The November 2021 release of ArcGIS Data Reviewer has new capabilities that automate and simplify the quality control of GIS data. This includes an expanded library of data validation checks to detect common errors in your data and enhanced workflows for managing errors detected during review. This unified set of capabilities to detect, manage, and report errors in your data enable you to lower data management costs while reducing risks in decision-making.
Data Reviewer provides a library of checks that automate the detection of common errors in GIS data. Automated checks assess different aspects of a feature’s quality that might include its attribution, spatial relationship with other features, or its integrity. Reviewer’s checks are simple to use since they are configurable and do not require specialized skills to implement beyond a good understanding of your quality requirements.
In the latest release, there are thirteen (13) new Reviewer checks that identify poor quality features in your data. A complete list of these checks can be found in the What’s new in ArcGIS Pro 2.9 topic.
Finding errors in attribution
The latest release includes a series of checks that enable you to assess the quality of a feature’s attribution in different ways. This includes identifying field values that are not unique (Unique Field Value check) and cardinality and relationship rule violations in data sources that participate in a Relationship class (Relationship check).
The Regular Expression check finds features or rows containing text values that do not match an expected pattern. For example, in U.S. roadway data management, road centerline features contain a globally unique identifier (GUID) attribute that is both unique in value and formatted based on a national standard. Values in this field include a locally assigned ID and a pre-defined agency identify code (example: unique_value@mycounty.mystate.us). Using the Regular Expression check, values which do not match this formatting pattern are returned as an error.
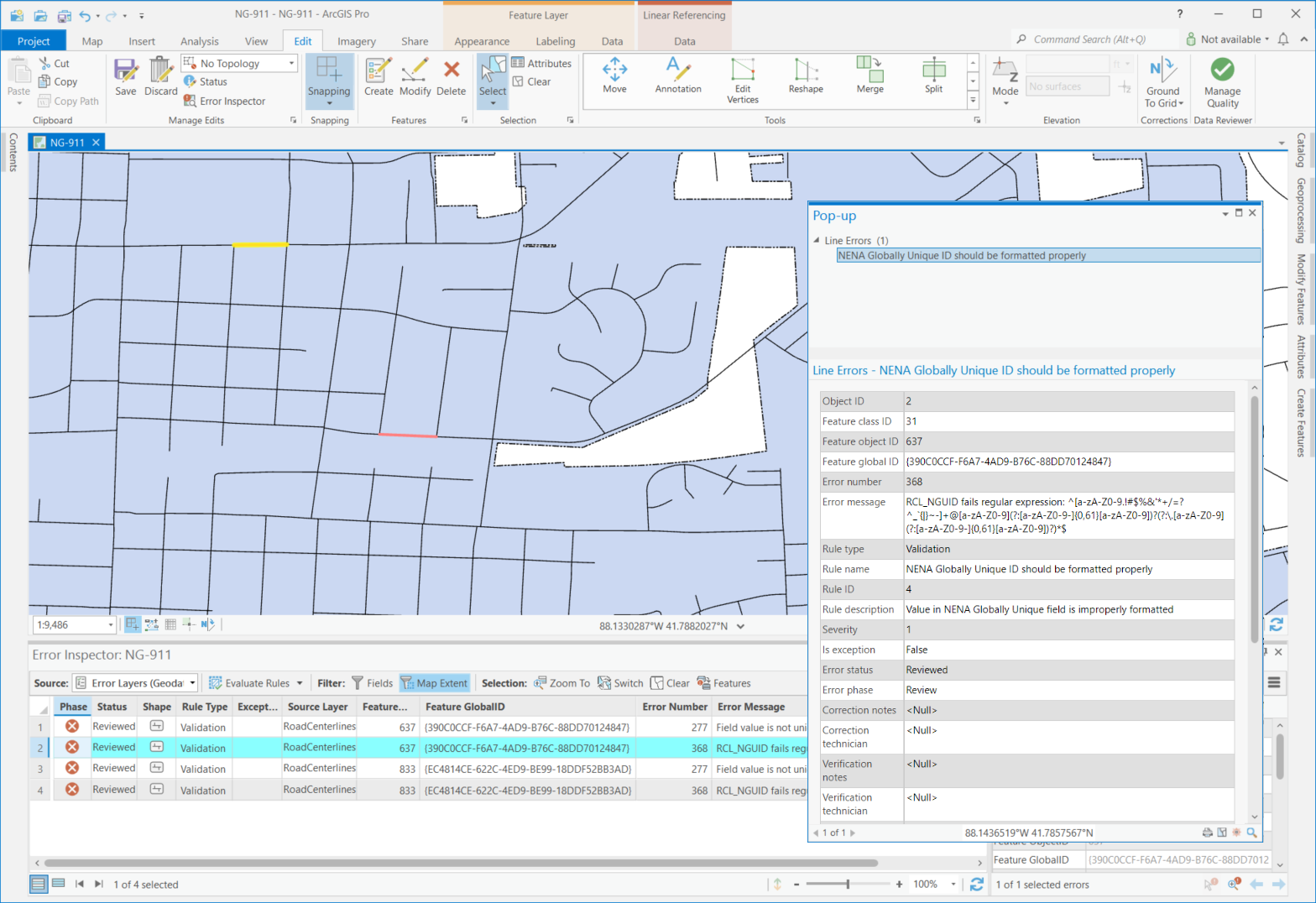
Finding errors in spatial relationships
Other new checks have been added to assess spatial relationships between multiple data sources. Frequently-used checks in this category include the Polygon Gap is Sliver and Composite checks. The first one helps to identify gaps between two or more polygon features based on the shape and size of the gap area. This can be particularly helpful when evaluating the quality of features created using non-Esri applications or those created without a proper snapping environment.
