot of feedback. You really need to understand mechanism and not just pattern.
[Editor’s Note: West relates an example in Scale about economic forecasting:]
It is sobering that no detailed model for how the economy actually works exists and that policy is typically determined by relatively localized, sometimes intuitive, ideas of how it should work. Very little of the thinking explicitly acknowledges that the economy is an ever-evolving complex adaptive system and that deconstructing its multitudinous interdependent components into finer and finer semiautonomous subsystems can lead to misleading, even dangerous, conclusions as testified by the history of economic forecasting. Like long-term weather forecasting, this is a notoriously difficult challenge, and to be fair to economists, we should recognize that they are pretty good at forecasting relatively short term, provided the economy remains stable. The serious challenge is to be able to predict outlying events, major transitions, critical points, and devastating economic hurricanes and tornadoes where their record has mostly been pretty dismal.
In Scale, you write, “Analyzing massive datasets of cell phone calls can potentially provide us with new and testable quantitative insight into the structure and dynamics of social networks and the spatial relationships between place and people and, by extension, into the structure and dynamics of cities.”
Do you feel geography has a role in improving our understanding of cities?
West: The way geography has redefined itself, or is redefining itself, it has the potential of integrating city information for improved understanding. This reinterpretation of geography is really important. I don’t think it has the big picture vision yet. I’m being egotistical here because of the reaction that I got from my talk. Those people, to my great amazement, saw this book as a way to help them see a bigger picture.
To learn more about the mechanisms of scale that apply to biology, cities, and companies, watch West’s 2017 Esri UC Keynote Address in its entirety in this video presentation. A profile of West and his work appears in ArcWatch. West’s book, Scale, can be found at bookstores now.
August 30, 2017 |
August 16, 2017 |
September 5, 2017 |