Are you a wildlife biologist seeking a spatial statistics tool to better predict the impact that climate change will have on the habitat of an endangered species? Or are you a spatial data scientist needing a space-time pattern analysis tool to facilitate your study of changes in statistical properties of a space-time cube? If yes, you are in luck because two of the newest tools in ArcGIS Pro 2.9, Presence-only Prediction and Change Point Detection, can empower you to do just that and so much more.
At the Developer Summit 2022 plenary, Alberto Nieto offered a glimpse of what can be accomplished with these new tools.
Watch the plenary video below, and then read the rest of the blog for a summary of the processes that Alberto explored in his demo.
Presence-only Prediction
The Presence-only Prediction tool utilizes known occurrence points and explanatory variables to estimate the probability of presence of a phenomenon across a study area. The tool adopts a maximum entropy (MaxEnt) approach to make predictions.
For his demo, Alberto used the Presence-only Prediction tool to predict the presence of hospitable habitats for gopher tortoises across the US and assess the effects of various climatic factors on their presence.
First, he showed a map of known gopher tortoise locations in a subset of the southwest US and explanatory rasters that describe the climate-related factors—namely, temperature, precipitation, and climate zones—which relate to the presence of the tortoises.
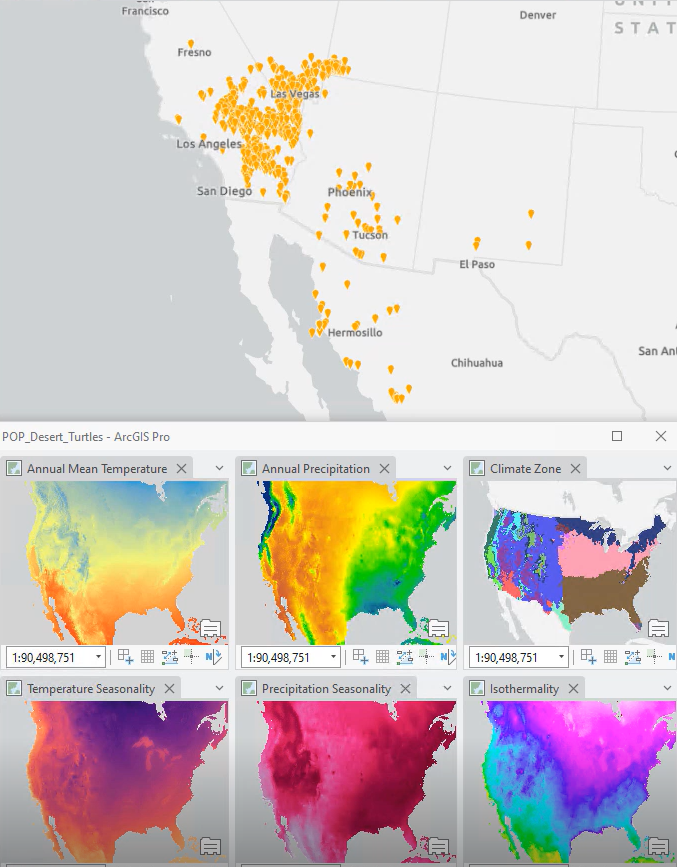
Next, he used the Presence-only Prediction tool with the known locations of the tortoises and the explanatory variables to create a presence prediction model. Since the tool uses a MaxEnt approach, defined absence locations were not required to generate the model. As part of the geoprocessing results, the tool provided a Classification Result Percentages chart to evaluate prediction performance across the training features. In the chart, the blue and orange bars represent the correctly and incorrectly classified presence points, respectively. The model correctly classified approximately 80% of the known location points.
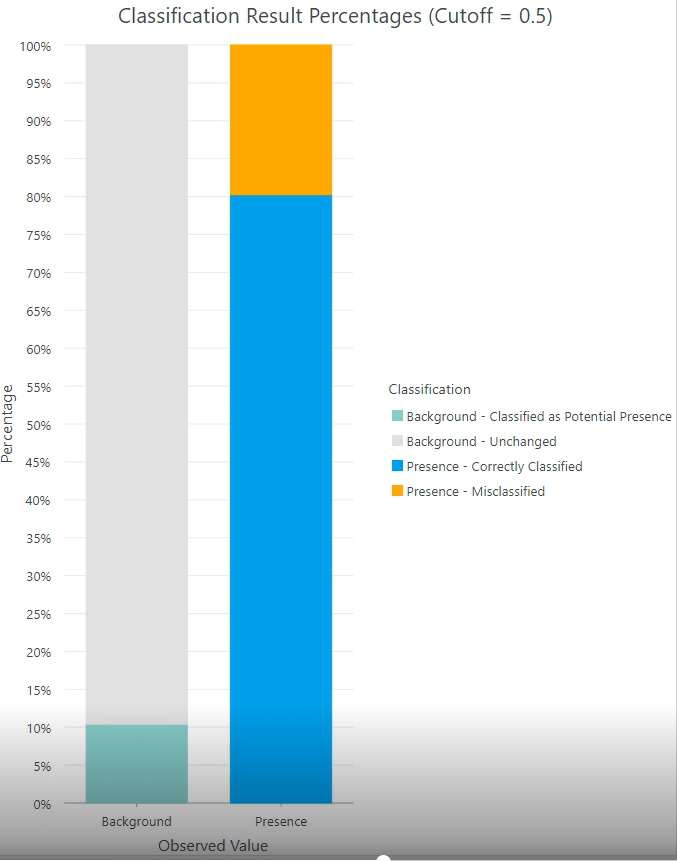
In the map of predicted locations, Alberto observed that favorable habitats for gopher tortoises are also probable in regions in Texas, Florida, and other states in the southeast US.
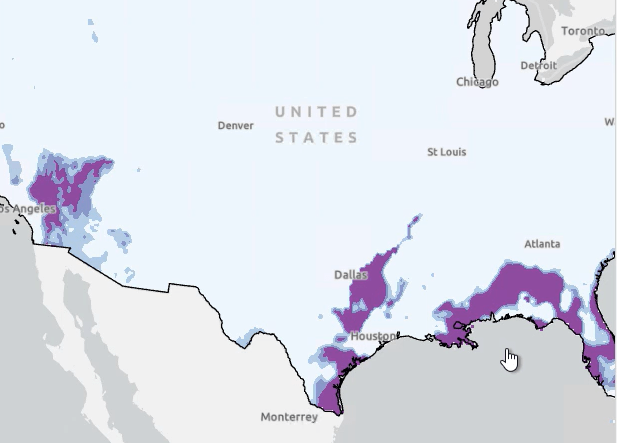
He then showed the Partial Response of Continuous Variables chart, also provided as part of the geoprocessing results. The chart comprises multiple charts; each chart visualizes the relationship between each explanatory variable on the presence probability of gopher tortoise habitats
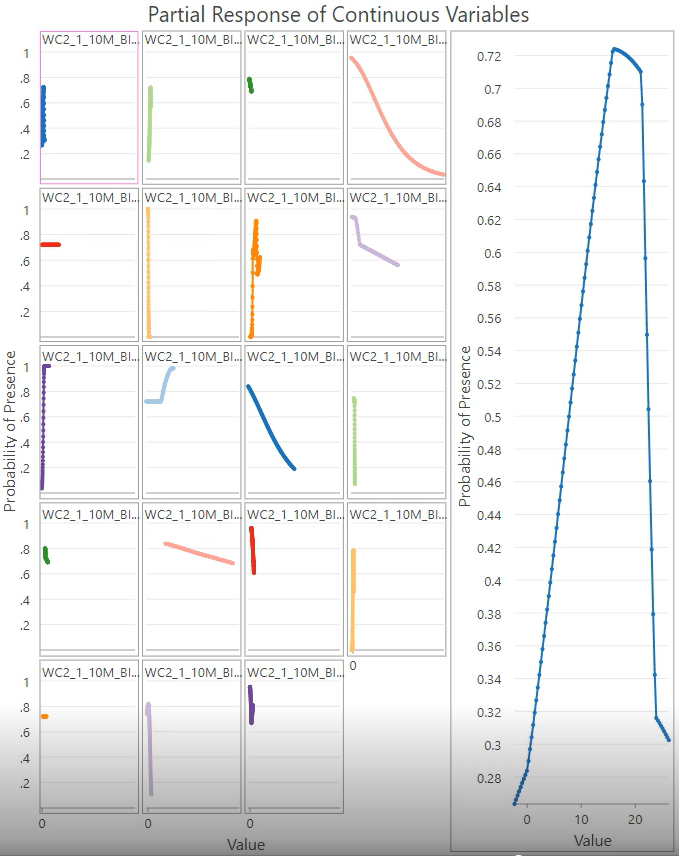
Finally, to understand the impact that climate change will have on the habitat of the gopher tortoise, Alberto used the US climate change projections to model estimated gopher tortoise distribution as the impacts of climate change are observed in the explanatory variables. The resulting estimates show the warming parts of the country may become suitable habitats for the tortoises while the existing habitats may become less favorable.
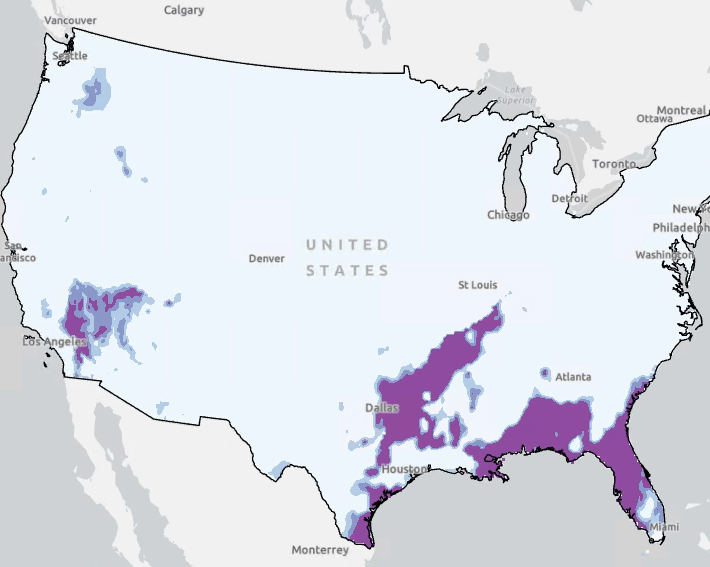
Change Point Detection
The Change Point Detection tool identifies time steps when a statistical property of the time series changes for each location of a space-time cube. The tool can detect changes in four statistical properties: mean, standard deviation, slope, and count.
For the demo, Alberto used the Change Point Detection tool to identify years when trends in small business loan applications changed across all US counties, placing particular emphasis on the influence of the COVID-19 pandemic in 2020.
Straight away, Alberto ran the Change Point Detection tool to detect changes in slope using a space-time cube containing the annual count of new small business loan applications across US counties from 2005 through 2020. The tool detected either 0, 1, or 2 change points across all counties.
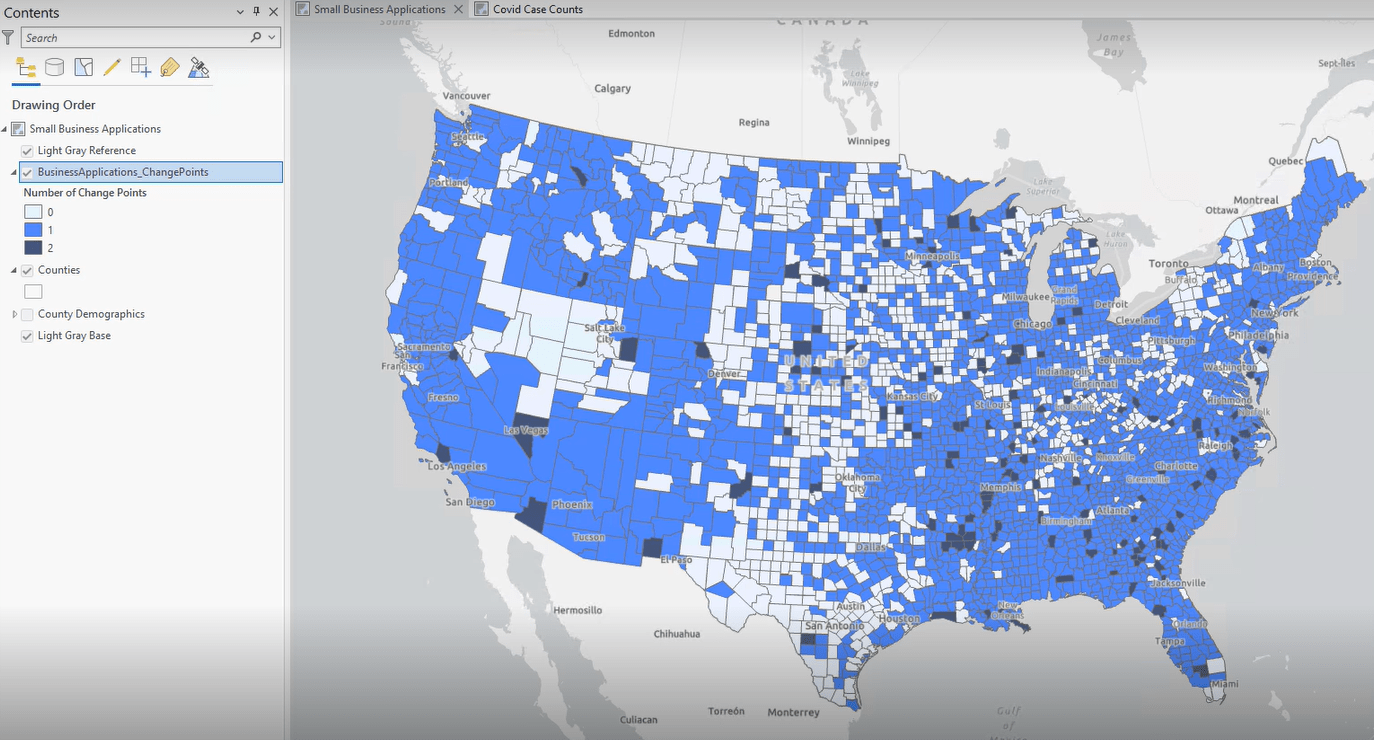
Next, he used the automatically configured time slider to show only those counties that experienced their first change point in 2019; these counties, mainly clustered in the southeast US, observed steady trends in small business loan applications between 2005 and 2019 but had a shift in trends between 2019 and 2020.
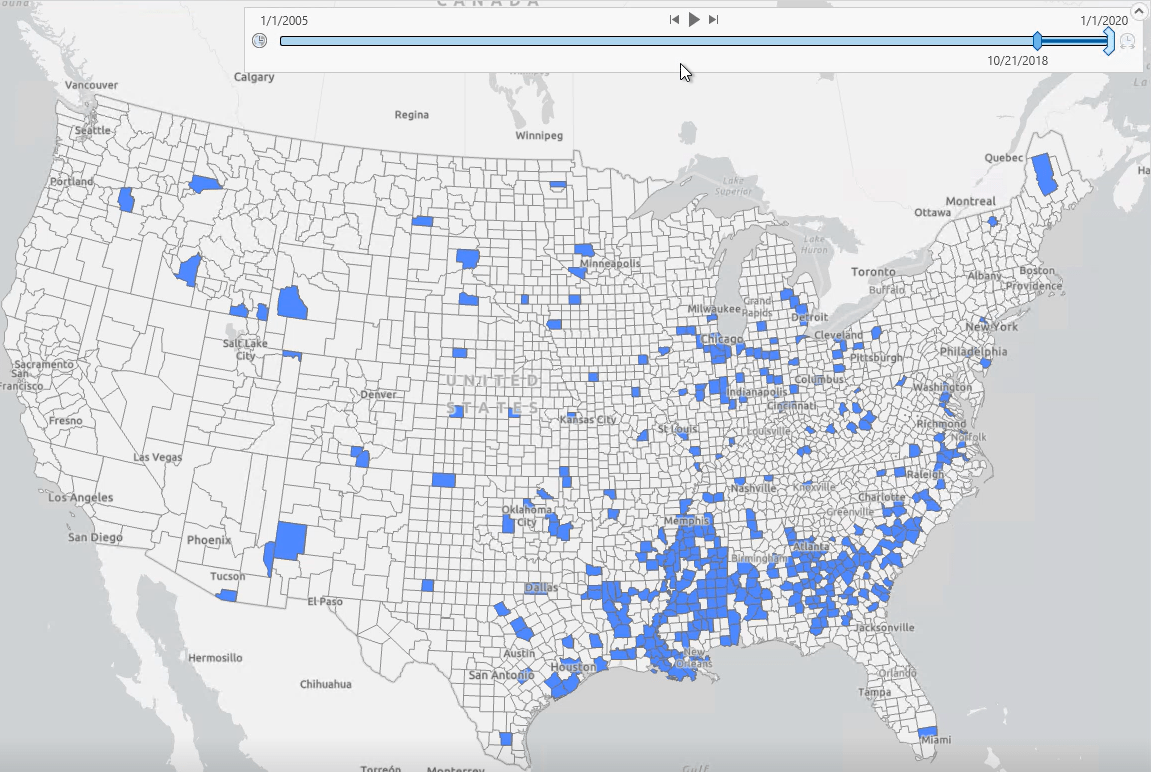
Alberto observed that some counties experienced a significant increase in small business loan applications, with some showcasing as much as a six-fold increase.
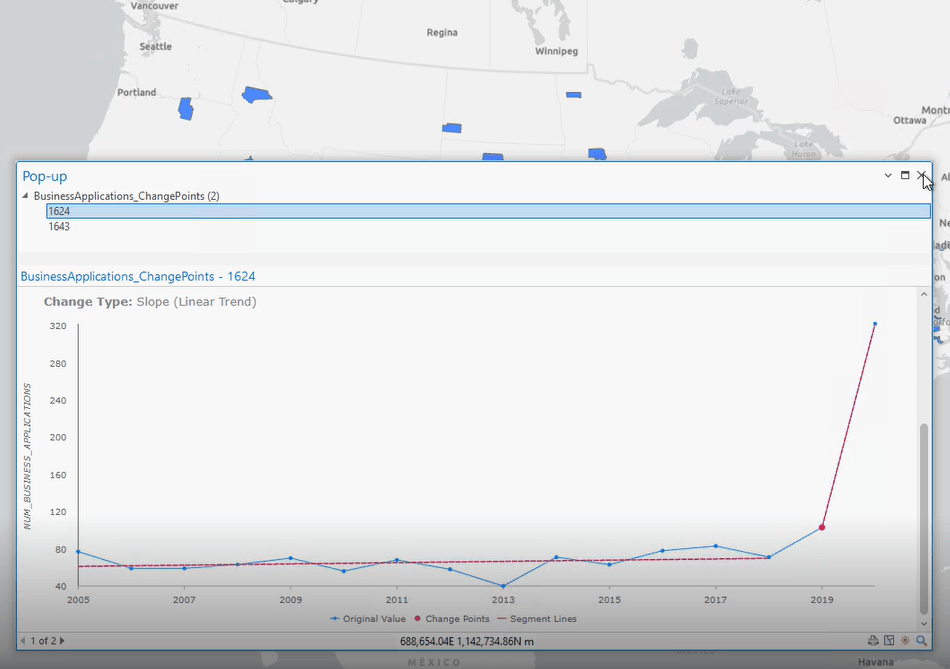
When viewed in conjunction with county demographics with the help of the Swipe tool, there was a discernible association between the percent of the population that identifies as Black or African American (not Hispanic or Latino) and the rise in the number of small business loan applications.
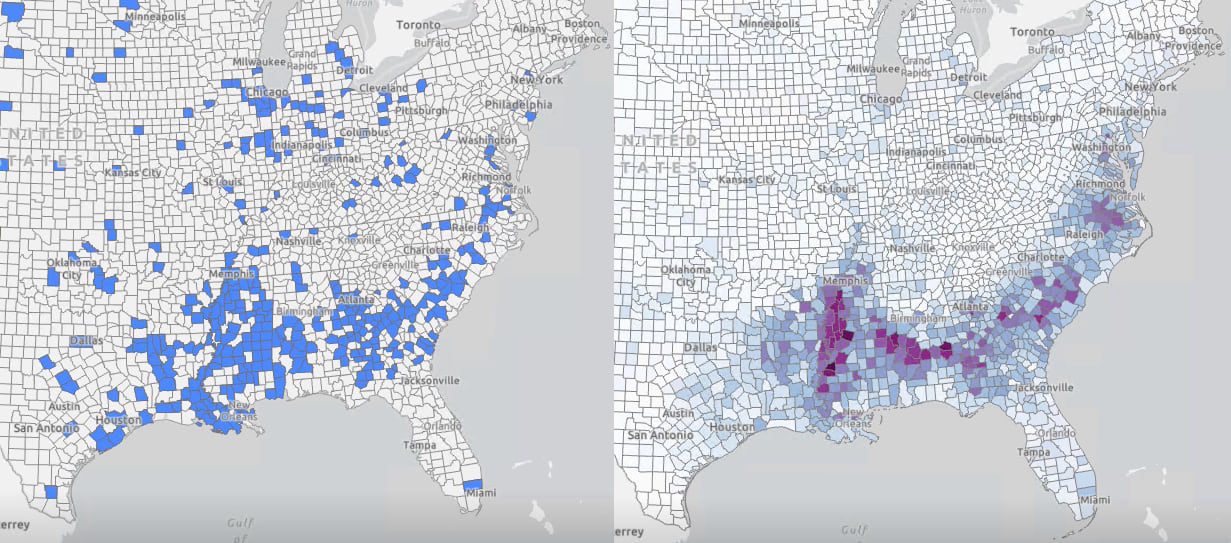
Learn more
Alberto’s demo showed how you can use the Presence-only Prediction tool and the Change Point Detection tool to predict the presence of a phenomenon and detect change points in time, respectively. Visit the ArcGIS Pro documentation to learn more about how you can use these tools to facilitate your GIS work. Additionally, visit Jie Liu’s blog—Presence-Only Prediction (MaxEnt) 101: Using GIS to model species distribution—for a detailed dive into the Presence-Only Prediction tool and step-by-step instructions on how to work the tool using the same dataset that Alberto explored in his demo.
Commenting is not enabled for this article.