This year’s annual Esri User Conference, held from July 10-14 in San Diego, marked a significant milestone as our largest UC event to date with approximately 18,000 in-person attendees and an additional 15,000 digital attendees. Whether attending UC in person or digitally, there were abundant opportunities for learning about spatial analytics through informative sessions, engaging demos, technical workshops, and the Spatial Analytics Summit. Keep reading for a UC recap of the key highlights and trends in spatial analytics that were discussed at UC!
Spatial Data Science is Everywhere
It’s increasingly clear in the last few years that spatial data science has gained momentum across many industries. More and more data is being collected in conjunction with geographic context – Internet of Things sensor data, mobile business apps that enable field workers to automatically record accurate location data, drones which capture high resolution imagery and video quickly and cheaply, and consumer apps that can track user movements if they opt in.
This increase in geospatial data is happening across all industries, including organizations that may not have longstanding experience in GIS. Whether in government, natural resources, healthcare, commercial business or otherwise – organizations are looking to leverage their new and valuable data for better intelligence that unlocks new opportunities, optimizes operations, mitigates risks, and drives innovation. Spatial data science enables us to analyze geographic patterns, relationships, and trends in ways that were previously unimaginable.
This expansion of interest in spatial data science was overwhelmingly evident at this year’s User Conference. From the first-ever Spatial Analytics Summit to the more than 50 workshops, special interest groups, and user presentations on spatial analytics, to the long lines at booths on the showcase floor, spatial analytics and data science were hot topics for both users and partners. Attendees wanted to understand what kinds of problems can be solved with spatial data science, learn about the techniques, and get a sense of what tools are available.
Top Three Trends
While many trends were discussed at the Summit and across the conference, the top trends we saw making an impact in spatial analytics and data science were cloud big data architectures, GeoAI, and graph analytics.
Gain an overview of Spatial Analysis & Data Science via this Plenary video (timestamp 1:22:55 – 1:27:28).
1. Cloud Big Data Architectures
Big data, often accumulated by automated data collection over long periods of time, continues to be a challenge for many organizations. Increasingly, customers are amassing this data in cloud data lakes and warehouses, but these large volumes of data mean nothing unless it can be used effectively. And to do so requires exploiting data in these cloud-native architectures at scale, without duplicating or moving the data to use in different software applications.
Big data is often processed and analyzed utilizing Apache SparkTM, an engine for distributing and processing data across parallelized jobs or computing nodes. Spark is used for data engineering and data science in many of the most widely used cloud platforms, and now with ArcGIS GeoAnalytics Engine, users can leverage a comprehensive library of spatial analytics from ArcGIS, directly within those environments.
GeoAnalytics Engine was featured at UC at the Spatial Analytics Summit, as well as several sessions and events. Check out the Big Data and GeoAnalytics UC technical session to learn more.
2. GeoAI
Artificial intelligence (AI) is one of the biggest topics in technology today. Use of AI is exploding in almost every industry for its potential to reduce manual, time-consuming workflows, or to quickly generate candidate solutions to problems that can then be further refined by subject matter experts. GeoAI is the application of artificial intelligence (AI) fused with geospatial data, science, and technology. It can be used to improve data quality, reduce the time needed to gain situational awareness, and improve decision-making by applying insight from spatial patterns and predictions.
There two main forms of GeoAI. The first is accelerated geospatial data generation from sources such as imagery, video, point clouds and text. This typically involves deep learning processes and algorithms to extract, classify, and detect individual spatial features. GIS users can easily get started with these capabilities through resources such as pretrained deep learning models that support building footprint & road extraction, land cover classification, object tracking, and more. The second main aspect of GeoAI is predictive modeling via spatial machine learning. Users can leverage these capabilities to detect clusters in spatial data, find patterns, and forecast outcomes. A primer on regression and classification is available to help familiarize users with these concepts.
At the Spatial Analytics Summit, case studies from GeoAI projects were shared by Pablo Rebufello of ICA, Uruguay, including use cases around predicting animal disease and detecting treetop data to inform forestry workflows. Catch the recording of the technical session GeoAI in ArcGIS: An Overview for more information.
3. Graph Analytics
Another new and exciting trend in spatial data science is in the area of graph analytics. Knowledge graphs integrate data from different sources and enable tracing networks of relationships between entities in the graph. This data fusion, combined with creating connections between data elements, can reveal patterns and expose indirect relationships that are otherwise difficult to see. Users can ask and answer questions easily, whether they are working supply chain optimization, financial fraud investigations, or identifying sources of risk.
As graph analysis relies on the generation and mining of relationships between entities, integrating graph workflows with spatial analysis opens up exciting possibilities. Graph entities can be visualized in their geographic context, exposing the existence of important spatial relationships that were not present in the explicit graph connections. This can provide a critical missing link in an analysis that might otherwise have been missed. ArcGIS Knowledge unlocks these kinds of capabilities within ArcGIS Pro, enabling users to analyze spatial and nonspatial, unstructured, and structured data together to speed up decision-making.
If you didn’t get a chance to connect with the Knowledge team at the Spatial Analytics showcase or attend the demo theaters and workshops, you can still get an overview by watching ArcGIS Knowledge: An Introduction or see more of what’s possible in the Advanced Analytics with Knowledge Graphs technical sessions.
Additional Resources
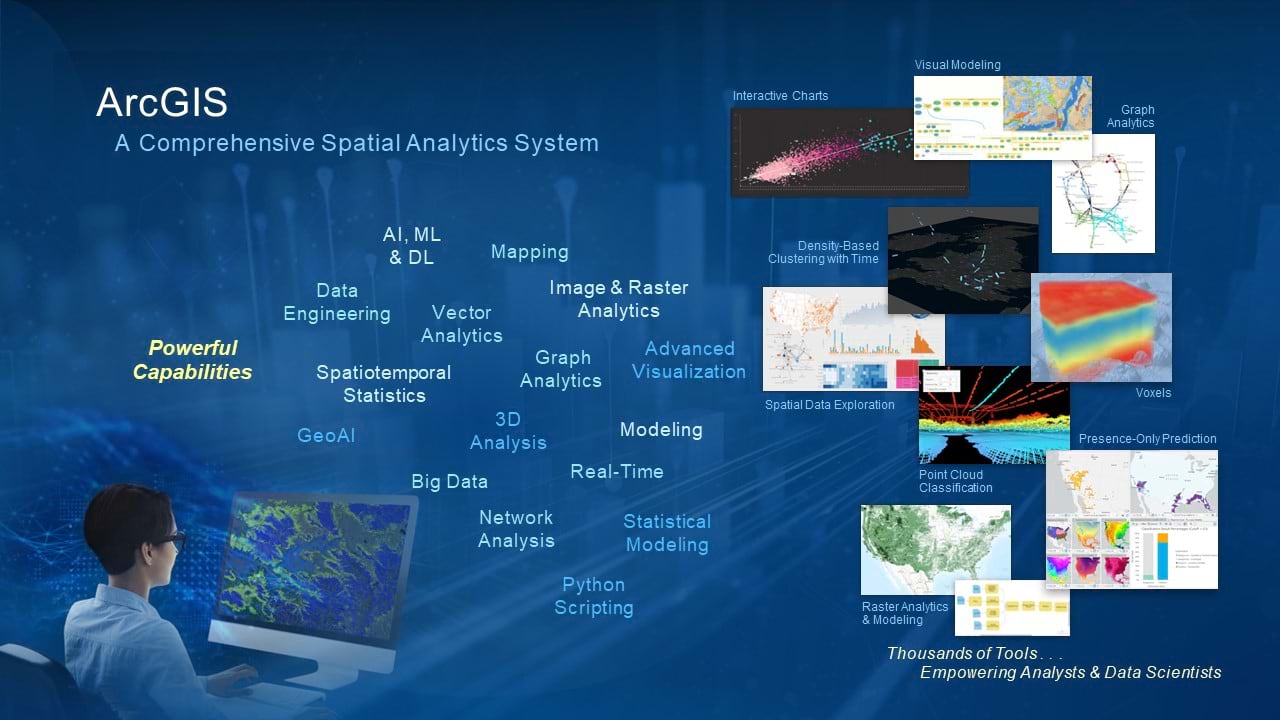
We hope you were able to take part in this year’s User Conference and benefit from the amazing community and resources for spatial data science. If you’re interested in learning more about capabilities in ArcGIS for analyzing and identifying geographic patterns, relationships, and trends, don’t hesitate to check out the Spatial Analytics and Data Science site, or the Spatial Statistics Resources hub.
Article Discussion: