Customers in a B2B or B2C setting want service at the moment of demand. To answer that call, innovative companies are borrowing techniques from manufacturers and combining them with location technology, IoT, predictive analytics, AI, and blockchain to deliver car shares, coffee, productive crops, and other services just in time for demanding customers.
In the latest installment of the WhereNext Think Tank series, Esri’s Brian Cross talks to Kevin Bolger, practice lead for AI and machine learning, about delivering smart service on time.
New Building Blocks for Smart Service
Brian Cross: Kevin, we’ve talked in the past about how IoT and location intelligence are changing the way manufacturers and retailers think about things like supply chain. But an area that really hasn’t gotten the attention it’s due is service delivery.
We know we’re moving to a service economy, but I don’t think we have a clear understanding of how location intelligence and IoT change the way service providers work. What are you seeing among market leaders?
Kevin Bolger: These companies now have information they didn’t have 5 or 10 years ago. They have data from IoT devices—cell phones, sensors, and other devices in the field—which gives them the opportunity to provide smart services they couldn’t offer before.
This includes delivering goods to someone who’s not at their home or a physical address. It means having products ready for a customer at the moment of demand because the company can analyze the information coming off the customer’s device and predict when they’ll arrive at the store. It means a warehouse worker knows exactly where to get a product off a shelf with the help of indoor location intelligence and AR/VR technology that shows them a picture of which part to pick.
Companies can provide all these smart services because they have data they didn’t have before, and because all that data is associated with a specific location.
Service Innovation in B2B and B2C
Cross: I see a couple of themes there. One is the coordinated handshake between a consumer buying something and the business delivering it. Another is employee productivity—being more efficient in the service activities they perform in the warehouse, for example. Are you seeing these innovations more in the B2C space or in B2B?
Bolger: The innovation we see in smart service is following a familiar path: B2C companies are pushing the cutting edge and doing the first implementations of the technology.
But it’s interesting that those B2C companies—as they use new data and technology to improve service—are actually applying techniques from the world of just-in-time manufacturing. In fact, some forms of smart service could be called just-in-time service—they involve delivering an item or service at the moment the customer needs it. That’s what manufacturers have been doing for years in their factories—delivering components and assemblies to the production line just as the right chassis comes along.
In both cases, the company needs data and predictive analytics to assess what will happen so they can organize their service business to deliver effectively.
Delivery at the Moment of Demand
Cross: In the consumer space, we’ve heard a lot about companies using innovative techniques to deliver packages to my house faster, for example. How else are B2C companies improving service with the help of location analytics?
Bolger: We’ve done some interesting exploratory work with a large coffee retailer. The concept was for a new mobile service where I could order my coffee before I leave the house and the shop would track me through its app and predict exactly when I would arrive at the location. They’d have the coffee ready for me when I walk in the door.
So I would no longer have to stand in line, and I wouldn’t have to worry about the coffee getting cold. On the other side, the retailer could better plan its staff and operations. And that’s all enabled by a geographic information system [GIS], which sees where the customer is, understands traffic patterns and expected construction delays, and predicts when they’ll arrive.
Another interesting example involves one of the world’s largest pizza companies. They’re using a mobile app that lets customers order a pizza at a location that has no address. If I’m at a park at a party with my family and I want to order pizza, I can do that through the app and have it delivered to the parking lot.
That kind of service innovation is only possible when you have an IoT device providing real-time location information and a mapping technology like GIS that plans the optimal service delivery.
[For more on how companies are using IoT data to their advantage, explore this e-book.]
The Cutting Edge of Service—Down on the Farm
Cross: Can you give us an example of how a B2B company is creating smart services, and the kind of value they’re delivering through tools like location intelligence and IoT?
Bolger: We’ve worked with some large equipment manufacturers in the agricultural business. They’re collecting data from IoT sensors on their equipment, then analyzing that with additional data like soil types, and creating smart maps that show farmers how to manage fields. Their location intelligence is so high, they’re able to tell farmers to plant a particular seed in a particular inch of ground. In some cases, the manufacturer delivers the plan digitally to [farmers’] equipment, and the equipment navigates on its own and executes the planting plan.
Those companies are now delivering a smart service, rather than just farming equipment. They can make that a value-added service if they choose, or they can create whole new lines of business around the services.
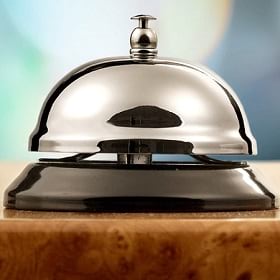
That's all enabled by a geographic information system [GIS], which sees where the customer is, understands traffic patterns and expected construction delays, and predicts when they’ll arrive.
Field Service Providers Get Real (Time) and Predictive
Cross: What about the service experts working in the field, whether that’s a team of high-tech workers, inspectors, or utility and oil field workers? How have location intelligence and new data sources changed the way they deliver service?
Bolger: We’re seeing organizations with fieldworkers doing a much better job of orchestrating their activities with location intelligence and GIS. They’re using smart maps to see where each technician is in real time and optimize service calls on the fly, rather than sticking to predetermined schedules. That creates efficiency and improves responsiveness to customer expectations.
Cross: Those kinds of organizations aren’t content to be reactive anymore. They seem to be more focused than ever on delivering preventive services that keep clients’ operations running without interruption.
Bolger: That’s true, and it’s a reaction to higher expectations among B2B and B2C customers. For example, we work with one of the world’s largest high-tech equipment manufacturers—hardware that is highly instrumented and monitored through IoT by the manufacturer. The company is doing analytics to understand the behavior of each piece of equipment and the conditions that may cause it to fail. They routinely sell service plans that include preventive maintenance.
But when something does fail, the service team sees the issue on a smart map and can dispatch the right service technician to the location quickly—at the moment of demand. And they can do that both outdoors and indoors, thanks to the emerging capabilities of Indoor mapping and positioning.
The company also uses GIS and IoT data for planning. Service managers see on a map exactly what equipment is installed in a given service territory, which helps them assign technicians effectively. They also run analyses on the failure rates of that equipment so they can stock the appropriate parts and keep their inventory carrying costs lower than competitors’.
Mapping the Uncharted Service World
Cross: It’s interesting how many companies we work with struggle to route fieldworkers effectively because they’re going to locations that don’t exist on public maps.
Bolger: Right, and traditionally they’ve relied on hand-drawn maps or the driver’s memory, which can be error prone and inefficient. Now they’re using new techniques like image detection and machine learning to do automated road mapping.
Think about an oil and gas company or an electric utility. [These companies] own huge swaths of land that often aren’t recorded on public roads. We’re working with those companies to do two things. One is capturing what we call breadcrumbs—data from IoT devices like a smartphone that reports where the field worker is every 5 or 10 seconds. Two is combining that information with aerial imagery of the dirt roads and automatically creating road segments on their maps with the help of machine learning and artificial intelligence.
This is a huge cost savings for these businesses because it helps them see the territory and create optimized routes for their technicians to service jobs quicker.
Speeding up the Service Delivery Chain
Cross: That brings up the concept of the service delivery chain. When products in the field fail, warehouse workers need to quickly find and deliver replacement parts. How are they doing that just in time?
Bolger: This is where indoor location technology, augmented reality, and blockchain are coming together in an interesting way.
Especially in the B2B world, companies are often obligated by an SLA to repair or replace products in short timeframes. Because of that, many of them are focused on making the warehouse more efficient.
Here’s one scenario we’ve seen: indoor location technology routes a warehouse worker to a certain section or shelf. The worker might be wearing special glasses or looking at a mobile device that shows the route. When the worker is in the right general area, an augmented reality tool on the glasses or the device reveals exactly where the replacement part is, and how many are needed for the job.
A blockchain record shows the chain of custody for those products—how their ownership has changed from manufacturer to shipper to trucking company to the OEM.
All those data points come together in one solution that allows the person in the warehouse to quickly retrieve the right parts and meet service delivery expectations.
Cars on Demand
Cross: We’re also seeing service innovation in the realm of car subscriptions. I’ve heard there’s an interesting intersection of IoT, just-in-time service, and even blockchain in that field. Can you give us a sense of what’s emerging?
Bolger: Rental car companies and car manufacturers have embraced the idea of letting people rent cars where and when they need them—again, at the point of demand. You’ve probably heard that people can use a mobile app to find a nearby car to rent, but there’s a lot that goes on behind the scenes to make that happen.
When the manufacturer or rental company wants to figure out where to place these cars, they’re using GIS-based location analytics to examine the demographics of certain neighborhoods so they can stage the right vehicles near the highest demand. They combine that analysis with IoT information on actual driving behaviors and patterns, and that insight helps them create a network of vehicles to answer customer needs across an entire city.
Behind the scenes, technology like blockchain plays a role too. If I walk up to a rental vehicle parked on the street, there are rules behind whether I’m allowed to take that car or not. Blockchain—or geoblockchain, as it’s coming to be known—manages the rules of ownership for specific cars in specific locations.
Innovation from the Sky
Cross: If we come back to the classic customer service example of delivering a meal, what other kinds of innovation are you seeing?
Bolger: We’re working with a leading national fast-casual restaurant that’s incorporating aerial imagery, machine learning, and location intelligence to understand how well they’re servicing customers at their restaurants.
This particular restaurant tends to have long lines at its drive-throughs, and executives wanted to understand how that contributes to drive-offs—people who leave when they see the size of the line, or who get in line but drive off because they get tired of waiting. The team applied machine learning to aerial imagery to classify the drive-offs, and then organized the information in GIS. That helps them understand how to improve services and retain every prospective customer.
We see that same motivation among many companies we work with—they want to meet their customers at the moment of demand. They’re using new data and tools to drive out inefficiencies and create business opportunities, one location and one service at a time.