Roads and highways can be considered the backbone of any society, enabling the mobility of people and goods, and helping fuel economic prosperity. The state of Bavaria has long taken pride in its strategic location as the center of Europe and has prioritized investing resources to ensure the health of its highway systems. With evolving needs in the transportation sector, however, the challenge that the administration is facing is utilizing digitized assets efficiently and investing in strategic conservation methods to expand, preserve, and renew road network infrastructure.
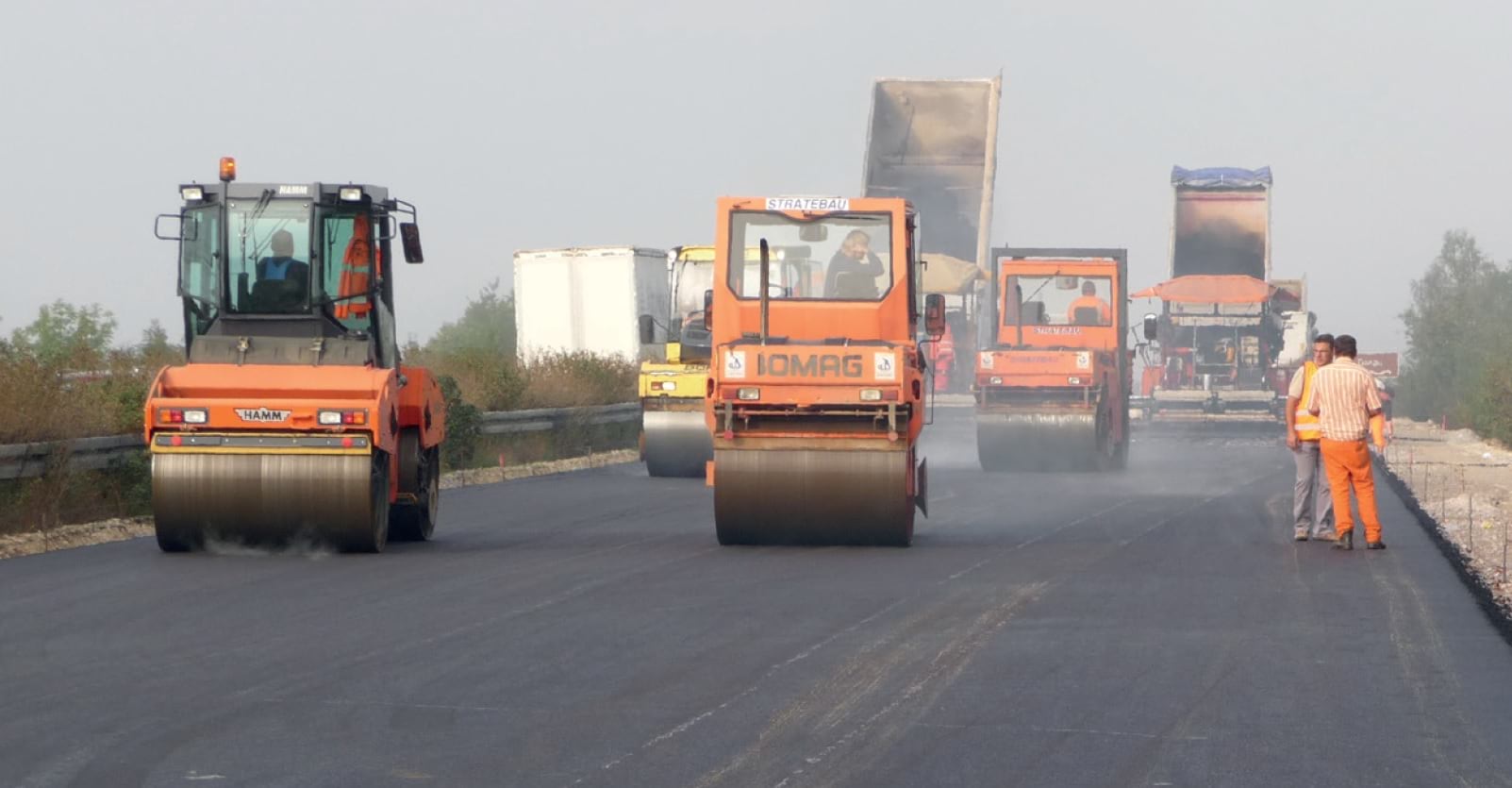
To meet this end, the Bavarian State Ministry of Housing, Building and Transport set out in 2019 to create a proof-of-concept model that predicts wear and tear on the Bundesautobahn 70 (BAB 70 or A70), a highly trafficked highway connecting multiple cities and towns in southern Germany, so that road maintenance can be efficiently planned at the proper time and location based on highway conditions. The organization partnered closely with a team of data scientists at Supper & Supper, a data science and consulting firm that advises on artificial intelligence (AI) solutions to solve problems.
The teams took on the challenge of leveraging road traffic density and road condition data, spanning several time steps between 2009 and 2017, to create a deep learning model that would predict variables representing several indicators of road conditions, such as road roughness and level of crack damage. The goal of the project was to tap into carefully curated time series data collected by the state, and leverage advancements in analytics technology to obtain meaningful predictions of how the highway would experience depreciation over time. This initiative would enable the state to plan predictive road maintenance and invest resources in areas that would most likely be impacted by the effects of use, as well as serve as a proof of concept for potential usage in other parts of the country.
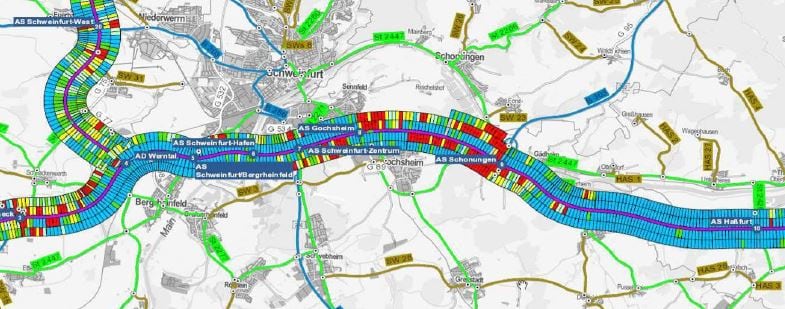
A technology stack of ArcGIS Pro and open source Python frameworks was utilized to access, wrangle, impute, analyze, and visualize data and results. The road conditions and traffic data were presented in the form of feature classes, where each feature was a 100-meter-long road section for every lane in both directions. Altogether, there were roughly 4,800 highway road sections along this portion of the A70 highway. The team used ArcGIS Pro to visualize the data and perform pre-processing functions such as identifying highway areas that overlapped, finding buffer zone areas and more. The data was then cleaned, missing data values were imputed, and the models were built using popular frameworks such as scikit-learn and TensorFlow.
Overall, the team’s efforts yielded a collection of deep learning models that predicted highway wear and tear, of which the stacked LSTM (Long Short-Term Memory) CNN (Convolutional Neural Network) model proved to be the most successful at predicting the results largely due to its focus on learning from measurements in both space and time.
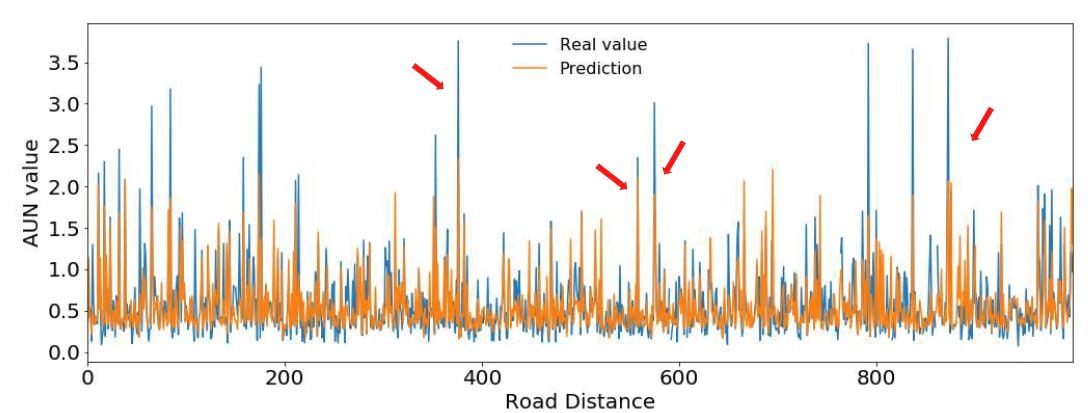
The final results were then published and visualized in ArcGIS Pro, with each road section symbolized to illustrate where road unevenness and cracks would likely develop over time. Results indicated that curved areas of the highway were the most likely to experience depreciation. These results were also visualized as an animation to show change in road conditions over time, enabling the state department to plan for predictive road maintenance actions and invest resources to ensure smooth highway operations.
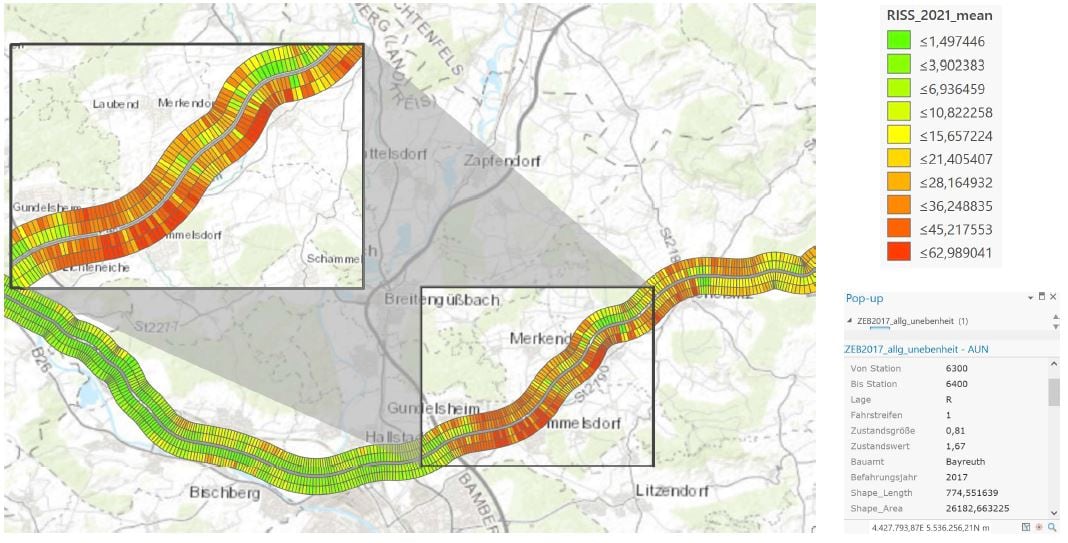
An efficient, well maintained highway infrastructure is the mark of a burgeoning economy and therefore highlights the importance of targeted proactive road maintenance. From a financial perspective, the approximately 23,000-kilometer-long network of roads and highways in Bavaria account for more than 40 billion euros in fixed assets for the state. With the goal of the state to maintain and grow this value while utilizing available budget funds, there is an inherent need to find modern solutions to alleviate the age-old problem of road maintenance. GeoAI solutions can be considered for these problems – and the Bavarian state is well on their way to breaking new ground in this endeavor.
If you are interested in exploring the spatial analysis and data science capabilities across ArcGIS, learn more here.
Article Discussion: