Introduction
In the realm of computer vision and image processing, segmentation models are indispensable tools, enabling pixel-wise classification to identify and delineate objects or regions in an image. However, conventional segmentation approaches often require laborious training on labeled datasets specific to the objects of interest, posing significant challenges in scalability and adaptability.
A groundbreaking innovation—Segment Anything Model (SAM) by Meta—promises to reshape the landscape of image segmentation. SAM uses zero-shot learning to generalize across domains without the need for extensive retraining, providing unprecedented flexibility and efficiency in identifying diverse objects in imagery.
At the heart of SAM lies its remarkable capability to segment virtually anything in images, driven by a comprehensive dataset known as Segment Anything 1-Billion mask dataset (SA-1B). With more than 11 million images and 1 billion masks, SAM boasts robustness in delineating object boundaries and discerning between various entities, transcending domain-specific limitations.
SAM is now integrated with ArcGIS and released as one of the pretrained models on ArcGIS Living Atlas of the World. This model performs pixel-wise classification by classifying the pixels into different classes. The classified pixels correspond to different objects or regions in the image.
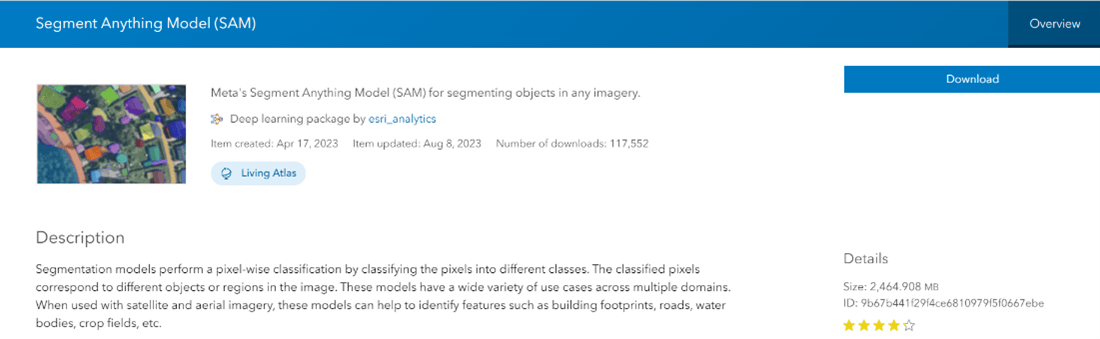
SAM empowers users across diverse domains, including urban planning, agriculture, environmental monitoring, and disaster response. By harnessing SAM, users can extract masks of various objects such as buildings, roads, vegetation, water bodies, and more from satellite and aerial imagery with unparalleled accuracy and efficiency.
The following video demonstrates the application of SAM in ArcGIS Pro for the detection of mussel farms, facilitating their management and monitoring.
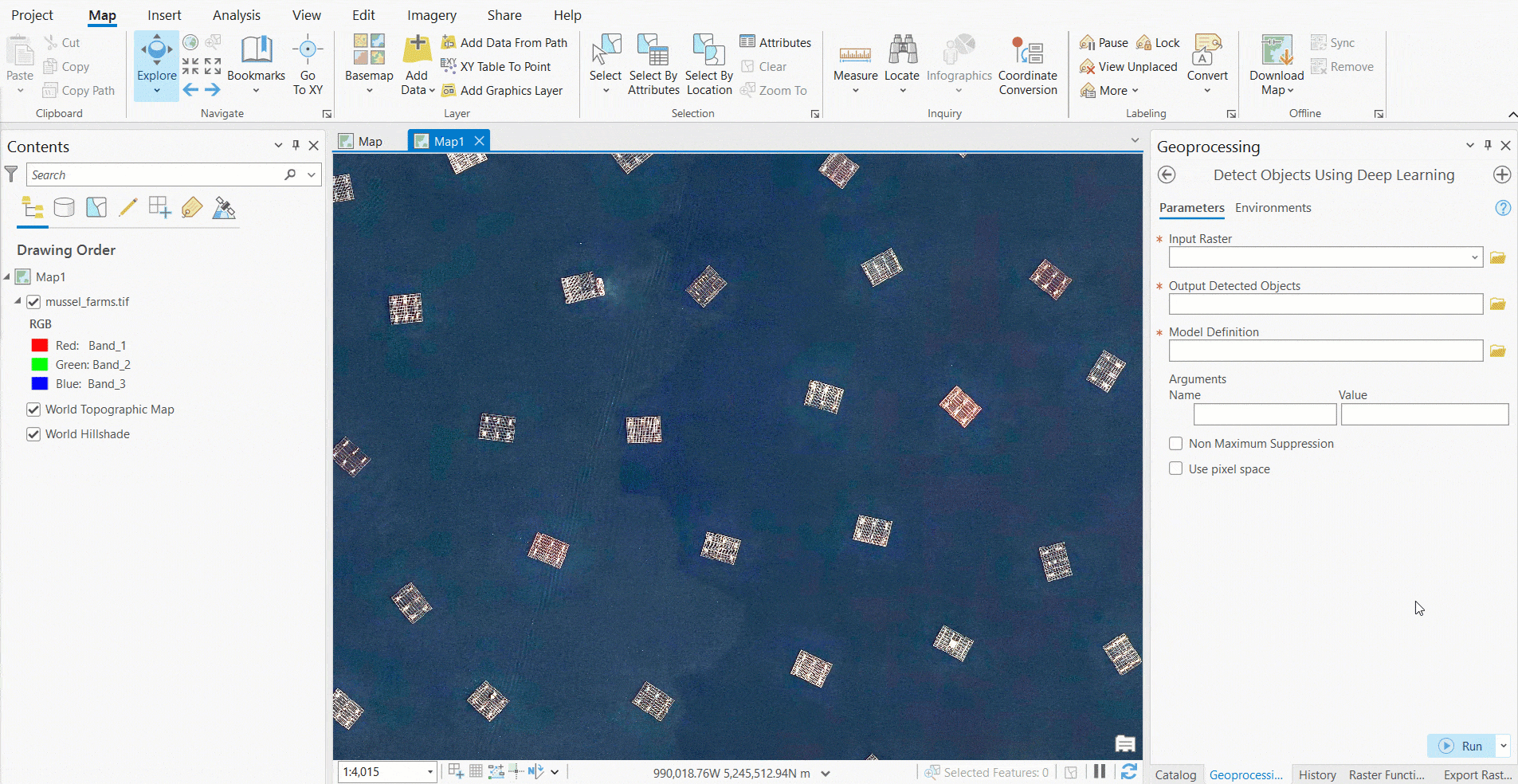
Tricks for optimal SAM usage
While SAM excels in segmenting diverse objects, users can enhance its performance by considering a few crucial factors:
- Parameter adjustment— SAM’s performance depends on parameters such as cell size and batch size. Adjusting these parameters according to the target object in an image is essential for optimal results.
- Post-processing: Additional post-processing using geoprocessing tools available in ArcGIS Pro may be necessary to refine SAM’s predictions and eliminate noise, such as false positives. Techniques such as applying definition queries based on confidence levels or shape attributes can effectively filter out unwanted artifacts.
Using these tricks, we have obtained clean results for various objects of interest shown in the story below:
Limitation
In a complex scene in which multiple features are present in the imagery such as buildings, roads and trees, SAM segments anything and everything that gets in the way of feature extraction.
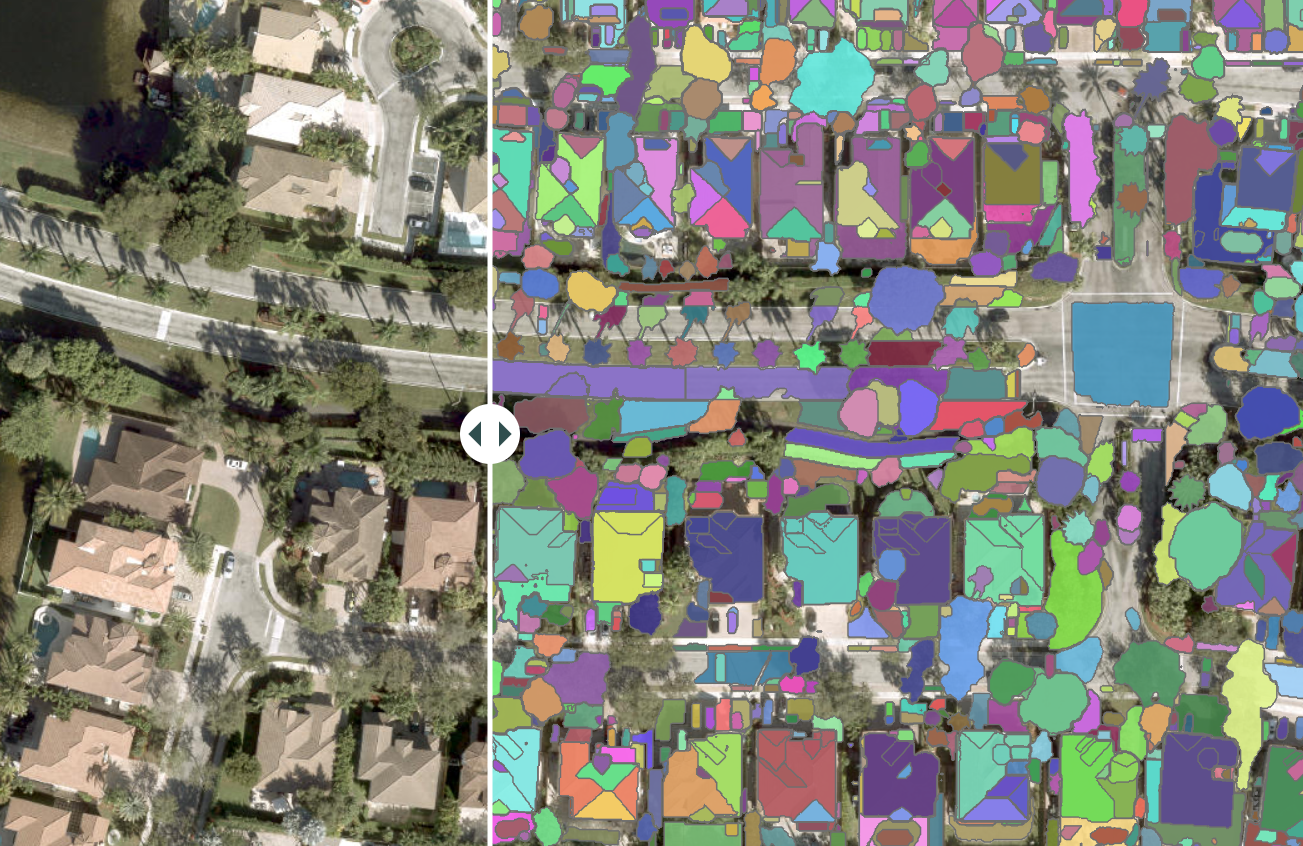
To address this limitation and prompt SAM to extract specific features from imagery, we have introduced Text SAM, a complementary model that allows users to extract features of interest using text prompts. To learn more about Text SAM, refer to our next blog post.
Conclusion
In summary, SAM revolutionizes image segmentation by diverging from traditional methods. Through zero-shot learning and extensive training data, SAM provides unmatched flexibility, accuracy, and efficiency in extracting insights from images. As we enter the age of data-driven decisions, SAM represents a pioneering tool, creating new possibilities in spatial analysis and beyond.
Resources
https://doc.arcgis.com/en/pretrained-models/latest/imagery/using-segment-anything-model-sam-.htm
Article Discussion: