Introduction
To protect respondents’ privacy the federal government has injected noise into nearly every statistic reported in the 2020 decennial census. As a data user you have a few options. You can ignore the noise and proceed in using census data as you always have. Or, if you’re concerned, you can ask the logical question, “How much noise?”
Until now it has been exceedingly difficult to know the answer to that question. Esri has developed a methodology to approximate the amount of noise infused into the Census 2020 data. The output includes counts from Census 2020 alongside estimates of noise and a range of possible values from Esri:
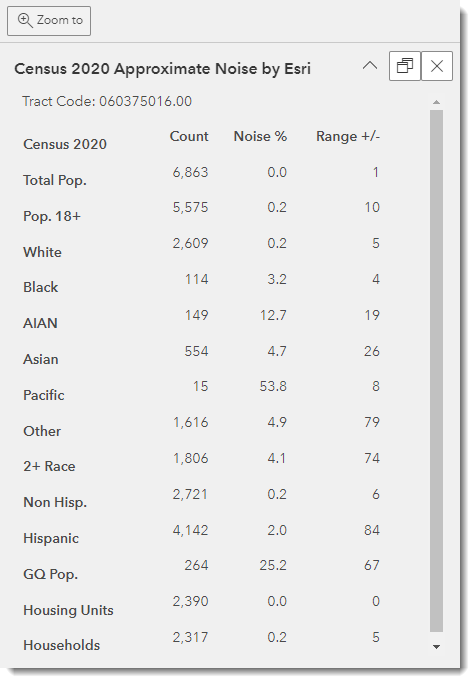
This technique estimates noise (variation from the actual counts) using materials developed by the U.S. Census Bureau to evaluate and fine-tune the 2020 privacy protection algorithms. If you want to better understand the magnitude of noise in Census 2020 data and how it impacts your use cases, there is a solution for you: Esri Extended Error Metrics.
New Era of Privacy Protection
The 2020 Census brought privacy protection issues out of the shadows and into the limelight. The U.S. Census Bureau has always been bound to protect individuals’ privacy in published data under Title 13. In prior censuses this was accomplished via a combination of data swapping and data suppression techniques. No one understood the extent that census data were altered to protect individuals’ privacy because it was never published. This all changed with the introduction of the Census 2020 Disclosure Avoidance System (DAS).
The Census 2020 DAS sparked a healthy debate over privacy protection methods. This decade, disclosure avoidance methods have been published and the Census Bureau has actively worked with stakeholders to refine their methods. The 2020 DAS has two primary components. First, the data are subjected to Differential Privacy (DP). This is a formal statistical approach used to inject “noise” from a symmetric distribution to each statistic. Second, postprocessing is used to adjust the noisy data so that it looks like census data that we are accustomed to using. These adjustments ensure that fractions or negative values are removed, components sum to their respective table totals, and impossible or improbable statistics are kept to a minimum.
How Does Noise Impact Census Data?
The Census Bureau has released multiple measures, metrics, and data products to help experts evaluate the tradeoff between noisy data and the threat of reidentification. A small but active community of demographers, statisticians, data scientists, and policy experts who understand the intricacies of the DAS has been highly engaged with the Census Bureau in evaluating impacts on data quality. But what about typical census data users who don’t have time to evaluate Rho, Epsilon, iterative adjustments to the top-down algorithm, or analyze Demonstration Data and Progress Metrics? Typical data users just want to understand, “How does noise impact census data?”
Esri Provides a Simple Answer
Data quality is important to every census data user. All data products have error and bias, however until now it has been very difficult to understand the impact of the new DAS on census statistics at the variable level. Esri’s approach is one that many data users are familiar with. Many data users have spent the last decade becoming comfortable with American Community Survey (ACS) data and Margins of Error (MOEs). If you understand MOEs then you will understand how Esri represents approximations of noise in the Census 2020 redistricting data. These maps provide estimates of percent noise and a range of values (+/-) that the true count is likely to be within for select variables from the Census 2020 PL 94-171 redistricting data. Currently these estimates are available exclusively through the linked application, web map and hosted feature layer for all counties, tracts, and block groups.
Example
-
-
-
County: Riverside, California (06065)
-
Census 2020 Asian Population: 171,243
-
Approximate Noise: 0.5%
-
Range: +/- 788
-
-
These metrics tell the data user that the Census likely injected around 0.5% noise for this variable to protect privacy. In this county the noise amounted to the addition or subtraction of around 788 persons. Knowing this, we can assume that the actual count of the Asian population was likely between 170,455 and 172,031 in Riverside County for Census 2020.
Map Application
Click the map to explore Esri Extended Error Metrics:
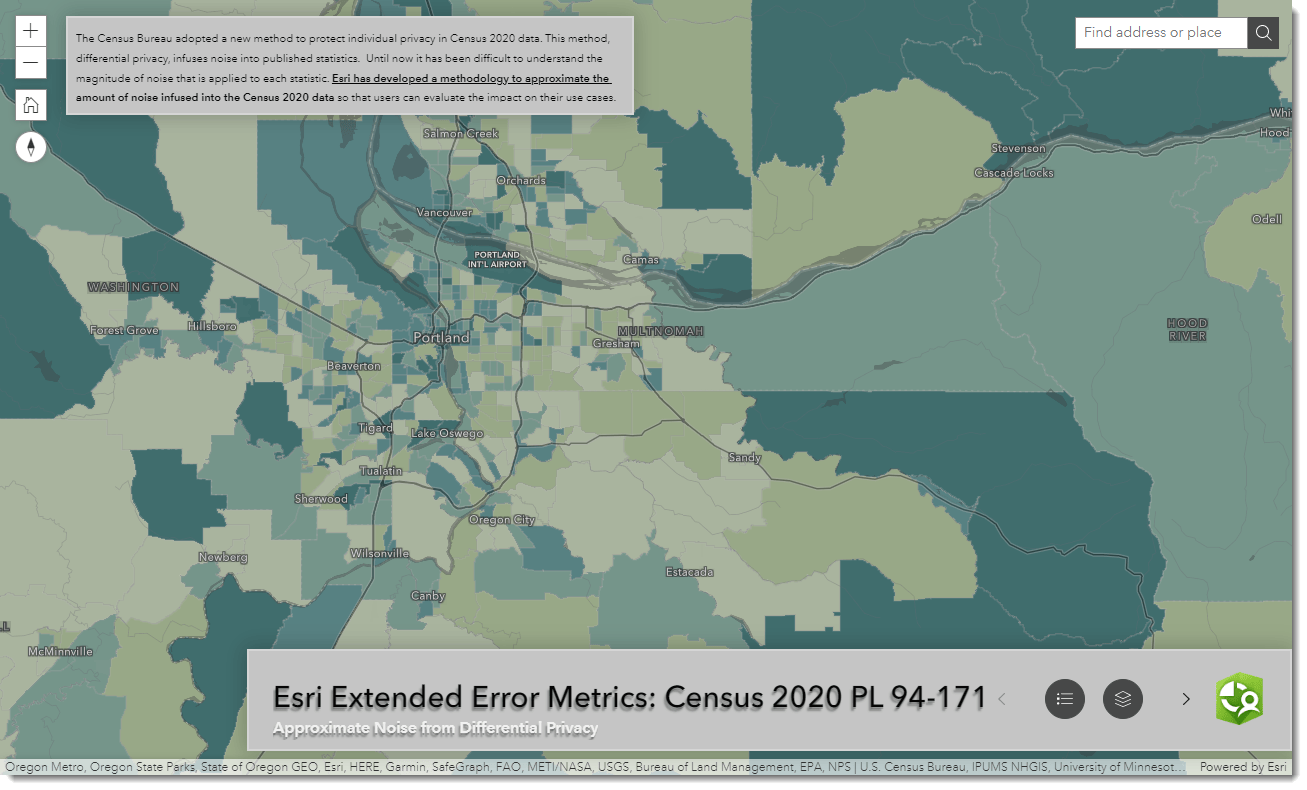
If the application does not fit your needs you can access a web map or hosted feature layer from Living Atlas of the World.
Call To Action
Esri strives to provide our users with best practices for working with the next generation of Census data. By extending and refining metrics of error or differences between the Census 2010 data and the differentially privatized version of the Census 2010 data we can approximate noise in the Census 2020 data for the same variables. Esri Extended Error Metrics provide a much-needed pragmatic understanding of the magnitude of noise that has been injected into 2020 statistics that is critical for making decisions based on the Census 2020 redistricting data.
We encourage you to explore the Esri Extended Error Metrics application, web map and hosted feature layer and let us know your feedback. Use the comments section below or email datasales@esri.com to let us know if you would like to see Esri Extended Error Metrics applied to all Census 2020 data accessible through Esri Demographics. Thank you for your interest and feedback.
How Do We Do It?
To help users understand the impacts of the DAS on the Census 2020 P.L. 94-171 redistricting data file, the Census Bureau released DAS Demonstration Data. The Demonstration Data consist of Census 2010 data run through the DAS that was used for Census 2020. This allows users to analyze differences between the differentially privatized Census 2010 data and the Census 2010 data as it was released[1]. These differences are the result of noise injection and post processing. Evaluating the differences for each variable provides insight on how the DAS will alter Census 2020 data.
In addition, the Census Bureau released Progress Metrics. These metrics are produced from the comparison between differentially privatized Census 2010 data and the Census 2010 data as it was released and provide measurements of accuracy, bias, and outliers. It is implied that when the 2020 DAS is applied to Census 2020 data, the same or a very similar amount of accuracy, bias, and outliers are to be expected. This information, although built from 2010 data, can tell us a great deal about how Census 2020 data will look after noise injection and postprocessing.
Esri Extended Error Metrics go beyond the Census Progress Metrics to include measurements of accuracy for all census variables, at more granular geographies including tract and block group-levels. Statistics are further stratified by total population quintile to achieve a more accurate approximation of noise for each variable, for each population size, at each level of geography. Population size and geography are important constraints for privacy protection. The larger the population size or characteristic the less the chance of re-identification. The smaller the population size or characteristic, the more noise is necessary for privacy protection.
Approximations of noise are built from the metric: Mean Absolute Percent Error (MAPE). This is a measure of the average absolute percent difference between released Census 2010 and differentially privatized Census 2010 values. MAPE is likely the most popular error measure because it provides an easy to interpret summarization of differences across percentage distributions. MAPE is an excellent approximation of injected noise irrespective of postprocessing. Approximations of range are built from multiplying the reported Census value with the approximation of noise. This provides a range of possible values that the non-noisy, true count is likely to be within.
Esri leveraged Privacy-Protected Summary Files (PPSFs) from IPUMS[2] for the production-level settings of the 2020 redistricting DAS to create Esri Extended Error Metrics. The PPSFs are tabular versions of the Privacy-Protected Microdata Files (PPMFs) produced by the Census Bureau.
This approach assumes that data swapping in the demonstration data is insignificant since it cannot be controlled for. Because only the Census Bureau has access to the un-swapped data, comparisons between the unaltered 2010 counts and the 2010 differentially privatized data are not possible. This assumption is likely to slightly overestimate the actual noise in the counts. These estimates also can not differentiate between the actual noise injected as part of DP vs. the adjustments to data from the post processing steps of the DAS.
[1] Census Metrics and Esri Metrics are both calculated on the Census 2010 data as it was released to the public. These Census 2010 data are the privatized version of the Census 2010 data. For Census 2010, the DAS largely consisted of data swapping. Swapping would interchange the characteristics from one individual with another individual to maintain privacy. Although the characteristics (e.g., race) were swapped, the population counts remained unchanged.
[2] David Van Riper, Tracy Kugler, and Jonathan Schroeder. IPUMS NHGIS Privacy-Protected 2010 Census Demonstration Data, version 20210608 [Database]. Minneapolis, MN: IPUMS. 2021.
Article Discussion: