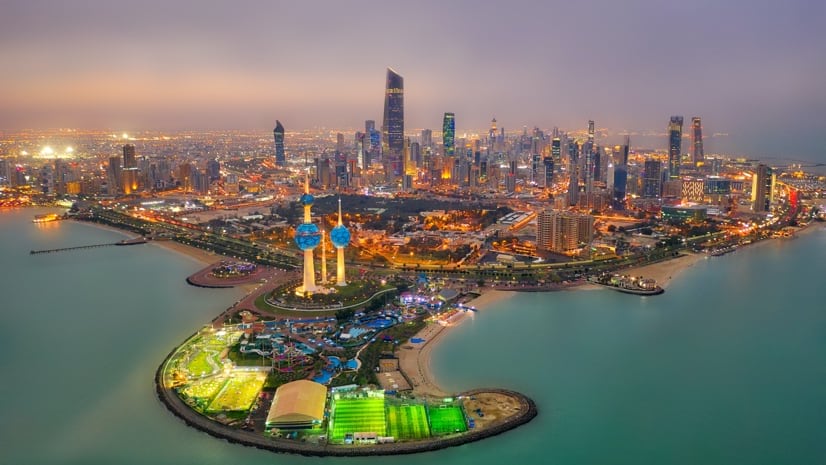
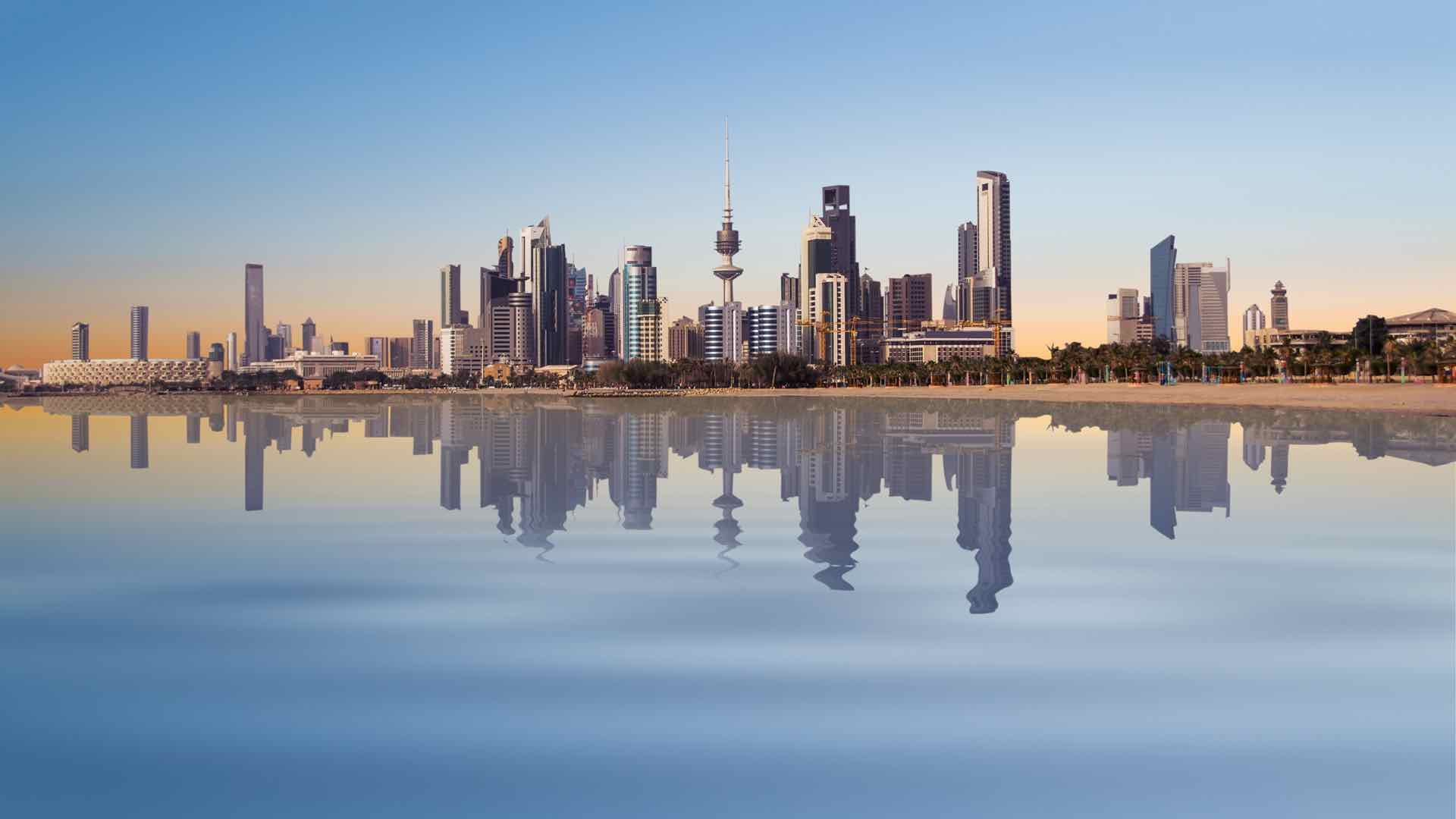
January 24, 2019 |
May 12, 2020
Kuwait Finder, a mobile location app built by the Kuwaiti government, was a smashing success when it was first released in 2013. Among its many achievements, it was a triumph of data-gathering and data-processing, offering authoritative turn-by-turn directions for Kuwait, a locale whose system of address numbers and street names can be confusing.
By the end of last year, Kuwait Finder had amassed 750,000 users. Within the country, that level of saturation makes Kuwait Finder a more popular wayfinding tool than Google Maps.
This popularity presented its own challenges including how to keep the application and underlying data up to date. Per capita, Kuwait is the fourth-wealthiest country in the world, and with the Kuwait National Development Plan goal to increase infrastructure expenditures by 11 percent, change is already well underway. Construction projects—including the world’s longest causeway, a new airport passenger terminal, and a new 500,000-person residential area called Silk City—amounts to $500 billion in active investments including major changes to transportation networks and other infrastructure.
To stay trusted, Kuwait Finder must capture and reflect this dynamism, with an up-to-the-moment authoritative geospatial rendition of the entire country.
To create Kuwait Finder initially back in 2012, a five-person staff at Kuwait’s Public Authority for Civil Information (PACI) tapped into the country’s long-term investment in geographic information system (GIS) technology. The GIS team pulled together data from various ministries, as well as PACI’s internal paper maps and AutoCAD files, to capture the current basemap. With the objective of updating the construction of new buildings and changes to the city’s streets, they studied satellite imagery to extract details for the map. But by the time the crew found the changes and input them back into the Kuwait Finder database, the infrastructure of Kuwait City had changed once again.
“Where are the new streets? Where are the building footprints?” Maher Abdel Karim, PACI’s GIS consultant said recently, summarizing the challenges. “Once we were finished updating them, there would be new satellite imagery we’d missed, and we’d have to repeat the process again.”
PACI needed to make necessary changes to Kuwait Finder in a way that was quick, inexpensive, accurate and simple—the more automated, the better. Compared to the vast resources available to a company like Google, PACI’s size and budget were limited.
PACI researched the viability of artificial intelligence to provide a solution.
“We thought, how can we use AI to automate the process?” Abdel Karim said. “We wanted to use machine learning to extract street data and building footprints from the satellite imagery while using the minimum amount of human input.”
Deep learning, a powerful form of AI, involves teaching a computer to detect patterns in large amounts of data, and to recognize and extract just the information you want. If done right, the algorithm acts quickly and thoroughly and even finds changes that human intuition would miss.
PACI’s GIS team needed to teach the computer how to recognize building footprints from satellite data, and also note which ones were new since the last batch of satellite images. Elsewhere in the Middle East, an oil-and-gas company used machine learning to alert it if any new structures were being built near its thousands of miles of pipeline. PACI’s task was a bit more complex as it wanted the computer to tell it about anything new across the entire country.
If properly trained, a machine learning algorithm can move conceptual mountains. At the beginning of the process, however, the computer needs to be taught to “read.” This requires the same patience and skill required to teach a young child to recognize letters, then words and sentences, and finally complex thoughts.
PACI needed to establish a “ground truth,” a common geospatial framework that would encompass the existing database. After much trial-and-error, the staff was able to train using 75 square kilometers of data to provide input for the model to scan 3,000 square kilometers of satellite imagery.
For PACI, the main challenge was giving the program enough information to recognize buildings and streets.
“The contrast between land, streets, and buildings, was very minimal,” Abdel Karim explained. “It’s hard even for a person to differentiate among them by eye. And our threshold for saying that our model worked was very high.”
Often, with shadows cast by the sweltering summer sun, building footprints did not easily align with the images captured by the satellite. The darkened overlapping edges often confused the program.
Streets posed their own unique problem. They had to be recognizable individually, but also as part of a coherent grid. PACI’s staff had to ensure that all streets in the imagery were connected by common “center lines”—and that the lines in the new images fit seamlessly with those in the existing street network.
“You really have to take your local experience and use it as a ‘flavor’ on top of the satellite imagery,” Abdel Karim said. “That’s what makes it possible for machine learning to perform these calculations.”
The teaching process required serious work. But once the machine learning model was ready, the rest was a relative breeze. PACI devoted time to training and fine tuning the model, knowing that this work would pay dividends into the future.
The model and training data set can be used today to update the database in about three hours and provide even more accurate maps than before. And this model will live on and can continue to be modified and enhanced by PACI into the future, taking into consideration new architecture styles, building types or other new features.
The model could now do, before lunchtime, a task that previously took five humans a year to complete. The time spent to train the machine to extract features from satellite imagery to its GIS repository paid off.
“You need to have a lot of patience,” Abdel Karim said. “It can be a fairly long, iterative process to train, predict, evaluate, and then go in and do it all over again. It’s a knowledge investment. You’re investing in your staff, who are working, studying, and learning how to do this, to get the desired output.”
However, the return is worth the investment. The organization now has the confidence that it can keep the Kuwait base map and Kuwait Finder updated in a timely manner and have it continue to be the authoritative source for information in Kuwait. The time and cost savings from this automation will allow the small team at PACI to keep innovating to stay ahead of the competition. The lessons learned from using deep learning and remotely sensed data will also feed new ideas for PACI staff and will foster more innovation.
“We will continue to use machine learning to create new features and new layers for our GIS repository that people really need and are looking for,” Abdel Karim said.
Explore more examples of where artificial intelligence and GIS intersect.
January 24, 2019 |
November 26, 2019 |
January 28, 2019 |