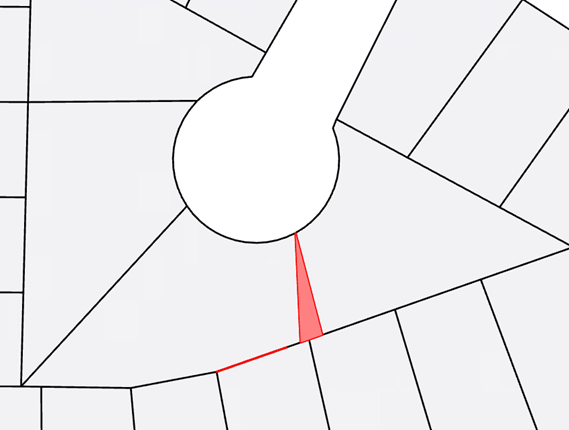
The Composite check finds features and rows that fail evaluation by two or more Reviewer checks. This can be particularly useful when error results from a single check do not completely meet a quality requirement. An example of this applies to mapping topographic features. When mapping a tunnel opening point it must be coincident with either the end of a road, railway, aqueduct, or canal feature. By combining the results from four (4) Feature on Feature checks, those tunnel openings which are not touching any of the above features are returned as an error.
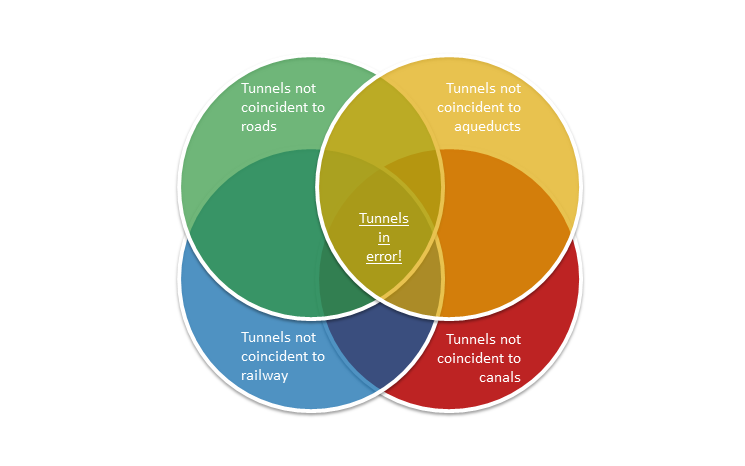
Finding errors in a feature’s integrity
Other new checks in this release include those that assess different aspects of a feature’s integrity. These include checks that assess Z-values to identify those which exceed a threshold when compared to other values on the same feature (Adjacent Vertex Elevation Change check) or contain values outside a defined range (Evaluate Z Values check).
Additional checks in this category are used to assess the quality of polygon features. One of them is the Polygon Sliver check to find polygon features that are considered a sliver based on its shape and (optionally) area. This check is particularly useful when assessing the quality of features created from conversion of hard copy documents or generated when data of different scales or extraction requirements are combined.
In this video, see how Data Reviewer’s configurable checks are used to implement data quality requirements.
Error Management
Errors detected during data validation provide insight into the sources of poor-quality data and, when coupled with a quality assurance plan, the risk they pose to activities which rely on high-quality data to meet an organization’s objectives. Leveraging error results in data editing workflows speeds the correction process by identifying the source and location of features that do not meet your quality requirements.
In this release, many Data Reviewer checks that evaluate a feature’s quality in the context of other features in the same or different data sources have been enhanced to improve error detection and reporting. This enhancement expands the features that are considered during data validation and results in improved reporting of error corrections when related features are edited and re-evaluated. Checks impacted by this change include those that assess the quality of a feature based on its spatial relationship to another feature (such as the Feature on Feature check) and those that assess a combination of spatial and attribute relationships between features (such as the Duplicate Feature check). Other checks enhanced in this release include those that evaluate attribute relationships between features (such as the Table to Table Attribute check).
In this video, see how Data Reviewer’s automated checks are used to identify features in your database that do not comply with data quality requirements.
Check out the resources below to learn more about Data Reviewer.
- Learn more about ArcGIS Data Reviewer
- Watch the introduction to ArcGIS Data Reviewer video
- Get started with ArcGIS Data Reviewer
- Connect with the ArcGIS Data Reviewer community
Article Discussion: